Transient Classifiers for Fink: Benchmarks for LSST
arxiv(2024)
摘要
The upcoming Legacy Survey of Space and Time (LSST) at the Vera Rubin
Observatory is expected to detect a few million transients per night, which
will generate a live alert stream during the entire 10 years of the survey.
This will be distributed via community brokers whose task is to select subsets
of the stream and direct them to scientific communities. Given the volume and
complexity of data, machine learning (ML) algorithms will be paramount for this
task. We present the infrastructure tests and classification methods developed
within the Fink broker in preparation for LSST. This work aims to provide
detailed information regarding the underlying assumptions, and methods, behind
each classifier, enabling users to make informed follow-up decisions from Fink photometric classifications. Using simulated data from the Extended LSST
Astronomical Time-series Classification Challenge (ELAsTiCC), we showcase the
performance of binary and multi-class ML classifiers available in Fink.
These include tree-based classifiers coupled with tailored feature extraction
strategies, as well as deep learning algorithms. We introduce the CBPF Alert
Transient Search (CATS), a deep learning architecture specifically designed for
this task. Results show that Fink classifiers are able to handle the
extra complexity which is expected from LSST data. CATS achieved 97%
accuracy on a multi-class classification while our best performing binary
classifier achieve 99% when classifying the Periodic class. ELAsTiCC was an
important milestone in preparing Fink infrastructure to deal with
LSST-like data. Our results demonstrate that Fink classifiers are well
prepared for the arrival of the new stream; this experience also highlights
that transitioning from current infrastructures to Rubin will require
significant adaptation of currently available tools.
更多查看译文
AI 理解论文
溯源树
样例
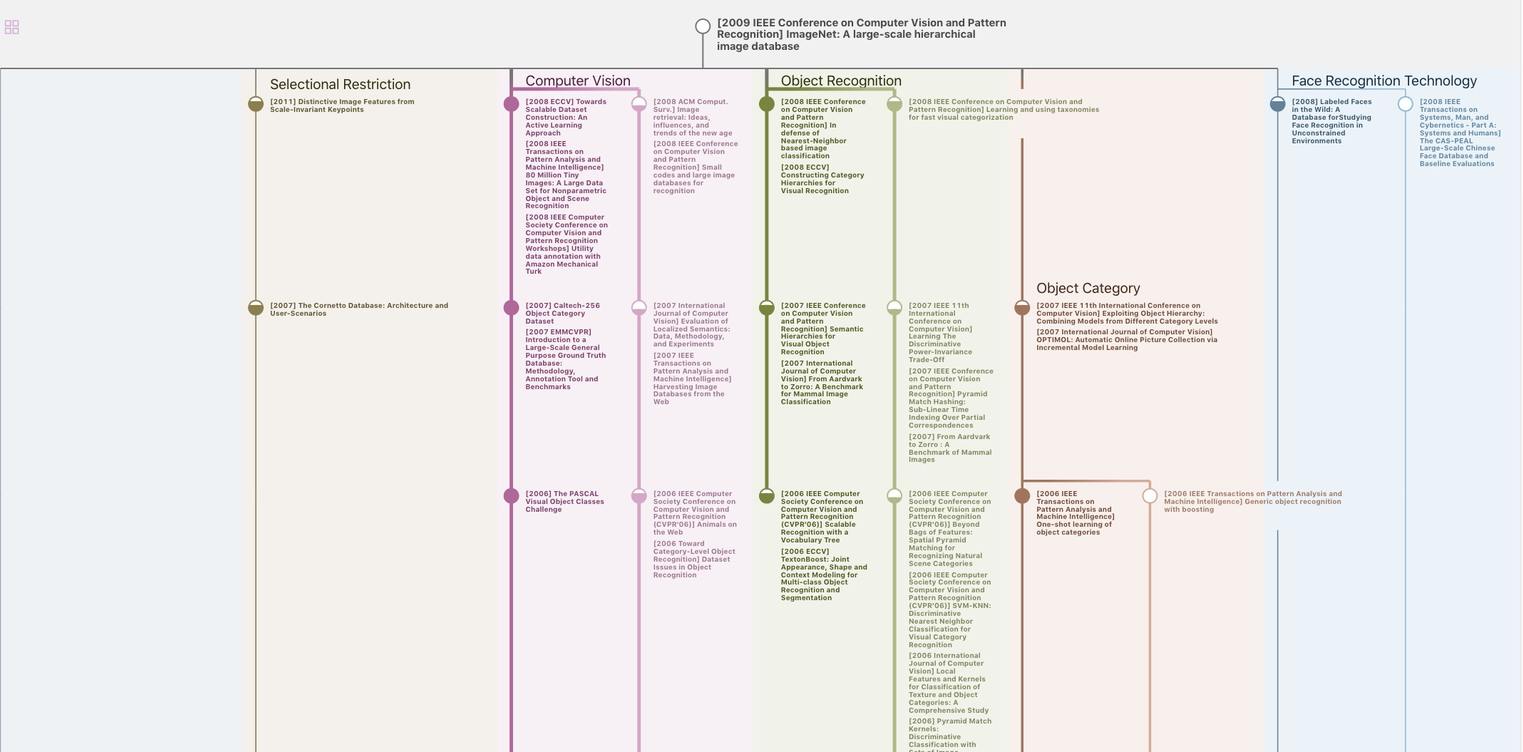
生成溯源树,研究论文发展脉络
Chat Paper
正在生成论文摘要