Capturing biological interactions improves predictive ability of complex traits via epistatic model
crossref(2024)
摘要
Abstract Recent studies have emphasized the significance of epistatic effects in unraveling the underlying genetic architectures of complex traits. However, the rich landscape of biological interactions is often disregarded when statistically modeling epistatic effects for the purpose of dissecting and predicting complex traits/diseases. In this study, we utilize KEGG pathways as carriers of biological interactions, thereby bridging the gap between statistical and biological interactions and enable to capture the epistatic effects of complex traits. We introduce biBLUP (biological interaction best linear unbiased prediction) model for modelling the epistatic effects among genes from the KEGG pathways. Simulation experiments demonstrate that biBLUP effectively captures two- and three-dimensional interaction effects underlying complex traits across diverse scenarios, resulting in a maximum increase of 62% in the predictive accuracy of the simulated complex traits. In a specific application on data consisted of 6,642 yeast lines, biBLUP successfully links complex phenotypes to biological pathways. By modeling the genetic interaction effects within the KEGG pathway associated with allantoin utilization, biBLUP achieves 40.36% improvement in accuracy for predicting the growth rate of yeast. Additionally, our study demonstrates that the incorporation of KEGG into biBLUP effectively captures the validated epistatic effects related to the flowering time of rice. This integration results in an improvement of 16.29% in the accuracy for predicting the flowering time of rice using biBLUP. In summary, this study enhances our understanding of the genetic architecture underlying complex traits and improves our ability to predict and study complex phenotypes more comprehensively.
更多查看译文
AI 理解论文
溯源树
样例
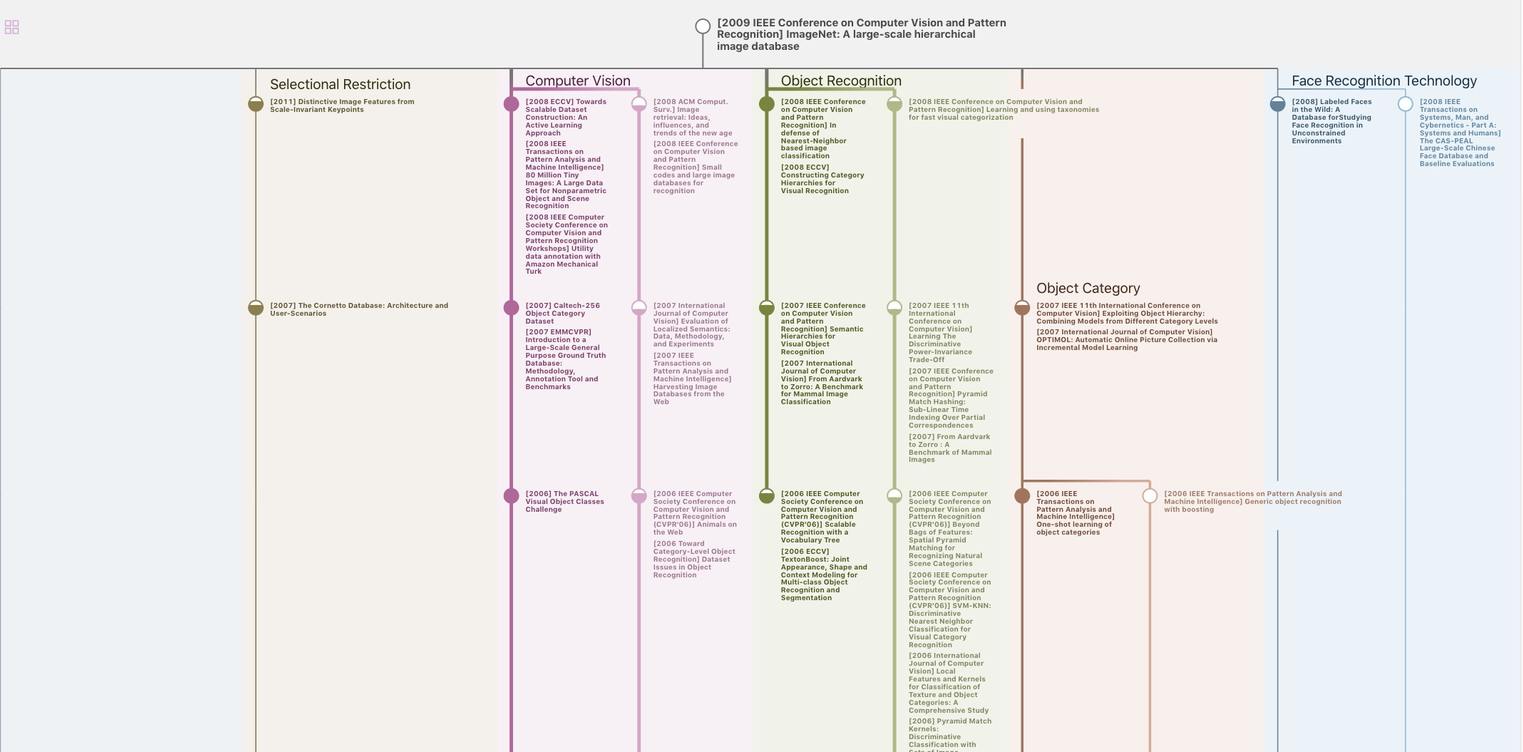
生成溯源树,研究论文发展脉络
Chat Paper
正在生成论文摘要