Decision intelligence-driven predictive modelling of air quality index in surface mining
Engineering Applications of Artificial Intelligence(2024)
摘要
Air pollution emanating from both human activities and natural phenomena, is a pervasive and critical issue that affects everyone. In mining, particularly concerning is the detrimental impact of dust contamination, which not only compromises air quality but also jeopardizes sustainability. In order to establish effective measures for preventing and managing mine dust pollution, it is crucial to have a comprehensive understanding of the persistent characteristics associated with air pollution. In response to this challenge, this study presents an innovative decision intelligence-driven predictive modelling of air quality index (AQI) in surface mining. The framework utilizes machine learning approaches, specifically integrating K-means clustering and random forest (RF) algorithms. A comprehensive dataset of 8928 environmental and operational factors impacting air quality was acquired from the Haerwusu Open-pit Coal Mine (HOCM) in China. The AQI was classified into five distinct levels in accordance with the National Ambient Air Quality Standards (NAAQS) established by the United States Environmental Protection Agency (EPA). The application of the K-means clustering algorithm served to reduce the effects of spectral variation in instances with substantial similarities. Subsequently, the RF method was employed to predict varying AQI levels. The findings revealed by the proposed model demonstrate a remarkable accuracy of 97% in predicting the AQI level in surface coal mining. This study provides improved air quality prediction for optimizing mining operation schedules during favorable weather conditions and implementing dust mitigation strategies, thereby enhancing decision-making processes and promoting green and climate-smart mining.
更多查看译文
关键词
Coal,Surface mining,Air,K-means clustering,Random forest
AI 理解论文
溯源树
样例
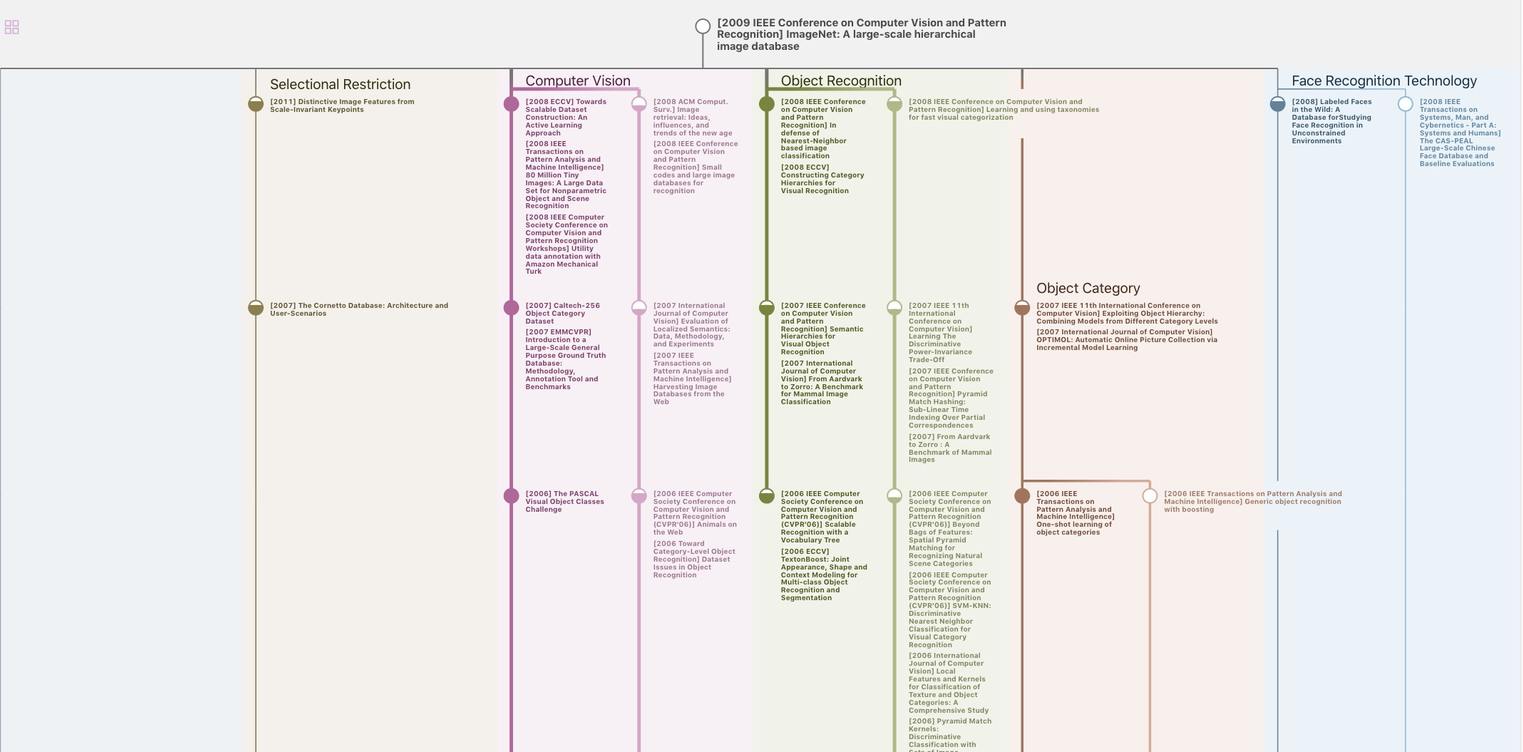
生成溯源树,研究论文发展脉络
Chat Paper
正在生成论文摘要