Balancing innovation and privacy : A machine learning perspective
JOURNAL OF DISCRETE MATHEMATICAL SCIENCES & CRYPTOGRAPHY(2024)
摘要
As digital innovation advances, concerns surrounding privacy escalate. This manuscript explores the intricate relationship between machine learning and privacy preservation. Beginning with a comprehensive literature review, we delve into the current state of privacy in the digital age and examine machine learning's role in addressing these concerns. The manuscript highlights key privacy-preserving techniques, including homomorphic encryption, differential privacy, and federated learning, providing in-depth insights into their applications and real-world implementations. Anticipating future challenges and trends, we recommend maintaining a delicate equilibrium between innovation and privacy in the dynamic landscape of machine learning.
更多查看译文
关键词
Privacy preservation,Machine learning,Digital innovation,Homomorphic encryption,Differential privacy,Federated learning
AI 理解论文
溯源树
样例
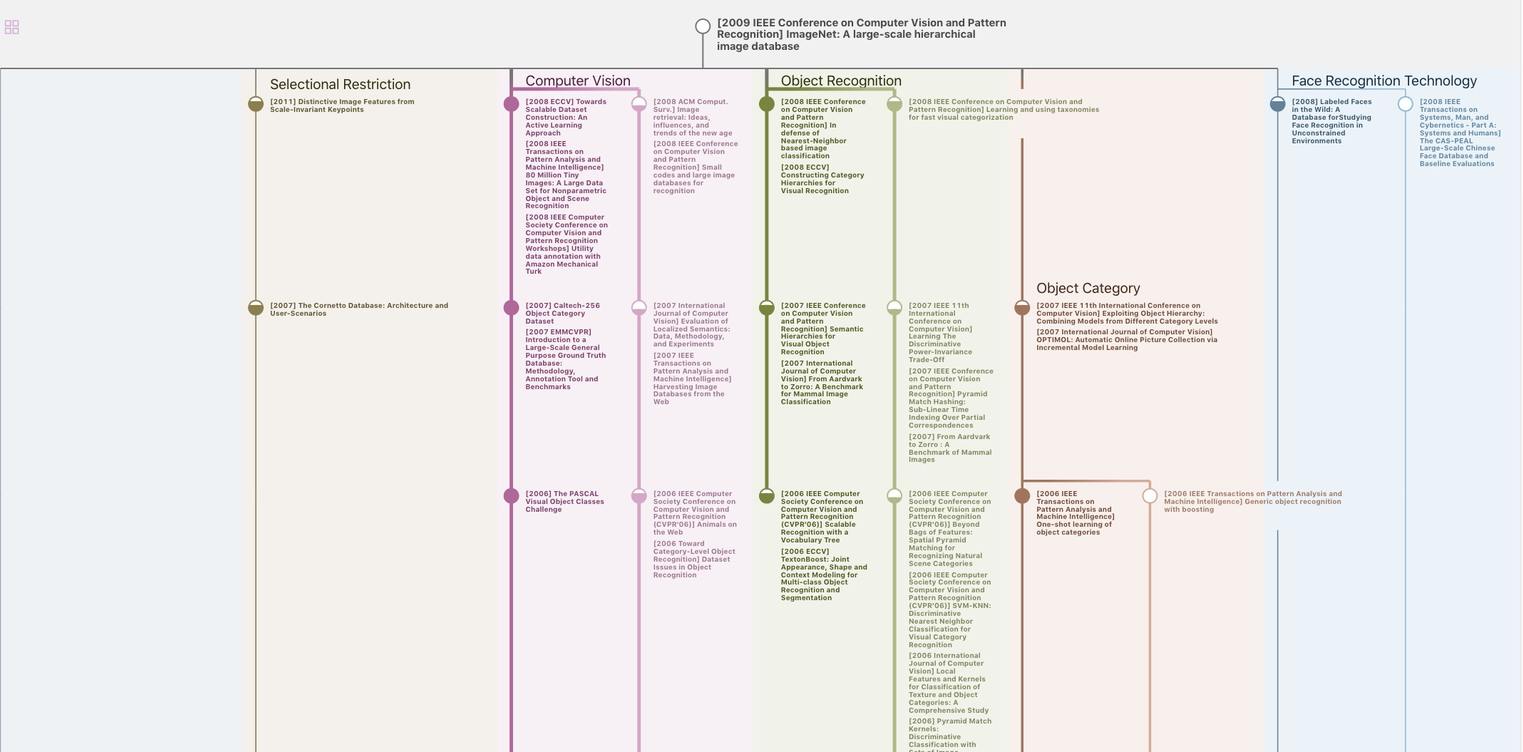
生成溯源树,研究论文发展脉络
Chat Paper
正在生成论文摘要