Local representation-based neighbourhood for robust classification
EXPERT SYSTEMS(2024)
摘要
Representation-based classification (RC) is an effective gauge of data similarity between a single instance and the whole dataset, which extends traditional individual-wise distance metrics using representation coefficients. These coefficients show remarkable discrimination nature via various regularisation terms, but the interference from potentially uncorrelated objects involved in this single-to-global relation can degrade the effectiveness of the coefficients. In order to filter out those unproductive, or even counter-productive, information from the decision making processes, this paper proposes a local representation-based classification (LRC) algorithm to improve the classification accuracy or the RC approach. LRC uses a single-to-local relation induced by the local representation-based neighbourhood (LRN) of each object, rather than the single-to-global relationship used by RC. Thanks to LRN, a compact and relevant dataset can be formed by selecting the most relevant data instances in the original dataset, to render a robust representation of a query. LRC was applied to multiple publicly available datasets, and the experimental results demonstrate the superiority of the proposed LRC algorithm as evidenced by the higher classification accuracy and more noise-tolerant capability in reference to alternative RC approaches. Moreover, the sampling ability of LRN is also verified via a comparative study.
更多查看译文
关键词
neighbourhood relation,regularisation,representation-based classification,robustness
AI 理解论文
溯源树
样例
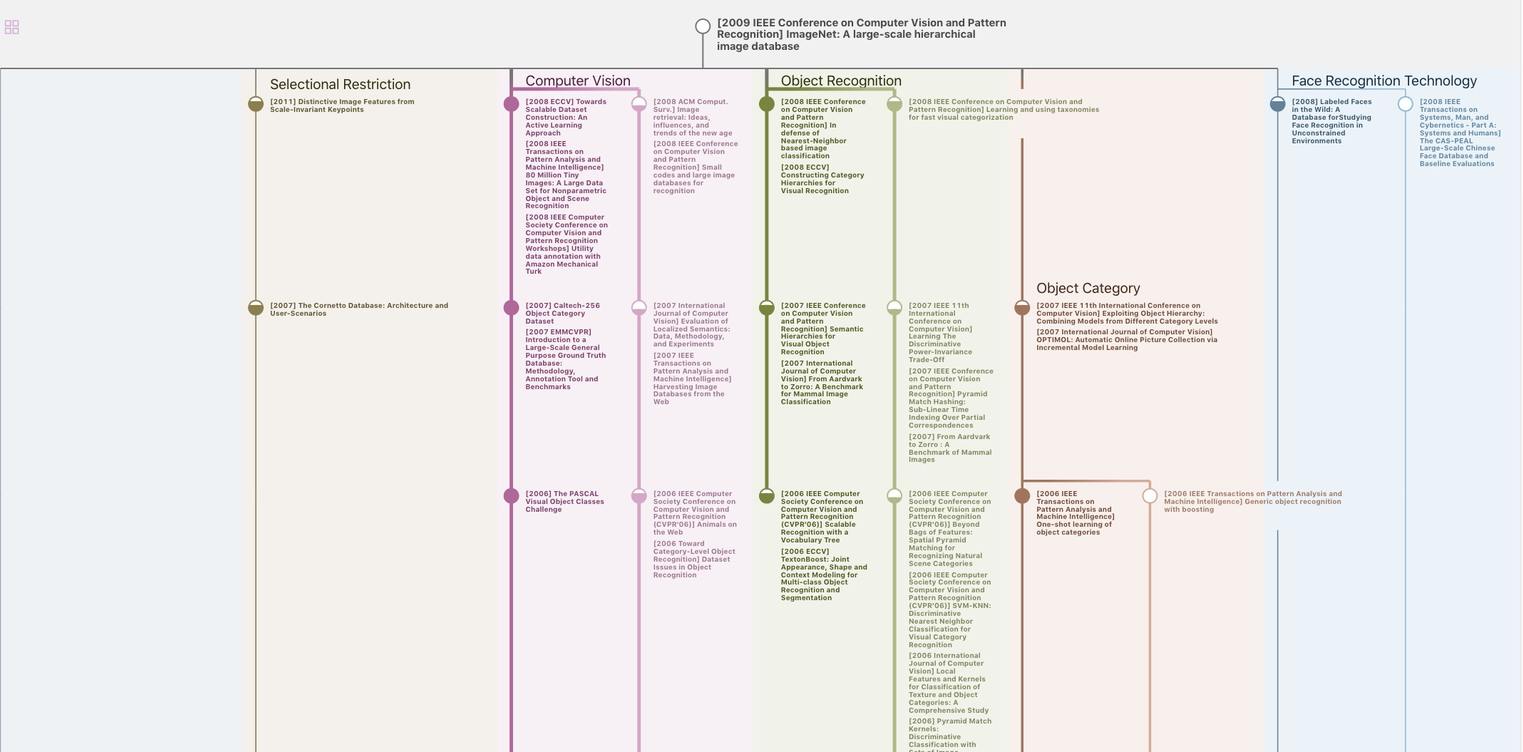
生成溯源树,研究论文发展脉络
Chat Paper
正在生成论文摘要