Lane-Changing Intention Recognition Based on Multivehicle Interaction Dynamic Graph Modeling in a Connected Environment
JOURNAL OF TRANSPORTATION ENGINEERING PART A-SYSTEMS(2024)
摘要
Accurate and proactive lane-changing (LC) intention recognition can assist drivers in making LC decisions to improve driving safety. However, the mechanism of drivers' LC decisions in dynamically changing environments is still not fully understood, which makes it difficult for advanced driver-assistance systems (ADASs) to make accurate LC decisions under different working conditions. To accurately capture the dynamic features before the generation of LC intention, relying on the multivehicle interaction capability of the connected environment, a LC intention recognition framework using graph theory to model the interaction relationship among multiple vehicles, i.e., the Multivehicle Interaction Dynamic Time Graph (MIDTG) framework, is proposed. First, the interaction relationship between LC vehicles and their surrounding vehicles in the connected communication range is modeled by graph theory. Second, the graph convolutional network (GCN) is used to extract spatial features of multivehicle interactions, and a long short-term memory (LSTM) neural network is used to learn the association of multivehicle interaction graphs in a time series. Finally, LC intentions are output through the Softmax function. The highD data set is used to validate the proposed model. Results show that the model can accurately extract the dynamic features of multivehicle interactions within a time window of 2.5 to 3.5 s, and the accuracy of LC intention recognition reaches 98%, which is an average improvement of 3.5% compared with other baseline models. The study provides a new way to model multivehicle interactions in the connected environment, which can be helpful for ADASs' LC decision-making. The significance of this study is to provide guidance for advanced driver-assistance systems (ADASs) to better assist drivers in making lane-changing decisions. In this study, a novel multivehicle interaction modeling method based on graph theory is proposed, combined with an advanced deep learning method to extract the spatiotemporal characteristics of multivehicle interaction, and finally applied to lane-changing intention recognition. The results show that the proposed method achieves good lane-changing intention recognition accuracy and can accurately extract key information from multivehicle interactions. This will provide a new way to predict driving behavior in a connected environment. This study will be meaningful for traffic operators and can spur a new wave of innovative applications in ADASs, such as lane-changing alerts.
更多查看译文
关键词
Lane changing,Intention recognition,Multivehicle interaction,Graph convolutional neural network,Long short-term memory (LSTM) neural network
AI 理解论文
溯源树
样例
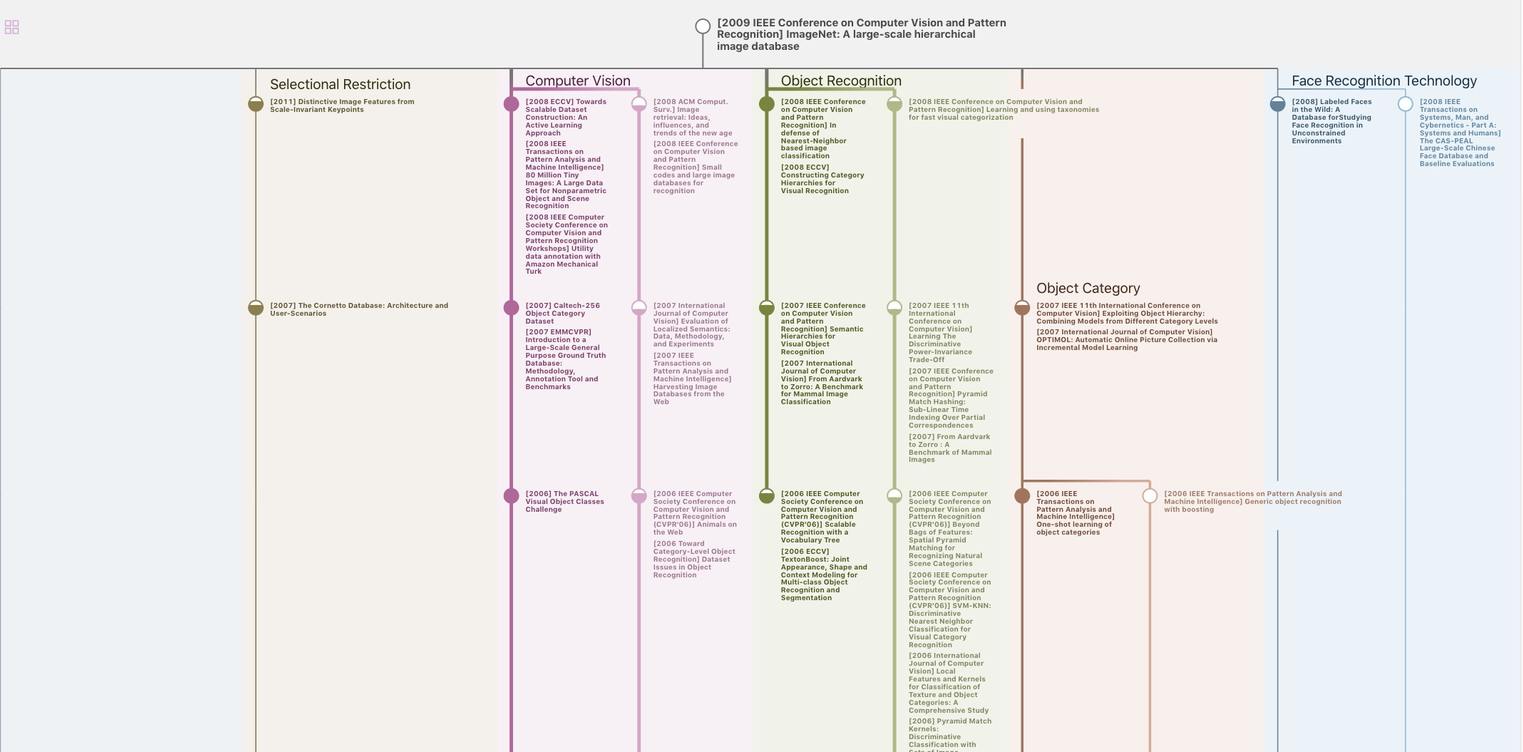
生成溯源树,研究论文发展脉络
Chat Paper
正在生成论文摘要