Results of the 2023 ISBI challenge to reduce GABA-edited MRS acquisition time
Magnetic Resonance Materials in Physics, Biology and Medicine(2024)
摘要
Use a conference challenge format to compare machine learning-based gamma-aminobutyric acid (GABA)-edited magnetic resonance spectroscopy (MRS) reconstruction models using one-quarter of the transients typically acquired during a complete scan. There were three tracks: Track 1: simulated data, Track 2: identical acquisition parameters with in vivo data, and Track 3: different acquisition parameters with in vivo data. The mean squared error, signal-to-noise ratio, linewidth, and a proposed shape score metric were used to quantify model performance. Challenge organizers provided open access to a baseline model, simulated noise-free data, guides for adding synthetic noise, and in vivo data. Three submissions were compared. A covariance matrix convolutional neural network model was most successful for Track 1. A vision transformer model operating on a spectrogram data representation was most successful for Tracks 2 and 3. Deep learning (DL) reconstructions with 80 transients achieved equivalent or better SNR, linewidth and fit error compared to conventional 320 transient reconstructions. However, some DL models optimized linewidth and SNR without actually improving overall spectral quality, indicating a need for more robust metrics. DL-based reconstruction pipelines have the promise to reduce the number of transients required for GABA-edited MRS.
更多查看译文
关键词
Benchmarking,Computer,Deep learning,Magnetic resonance spectroscopy,Neural networks
AI 理解论文
溯源树
样例
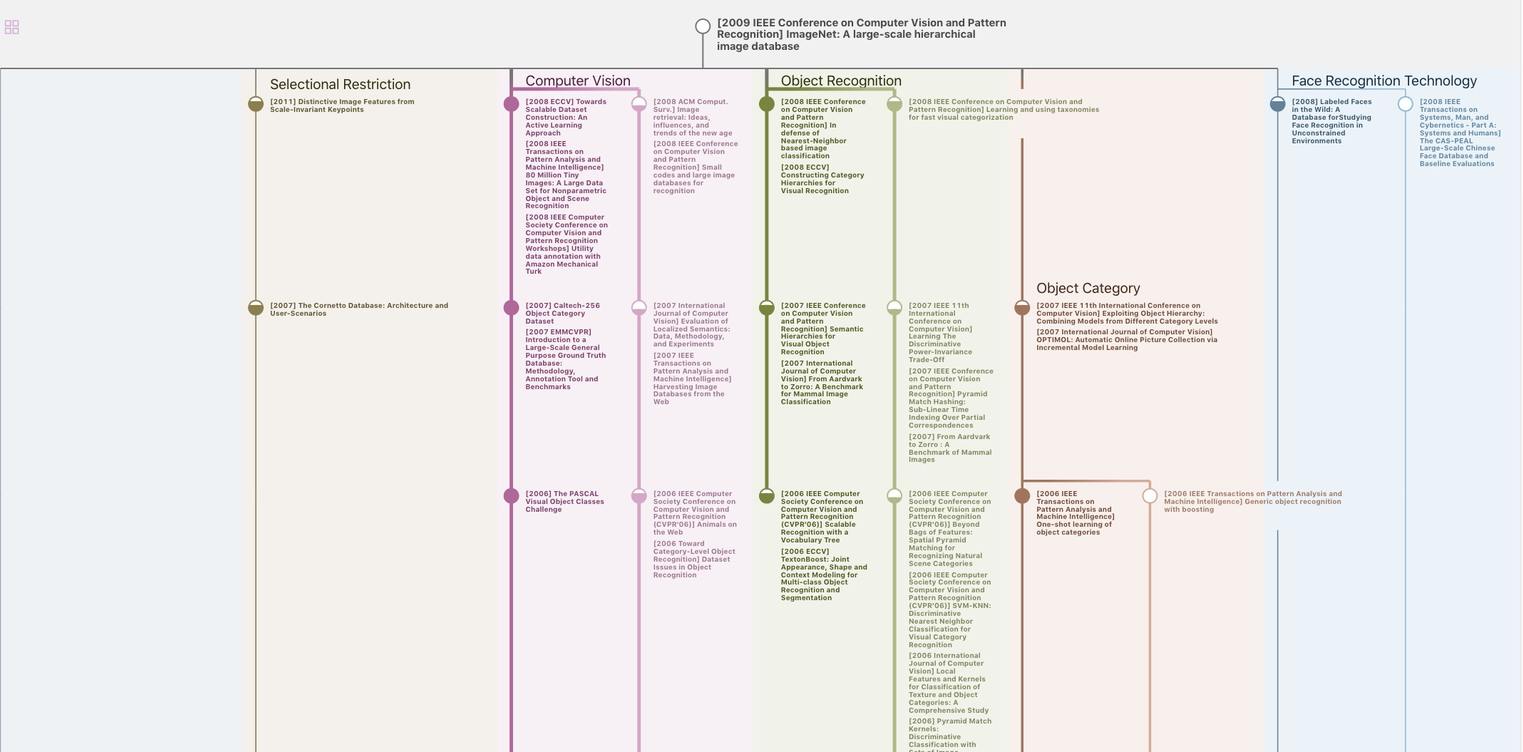
生成溯源树,研究论文发展脉络
Chat Paper
正在生成论文摘要