Improved Consistency of Satellite XCO2 Retrievals Based on Machine Learning
GEOPHYSICAL RESEARCH LETTERS(2024)
Abstract
Quantifying atmospheric CO2 over long periods from space is crucial in understanding the carbon cycle's response to climate change. However, a single satellite offers limited spatiotemporal coverage, making comprehensive monitoring challenging. Moreover, biases among various satellite retrievals hinder their direct integration. This study proposed a machine learning framework for fusing the column-averaged dry-air mole fraction of CO2 (XCO2) retrievals from Greenhouse Gases Observing Satellite (GOSAT) and OCO-2 satellites. The best model (R-2 = 0.85) presented improved consistency of GOSAT retrievals by reducing 71.5% of the average monthly bias while using OCO-2 retrievals as a benchmark, indicating the fusion data set's potential to enhance observation coverage. Incorporating the adjusted GOSAT XCO2 retrievals into the OCO-2 data set added an average of 84.7 thousand observations annually, enhancing the yearly temporal coverage by 53.6% (from 14 to 21.5 days per grid). This method can be adapted to other satellites, maximizing satellite resources for a more robust carbon flux inversion.
MoreTranslated text
Key words
data fusion,machine learning,satellite observation,XCO2 retrieval,carbon cycle
AI Read Science
Must-Reading Tree
Example
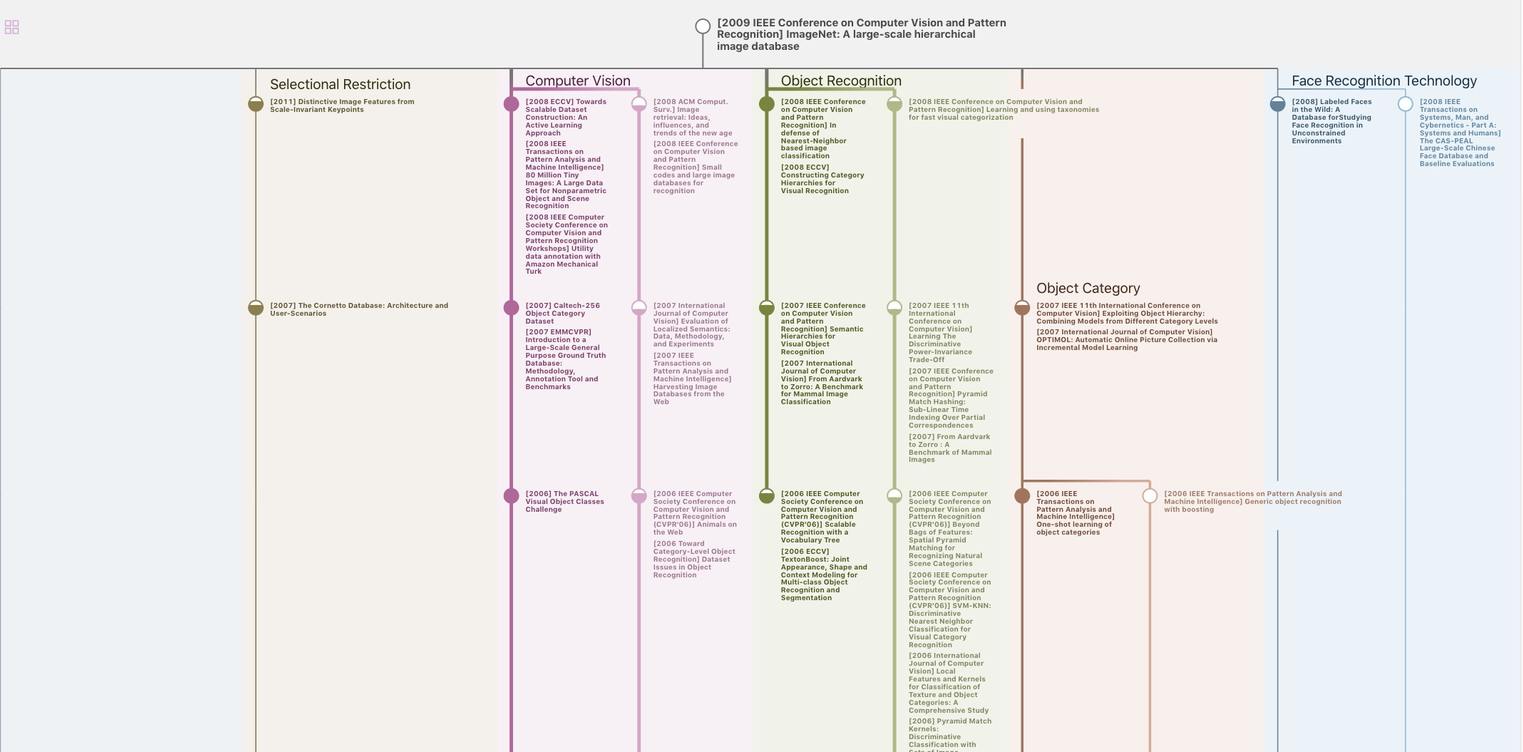
Generate MRT to find the research sequence of this paper
Chat Paper
Summary is being generated by the instructions you defined