F2M: Ensemble-based uncertainty estimation model for fire detection in indoor environments
ENGINEERING APPLICATIONS OF ARTIFICIAL INTELLIGENCE(2024)
摘要
Early fire detection and timely notification are paramount for preventing human and material casualties caused by fire. As a result, scientists have developed various fire monitoring systems based on sensors and images. Image -based systems have proven more advantageous than sensor -based ones as they provide additional details about the fire, such as its location, intensity, and progression. However, accurately determining the shape and boundaries of flames in image -based systems is difficult due to various factors, including backgrounds, different fire sizes, and interference from objects that resemble flames. In this study, we first evaluate convolutional neural networks used in related research and benchmark them on our challenging indoor fire dataset. Secondly, we propose a novel Feature Merging Model (F2M), which combines the output fire segmentation masks of the top five segmentation models obtained through the performed evaluation. The F2M relies on a novel mechanism that introduces bias into the mask estimation and achieves better results than all the tested methods, along with Monte -Carlo dropout for uncertainty estimation. The F2M ensemble -based uncertainty estimation model achieved improvements in comparison to the best performance convolutional neural network U -Net++, on three different image resolutions: 256 x 256, 640 x 640 and 800 x 800, according to the following metrics: Total error, Dice coefficient, and IoU score.
更多查看译文
关键词
Fire dataset,Fire detection,Indoor,Machine learning,Uncertainty estimation,Semantic segmentation,Benchmark
AI 理解论文
溯源树
样例
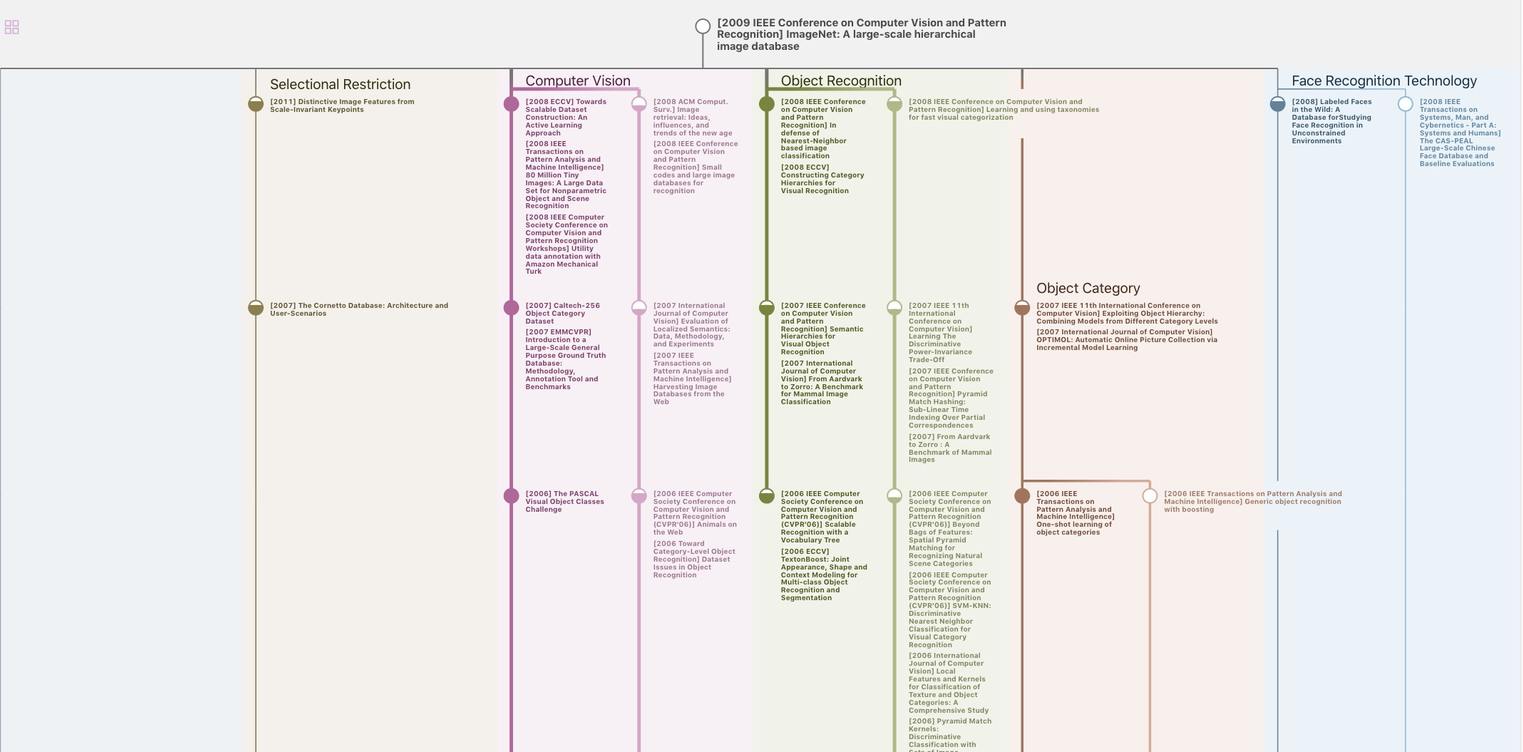
生成溯源树,研究论文发展脉络
Chat Paper
正在生成论文摘要