Compressive strength and sensitivity analysis of fly ash composite foam concrete: Efficient machine learning approach
ADVANCES IN ENGINEERING SOFTWARE(2024)
Abstract
This study aims to propose a reliable machine learning model for predicting the compressive strength of fly ash composite foam concrete (FFC), improving the waste of work time, cost and resources due to over -testing. Firstly, 320 groups of FFC samples were collected from the previous literature to establish the database, which contained eight feature variables such as wet density, cement content, fly ash content, sand content, water-cement ratio, foam content, curing age and compressive strength. Secondly, the database was pre -processed by Z-score method to improve the data quality. Then, the pre -processed database were trained and tested based on eight machine learning algorithms (such as multiple linear, Bayesian regression, K-nearest neighbor, decision tree, random forest, support vector machine, gradient boosting and deep neural network), and their differences, advantages and disadvantages were compared. Finally, the shaply additive explanation and partial dependence plot were applied to explain the potential effects of input variables for the compressive strength development. The test results show that the dispersion of database after pre-processing is significantly improved, and feature variables show normal distribution. The support vector machine model has the best generalization performance, and its prediction accuracy and error for the test set of FFC are minimum (the coefficient of determination is 0.95). The key influential factors of FFC compressive strength are wet density, cement content, sand content, foam content and water-binder ratio, distributing in 600 kg/m3 - 2400 kg/m3, 20 % - 80 %, 0 % - 70 %, 0 % - 40 % and 0.1 - 0.8, respectively.
MoreTranslated text
Key words
Foam concrete,Fly ash,Machine learning,Compressive strength,Sensitivity analysis
AI Read Science
Must-Reading Tree
Example
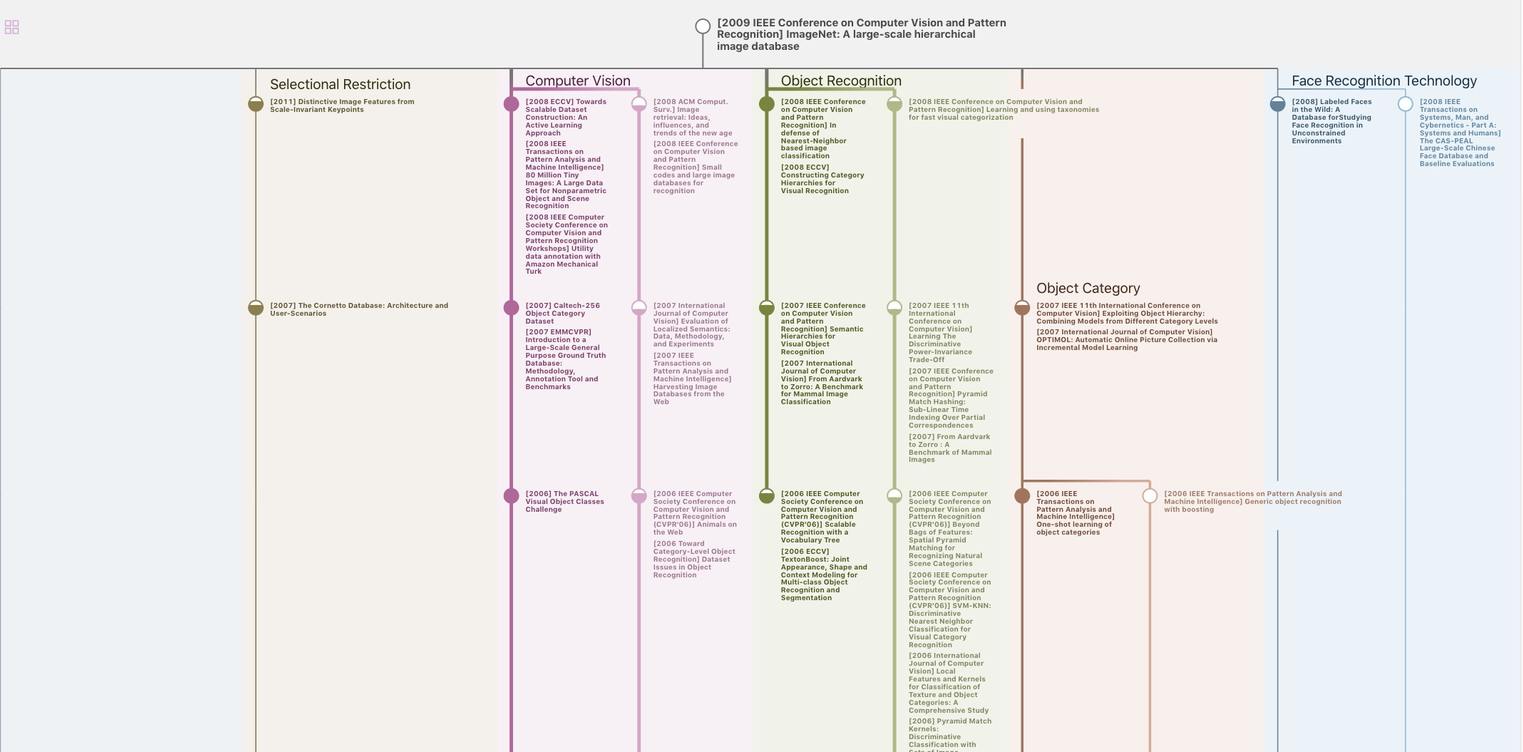
Generate MRT to find the research sequence of this paper
Chat Paper
Summary is being generated by the instructions you defined