A multi-time-scale multi-step prediction method for gas utilization rate in a BF based on just-in-time learning
Control Engineering Practice(2024)
摘要
Gas utilization rate (GUR) is an important indicator of the blast furnace (BF) condition. Its accurate prediction provide guidance for the BF industrial practitioners. GUR has complex multi-time-scale characteristics, and the performance and accuracy of its prediction models require improvement. This paper presents a multi-time-scale just-in-time learning multi-step prediction method to continuously optimize the prediction model on multi-time scales for predicting the future GUR. First, this paper describes BF operating parameters that affect the development of GUR by causal analysis and selects two parameters combined with the information entropy. Next, this paper presents a BF dynamic time warping distance method to calculate the optimal distance between operating parameters and GUR, and accurately divides different time scales from qualitative and quantitative perspectives. Then, this paper presents a just-in-time learning multi-step GUR prediction model to improve the performance, which provides a model updating method and a more accurate prediction model for predicting long-term future GUR. Finally, some experimental results based on actual production data show that the method predicts the GUR accurately in the complex production environment.
更多查看译文
关键词
Blast furnace,Gas utilization rate,Multi-time scales,Dynamic time warping distance,Causal analysis,Just-in-time learning,Multi-step prediction
AI 理解论文
溯源树
样例
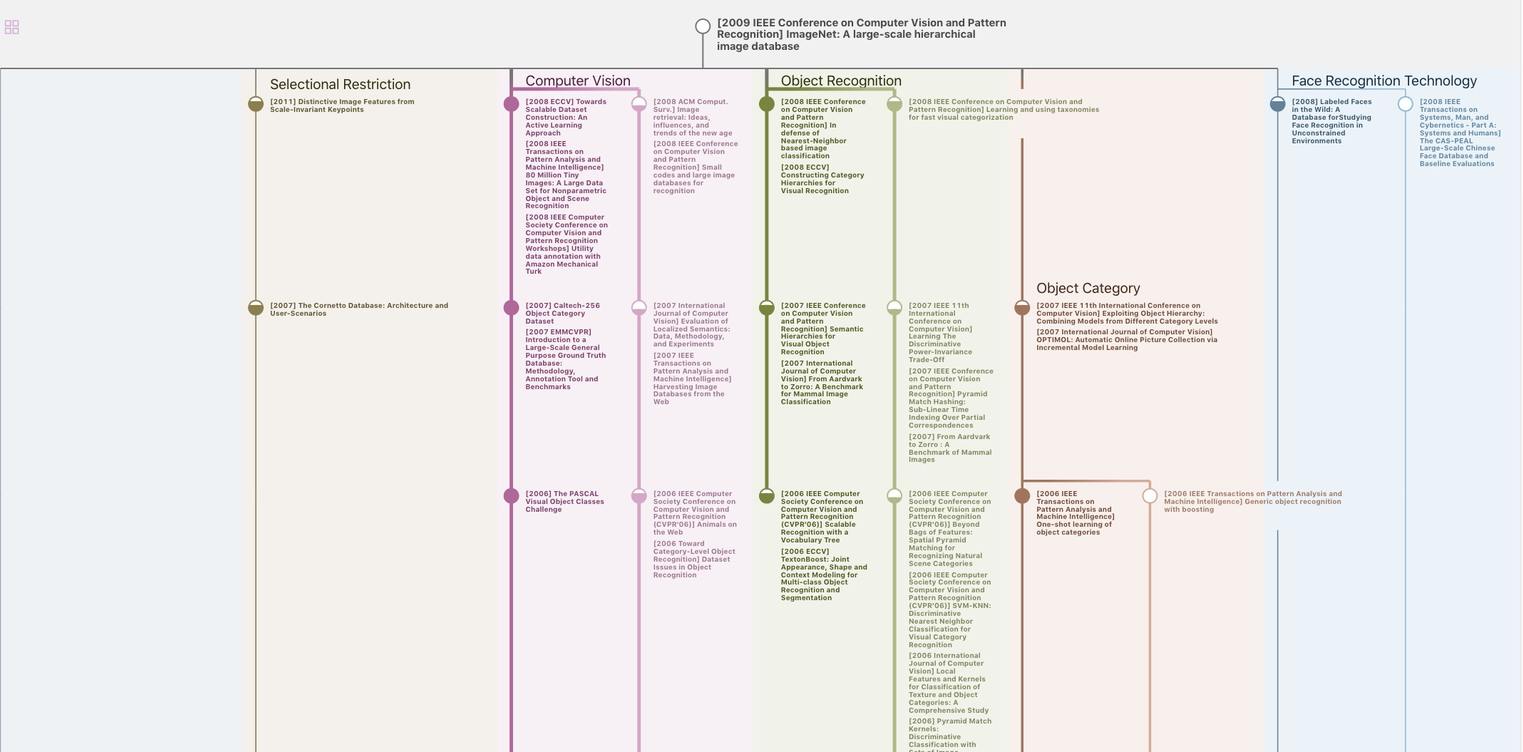
生成溯源树,研究论文发展脉络
Chat Paper
正在生成论文摘要