Towards multi-scene learning: A novel cross-domain adaptation model based on sparse filter for traction motor bearing fault diagnosis in high-speed EMU
Advanced Engineering Informatics(2024)
摘要
Fault diagnosis of traction motor bearing is of great significance to improve the reliability and safety of high-speed electric multiple units (EMU). While the fault diagnosis method based on cross-domain adaptation has been successful in scenarios involving speed or load fluctuations, existing methods ignore the independence and diversity of features, resulting in unsatisfactory diagnostic results under multi-scene learning, thereby reducing the generalization ability. Moreover, the development of complex models is time-consuming, and their computational efficiency is low. To address these issues, this study proposes a novel cross-domain adaptation model based on sparse filtering (SFCDA), which consists of only two fully connected (FC) layers. Firstly, pre-training is conducted to utilize the soft reconstruction penalties to constrain the weights of sparse filtering and improve the independence of features. The weights of unsupervised training are used to initialize the parameters of the first FC layer of the SFCDA model. Secondly, a multiple sparse regularization (MSR) algorithm is proposed and used to constrain the SFCDA. Then, fine-tuning is conducted, in which the structural alignment function is used to measure the distribution distance between the source and target domain data. Minimizing the kernel norm can improve the diversity of features and enhance the robustness. Finally, the effectiveness of SFCDA in multi-scene learning is proved theoretically. It is validated in three fault diagnosis scenes in four different bearing datasets. The results show that the suggested approach is more straightforward and has a better fault diagnosis effect than the state-of-the-art domain adaptive approaches.
更多查看译文
关键词
Bearing fault diagnosis,Sparse filter,Cross-domain adaptation
AI 理解论文
溯源树
样例
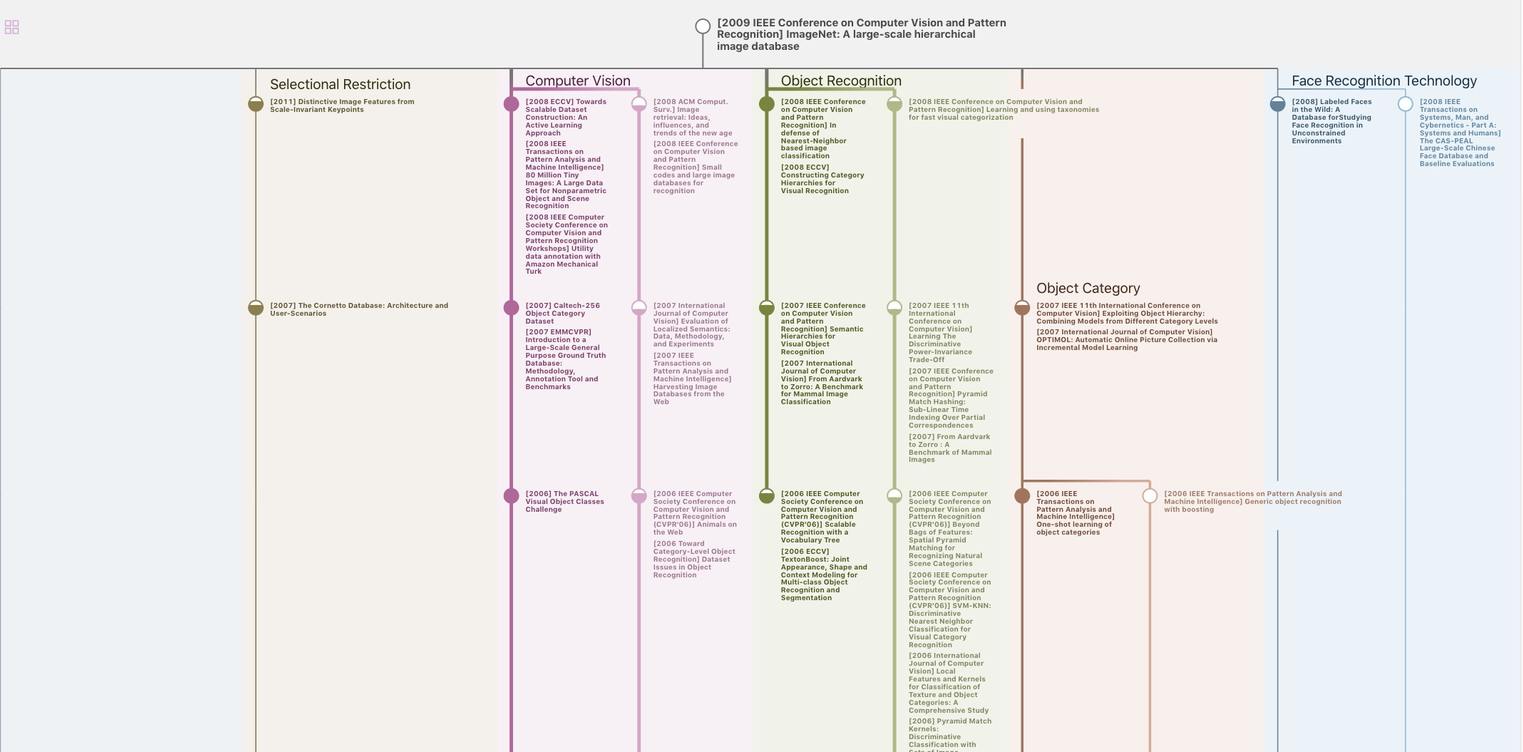
生成溯源树,研究论文发展脉络
Chat Paper
正在生成论文摘要