Deep learning for the automatic detection and segmentation of parotid gland tumors on MRI
Oral Oncology(2024)
摘要
Objectives
Parotid gland tumors (PGTs) often occur as incidental findings on magnetic resonance images (MRI) that may be overlooked. This study aimed to construct and validate a deep learning model to automatically identify parotid glands (PGs) with a PGT from normal PGs, and in those with a PGT to segment the tumor.
Materials and methods
The nnUNet combined with a PG-specific post-processing procedure was used to develop the deep learning model trained on T1-weighed images (T1WI) in 311 patients (180 PGs with tumors and 442 normal PGs) and fat-suppressed (FS)-T2WI in 257 patients (125 PGs with tumors and 389 normal PGs), for detecting and segmenting PGTs with five-fold cross-validation. Additional validation set separated by time, comprising T1WI in 34 and FS-T2WI in 41 patients, was used to validate the model performance.
Results and conclusion
To identify PGs with tumors from normal PGs, using combined T1WI and FS-T2WI, the deep learning model achieved an accuracy, sensitivity and specificity of 98.2% (497/506), 100% (119/119) and 97.7% (378/387), respectively, in the cross-validation set and 98.5% (67/68), 100% (20/20) and 97.9% (47/48), respectively, in the validation set. For patients with PGTs, automatic segmentation of PGTs on T1WI and FS-T2WI achieved mean dice coefficients of 86.1% and 84.2%, respectively, in the cross-validation set, and of 85.9% and 81.0%, respectively, in the validation set. The proposed deep learning model may assist the detection and segmentation of PGTs and, by acting as a second pair of eyes, ensure that incidentally detected PGTs on MRI are not missed.
更多查看译文
关键词
Parotid gland tumors,Non-contrast-enhanced MRI,Deep learning,Automatic tumor detection and segmentation
AI 理解论文
溯源树
样例
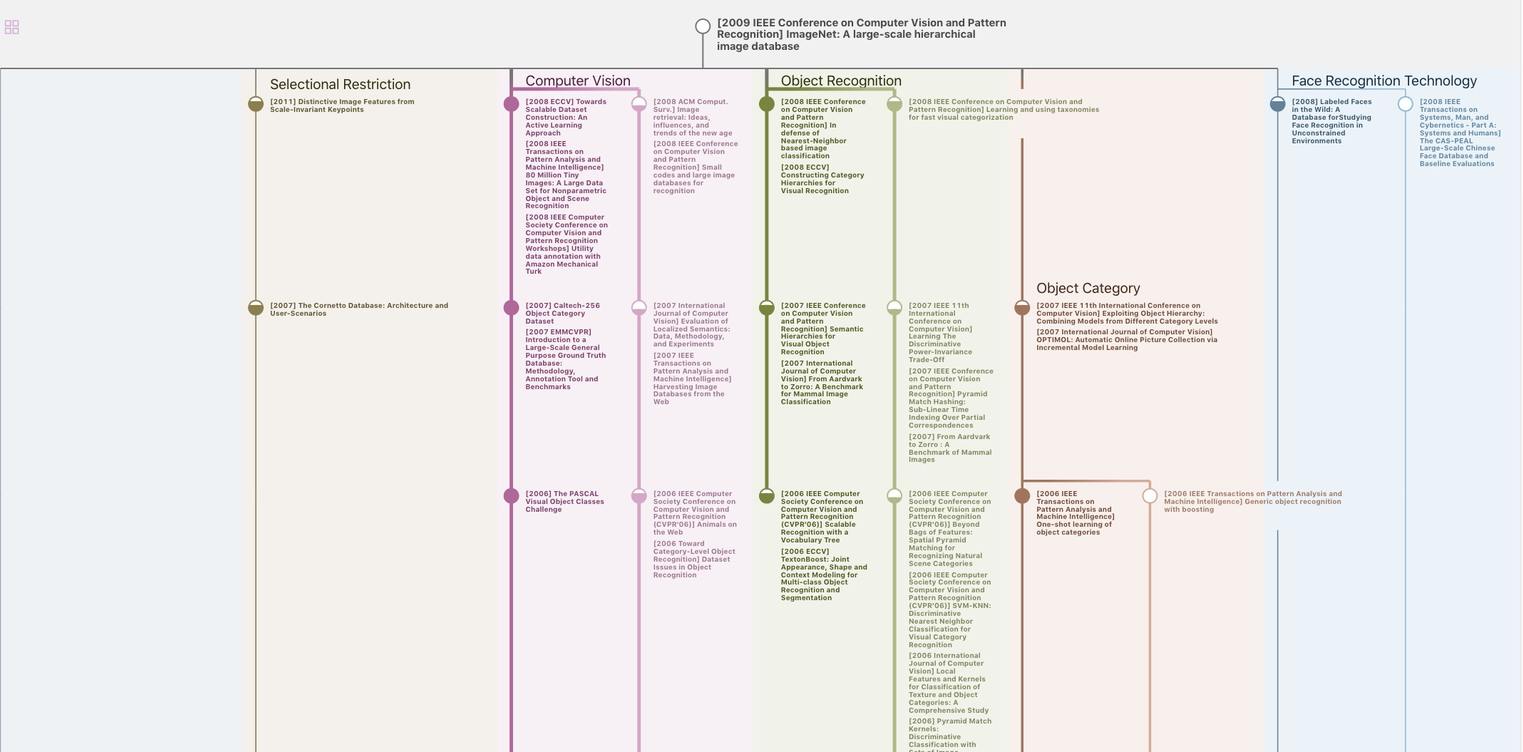
生成溯源树,研究论文发展脉络
Chat Paper
正在生成论文摘要