Enhancing Monocular 3D Object Detection through Data Augmentation Strategies
IEEE Transactions on Instrumentation and Measurement(2024)
摘要
Data augmentation is a crucial component of machine learning. In 2D object detection tasks, it can significantly enhance the performance of detectors without increasing the inference cost. Data augmentation methods, such as random translation and random resizing, have become standard practices for 2D object detectors. However, in monocular 3D object detection tasks, the data augmentation methods used in 2D object detection cannot be directly applied due to the different representations of object positions. In this study, a method is proposed to migrate a 2D object detection data enhancement method to monocular 3D object detection while preserving coordinate and size cues. Additionally, we address the sampling bias problem associated with data augmentation in this process. We introduce an unbiased sampling strategy and several new augmentation methods specifically designed for monocular 3D object detection. Our proposed method achieves a performance of 20.47% AP
3D
(IOU=0.7, Car, Moderate) on the KITTI dataset and a speed of 45 FPS on RTX 2080Ti GPUs, outperforming all previous monocular methods. The source codes are at: https://github.com/jiayisong/DA3D.
更多查看译文
关键词
Monocular 3D object detection,deep learning,autonomous driving,data augmentation
AI 理解论文
溯源树
样例
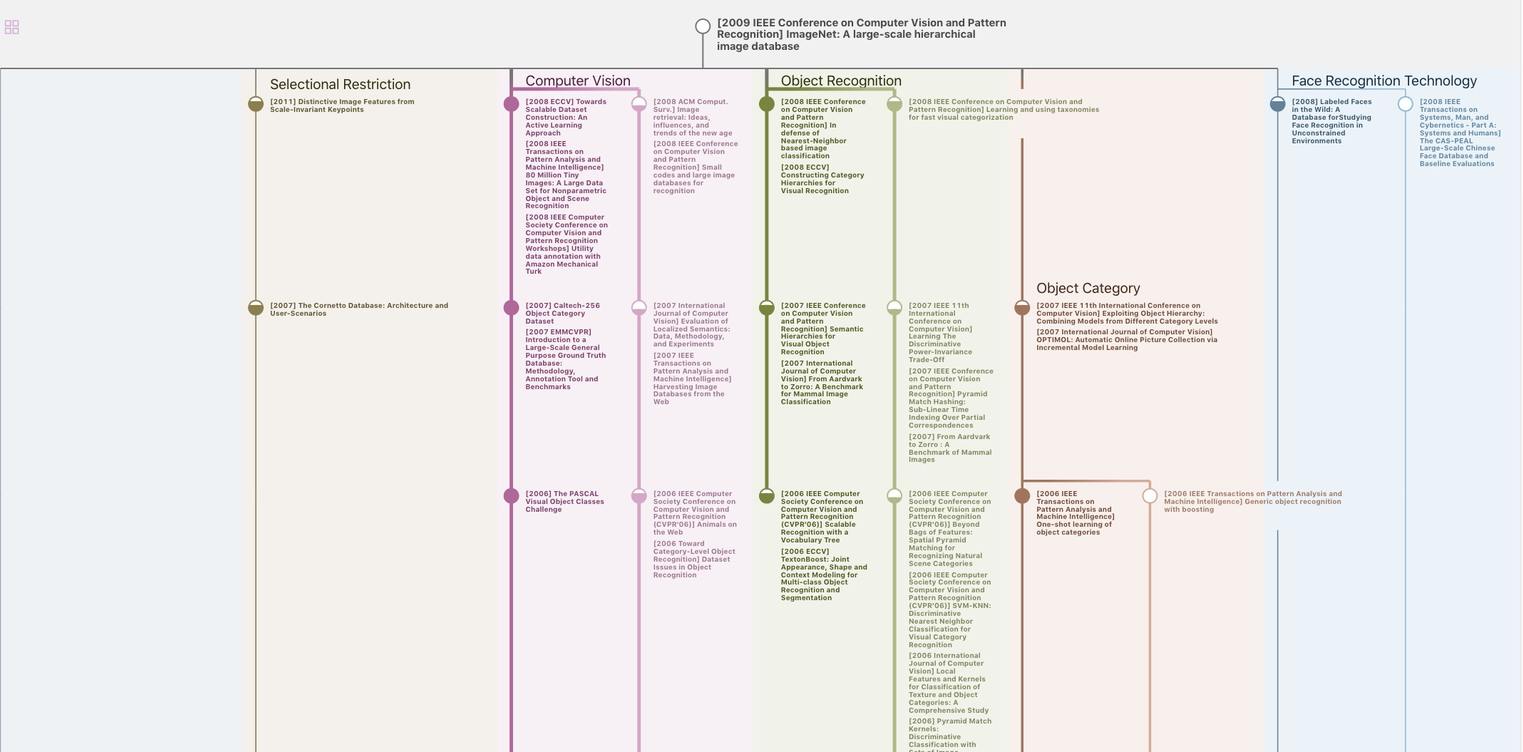
生成溯源树,研究论文发展脉络
Chat Paper
正在生成论文摘要