Early remaining-useful-life prediction applying discrete wavelet transform combined with improved semi-empirical model for high-fidelity in battery energy storage system
Energy(2024)
摘要
The recycling of lithium-ion batteries (LIBs) from electric vehicles (EVs) for augmenting the capacity of battery energy storage systems (BESS) presents a sustainable approach to leverage investment in LIBs, mitigating economic losses. This study highlights the critical role of accurate capacity estimation and remaining-useful-life (RUL) prediction in determining optimal replacement and augmentation schedules. We introduce a semi-empirical (SE) model that integrates experimental aging data with an adaptive method and an electrochemical model to capture the degradation trends of LIBs accurately. The adoption of the Moore-Penrose pseudoinverse (Pinv) method, suited for the SE model's multi-parameter, single-output nature, significantly improves capacity estimation accuracy, achieving a maximum error below 0.2 Ah. This improved SE (ISE) model accounts for capacity trends and addresses non-stationary phenomena, including noise and signal distortion during parameter identification and capacity recovery phases.To address these challenges, we propose using discrete wavelet transform (DWT) for the decomposition of non-stationary signals, adjusting the window size to signal characteristics for enhanced noise reduction. The application of DWT to denoise α and β parameters, characterized by their non-constant periodicity and noise interference, facilitates the development of DWT combined with the ISE (DWT-ISE) aging model for early RUL prediction. Experimental validation reveals that our DWT-based approach minimizes root-mean square error (RMSE) and mean absolute error (MAE) for early RUL prediction, demonstrating the model's superiority at 300 cycles for identification and achieving the lowest prediction error at 400 cycles. Furthermore, comparison results of other models (Particle filter, and long short-term memory) reveal that our DWT-ISE aging model exhibits the best RMSE and MAE on limited data. Specifically, the RMSE and MAE values for RUL prediction, underscored as 0.032% and 0.027% respectively at 300 cycles, are confirmed as the highest evaluation metrics.
更多查看译文
关键词
Lithium-ion battery,Recycling of reused batteries,Semi-empirical aging model,Moore-Penrose pseudoinverse matrix,Discrete wavelet transform,Early remaining-useful-life prediction
AI 理解论文
溯源树
样例
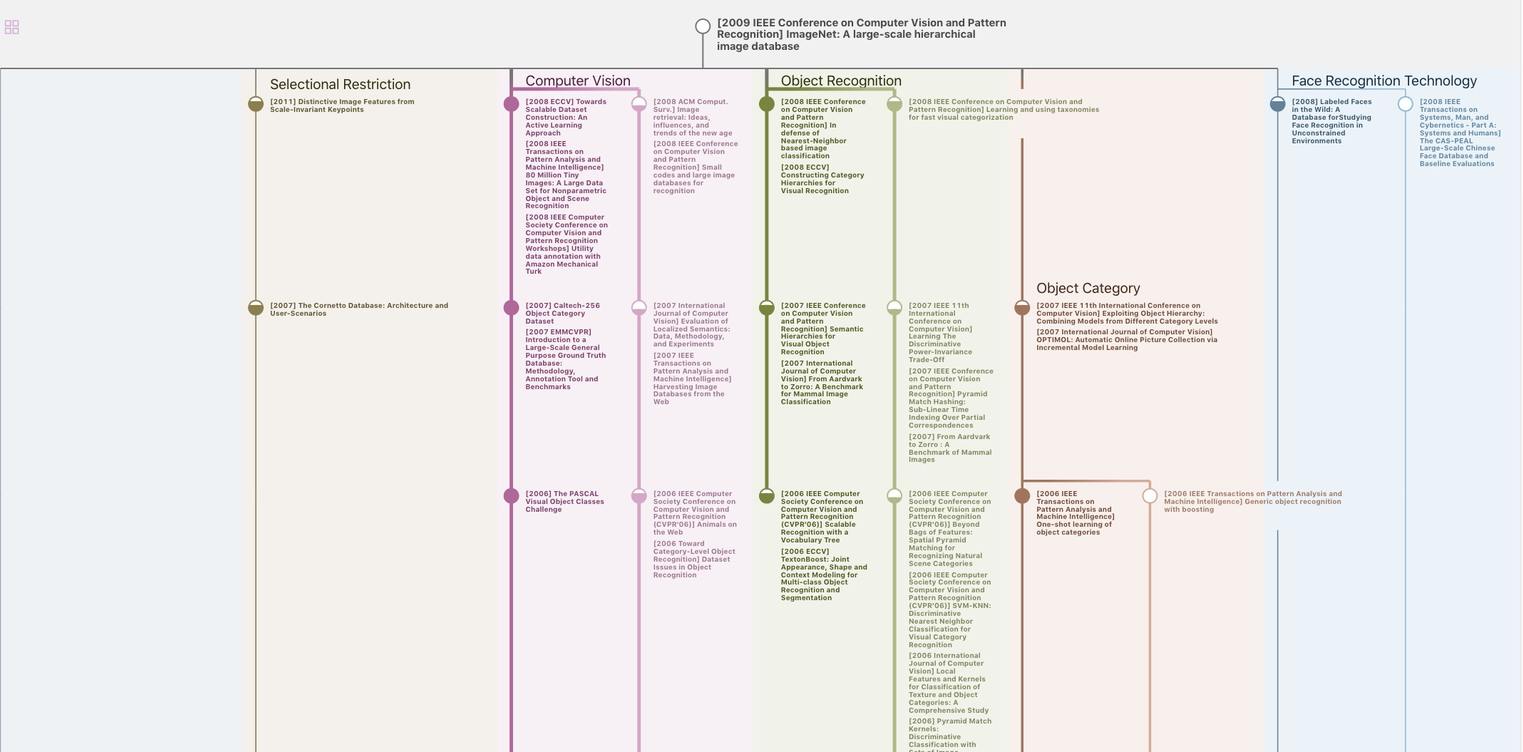
生成溯源树,研究论文发展脉络
Chat Paper
正在生成论文摘要