SAW-GAN: Multi-granularity Text Fusion Generative Adversarial Networks for text-to-image generation
Knowledge-Based Systems(2024)
摘要
Text-to-image generation is a challenging task that aims to generate visually realistic images semantically consistent for a given text. Existing methods mainly exploit the global semantic information of a single sentence while ignoring fine-grained semantic information such as aspects and words, which are critical factors in bridging the semantic gap in text-to-image generation. We propose a Multi-granularity Text (Sentence-level, Aspect-level, and Word-level) Fusion Generative Adversarial Network (SAW-GAN), which comprehensively represents textual information from multiple granularities. To effectively fuse multi-granularity information, we design a Double-granularity-text Fusion Module (DFM) fusing sentence and aspect information through parallel affine transformation and a Triple granularity-text Fusion Module (TFM) fusing sentence, aspect and word information by designing a novel Coordinate Attention Module (CAM), which can precisely locate the visual areas associated with each aspect and word. Furthermore, we use CLIP (Contrastive Language-Image Pre-training) to provide visual information to bridge the semantic gap and improve the model’s generalization ability. Our results show significant performance improvements over state-of-the-art methods using Conditional Generation Adversarial Network (CGAN) on CUB (FID from 13.91 to 10.45) and COCO (FID from 14.60 to 11.17) datasets with photorealistic images of richer details and text-image consistency.
更多查看译文
关键词
Text-to-image generation,Text-image information fusion,Attention mechanism,CLIP
AI 理解论文
溯源树
样例
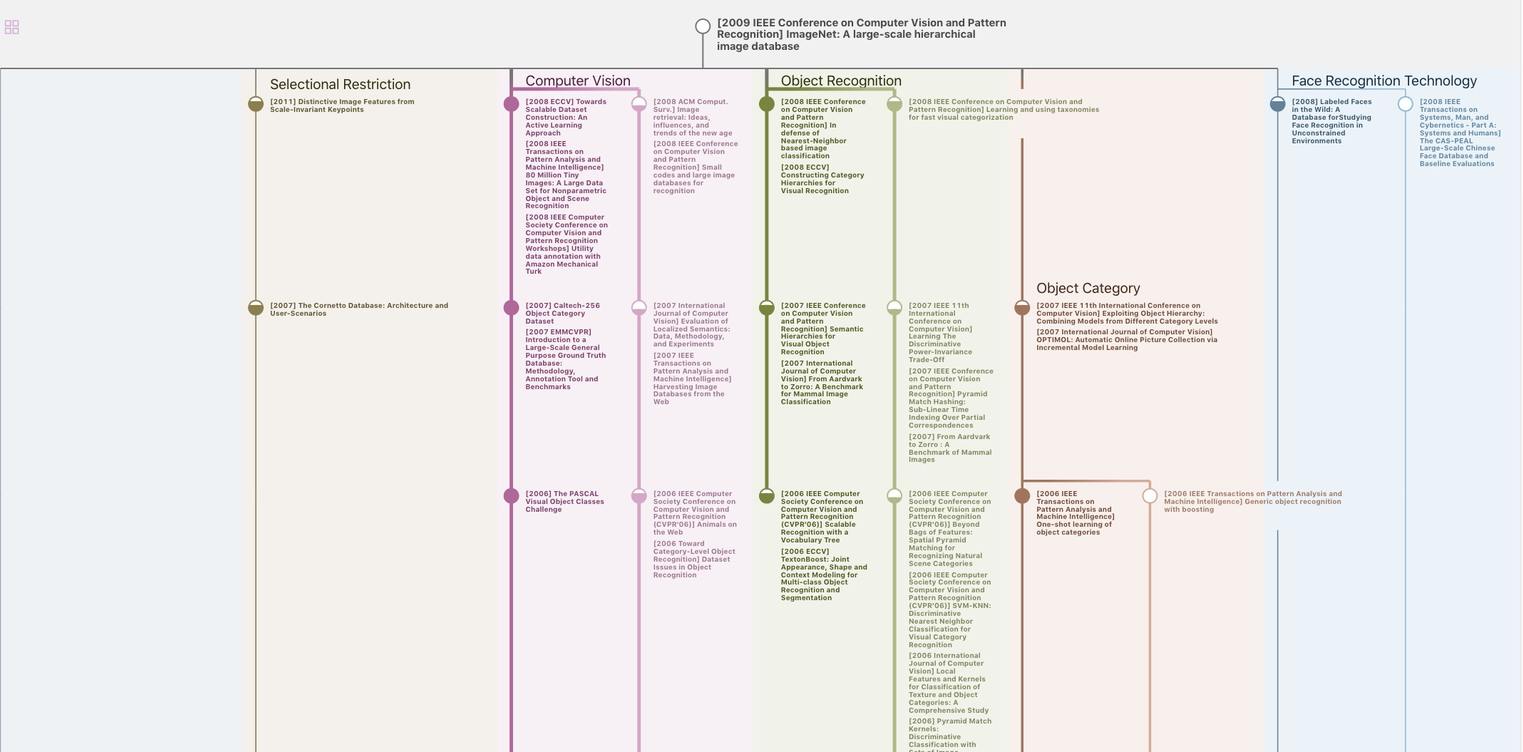
生成溯源树,研究论文发展脉络
Chat Paper
正在生成论文摘要