An evolutionary approach to extreme individual impact opinions based on time sunk costs
Intelligent Data Analysis(2024)
摘要
Large-scale studies indicate that the distinct approach to opinion fusion employed by extreme agents exerts a more potent influence on overall opinion evolution when compared to regular agents. The presence of extreme agents within the network tends to undermine the development of opinion neutrality, which is harmful to the guidance of online public opinion. Notably, prior research often overlooks the existence of opinion extreme agents in social networks. However, existing researches seldom consider the time sunk cost in the evolution of opinions. Building upon this foundation, we introduce a temporal dimension to the opinion evolution, integrating the time sunk cost with the opinion evolution process. Furthermore, we devise an agent partitioning method that categorizes agents into four states based on their opinion values: watch state, subjective state, firm state, and extreme state, with extreme state agents generally expressing radical opinions. We constructed an agent network based on the phenomenon of time sunk costs and proposed a model for the evolution of extreme opinions in this network. Our study found that the information sharing among extreme agents significantly influences the extremization of opinions in various networks. After restricting the exchange of opinions on extreme agents, the number of extreme agents in the network decreased by 40% to 50% compared to the initial situation. Additionally, we also discovered that imposing restrictions on extreme agents in the early stages can help increase the possibility of network opinions moving towards neutral positions. When restriction of extreme agents(REA) was performed at the beginning of the experiment compared to REA in the midway of the experiment, the final number of extreme state agents decreased by 15.57%. The results show that extreme agents have a great influence on the spread and evolution of extreme opinions on platforms.
更多查看译文
AI 理解论文
溯源树
样例
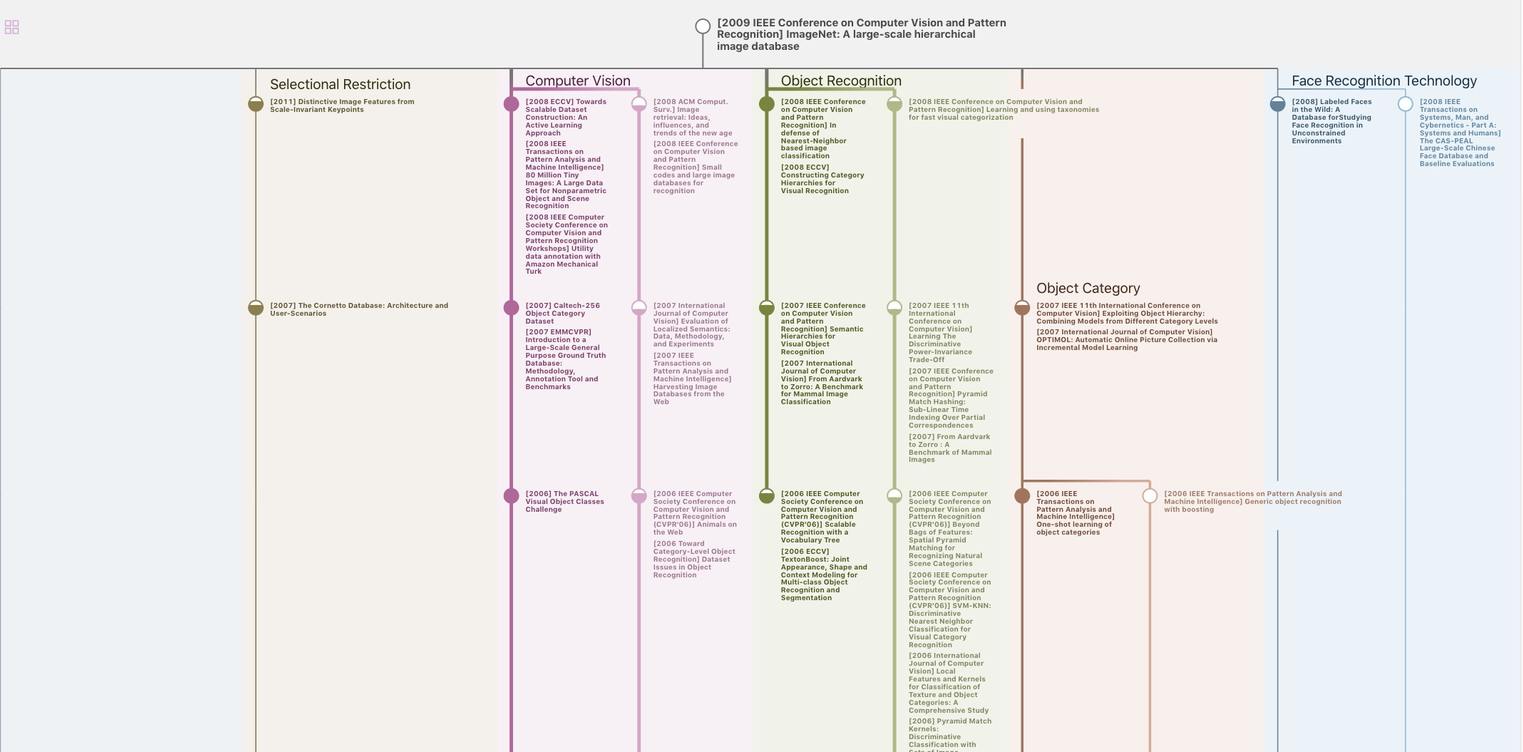
生成溯源树,研究论文发展脉络
Chat Paper
正在生成论文摘要