Prototype Comparison Convolutional Networks for One-Shot Segmentation
IEEE ACCESS(2024)
Abstract
In few-shot semantic segmentation (FSS), the key challenges are efficiently tuning the interaction between the support set and the query set and distinguishing between context, background, and interfering items. To address these challenges, we propose prototype comparison networks for one-shot segmentation (OPCN) to capture the details required for FSS. Specifically, we offer the Fusion Interaction Module (FIM) to improve the segmentation performance by capturing the correlation and semantic information between the support set and query set features. Subsequently, we propose the Feature Enhancement Module (FEM), which aims to enhance the information required in the support set and query set features while increasing the focus on critical details by reducing the weight of the background regions to provide a more targeted feature representation for subsequent query image segmentation. Then, we propose the Feature Refinement Module (FRM) to filter irrelevant background information in the features and specify the target location region. Finally, the Feature Matching Module (FM) generates the final segmentation mask for the query image. Extensive experiments on the PASCAL-5i and COCO-20i datasets show that our approach achieves excellent performance in the case of the one-shot setup.
MoreTranslated text
Key words
Few-shot semantic segmentation,convolutional neural network,prototype network,feature matching
AI Read Science
Must-Reading Tree
Example
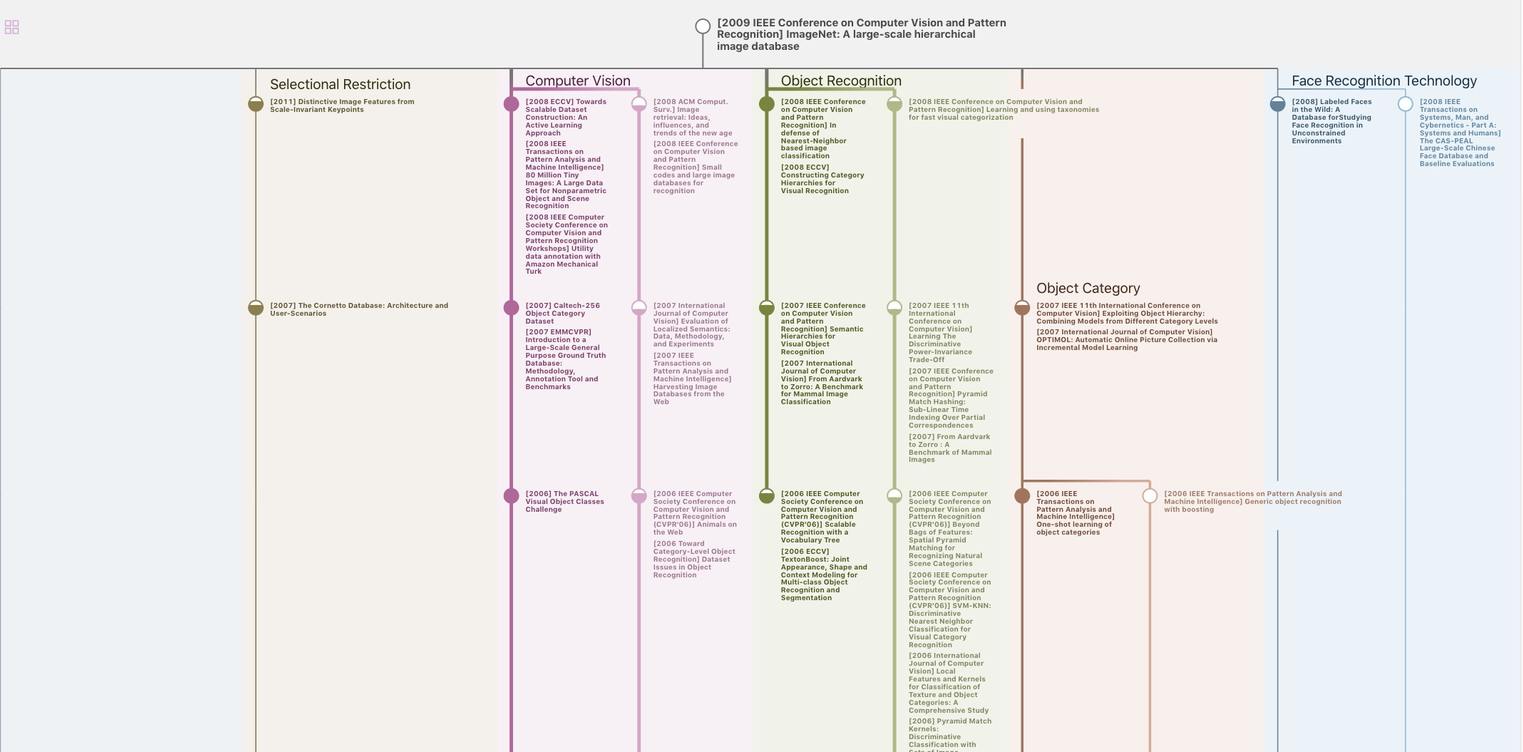
Generate MRT to find the research sequence of this paper
Chat Paper
Summary is being generated by the instructions you defined