Two-Phase Dual-Adversarial Agents With Multivariate Information for Unsupervised Anomaly Detection of IIoT-Edge Devices
IEEE Internet of Things Journal(2024)
摘要
With the improvement of intelligence and integration, automatic supervision of large-scale systems is a current challenge in guaranteeing the high-reliability of edge devices. Hence, fast & accurate anomaly detection (AD) has become an urgent need via the edge computing of the industrial Internet of Things (IIoT). For this purpose, this paper creatively proposes a dual agents based on two-phase adversarial training strategy (2P-DAs) to perform rapid, stable and unsupervised AD for large-scale IIoT-edge devices. It integrates the superiorities of deep autoencoder (AEs) and generative adversarial network (GANs), utilizing normal multivariate time-series as inputs, 1-Encoder vs. 2-Decoders architecture as backbone, and two-phase unsupervised adversarial learning to make it isolate anomalies while providing efficient training. On the one hand, this allows the inherent limitations of AEs to be overcome by training a model capable for recognizing non-anomalies and thus performing a good reconstruction. On the other hand, dual structures allow for stability in adversarial training, thereby solving the issues of collapse and non-convergence encountered in GANs. Two practical industrial data, as cloud & edge data, are used to verify the robustness, inference speed and high detection performance of 2P-DAs in IIoT-edge AD, which demonstrates an impressive performance under multiple evaluation indexes.
更多查看译文
关键词
Anomaly detection,industrial Internet of Things,dual autoencoders,unsupervised adversarial learning,multivariate time-series
AI 理解论文
溯源树
样例
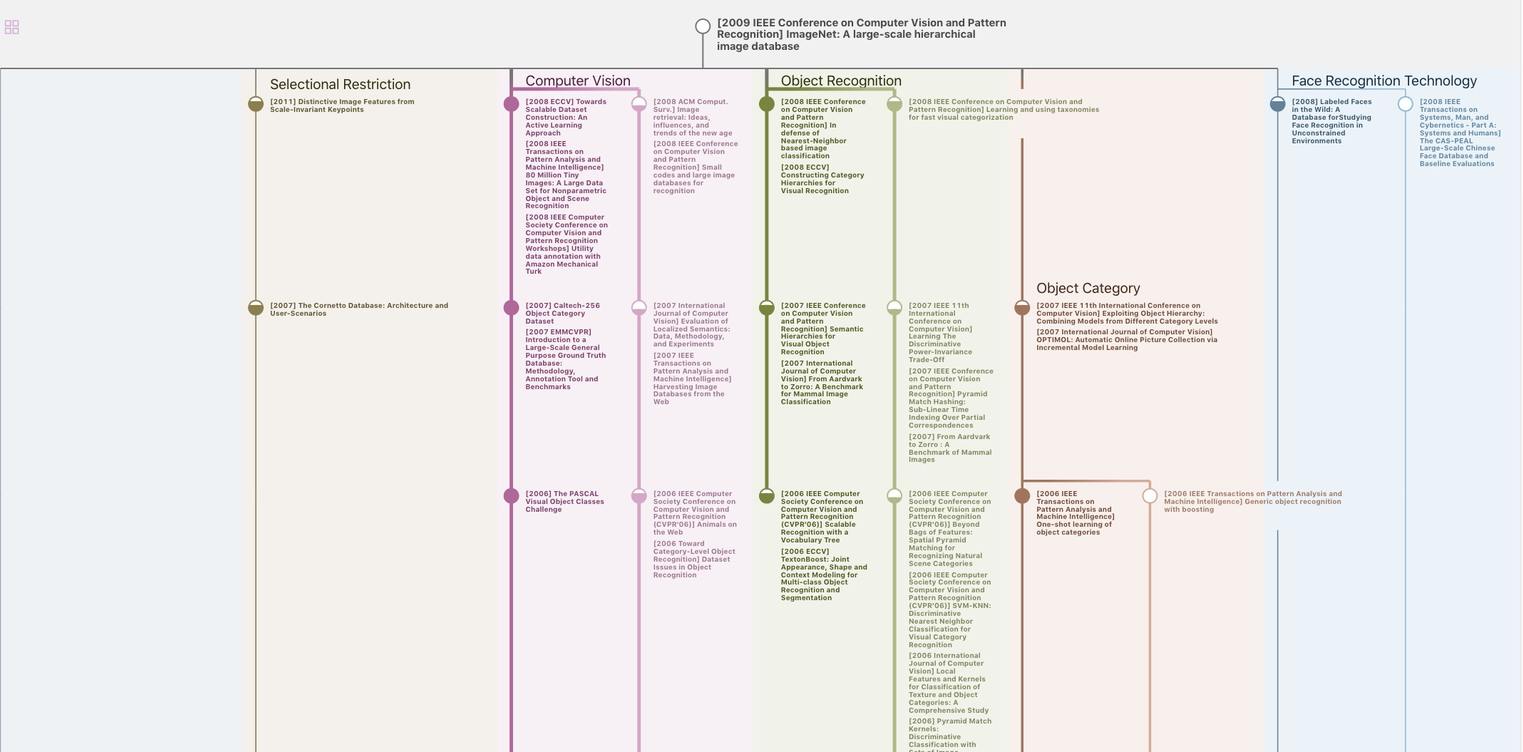
生成溯源树,研究论文发展脉络
Chat Paper
正在生成论文摘要