Towards Privacy-Preserving Waste Classification in the Internet of Things
IEEE Internet of Things Journal(2024)
摘要
Diffuse waste data and associated privacy concerns present significant challenges for effective waste classification in the Internet of Things (IoT) realm. This research introduces a novel approach that leverages differential privacy (DP) and federated transfer learning (FTL) to address the issues, enabling waste classification while preserving privacy within the IoT ecosystem. By integrating Federated Learning (FL), Transfer Learning (TL), and DP, our proposed method facilitates collaborative training while ensuring data privacy. In this methodology, a pre-trained model, initially trained on the ImageNet dataset, is disseminated to IoT devices. Subsequently, these devices perform local training using the TrashNet and Garbage Classification datasets. This process allows devices to capture waste characteristics unique to their individual environments. Through the fusion of general knowledge pertaining to the trained model and local insights, the proposed approach achieves efficient waste classification. The study critically examines the implications for privacy, biases resulting from limited local data, and trade-offs between privacy and model performance. The experimental evaluation demonstrates the effectiveness of the approach and underscores the importance of ensuring privacy-sensitive waste classification. This research contributes to the discourse on FTL and encourages further research into privacy-preserving waste classification within the IoT.
更多查看译文
关键词
Federated Learning,Internet of Things,Privacy Preservation,Transfer Learning,Waste Classification
AI 理解论文
溯源树
样例
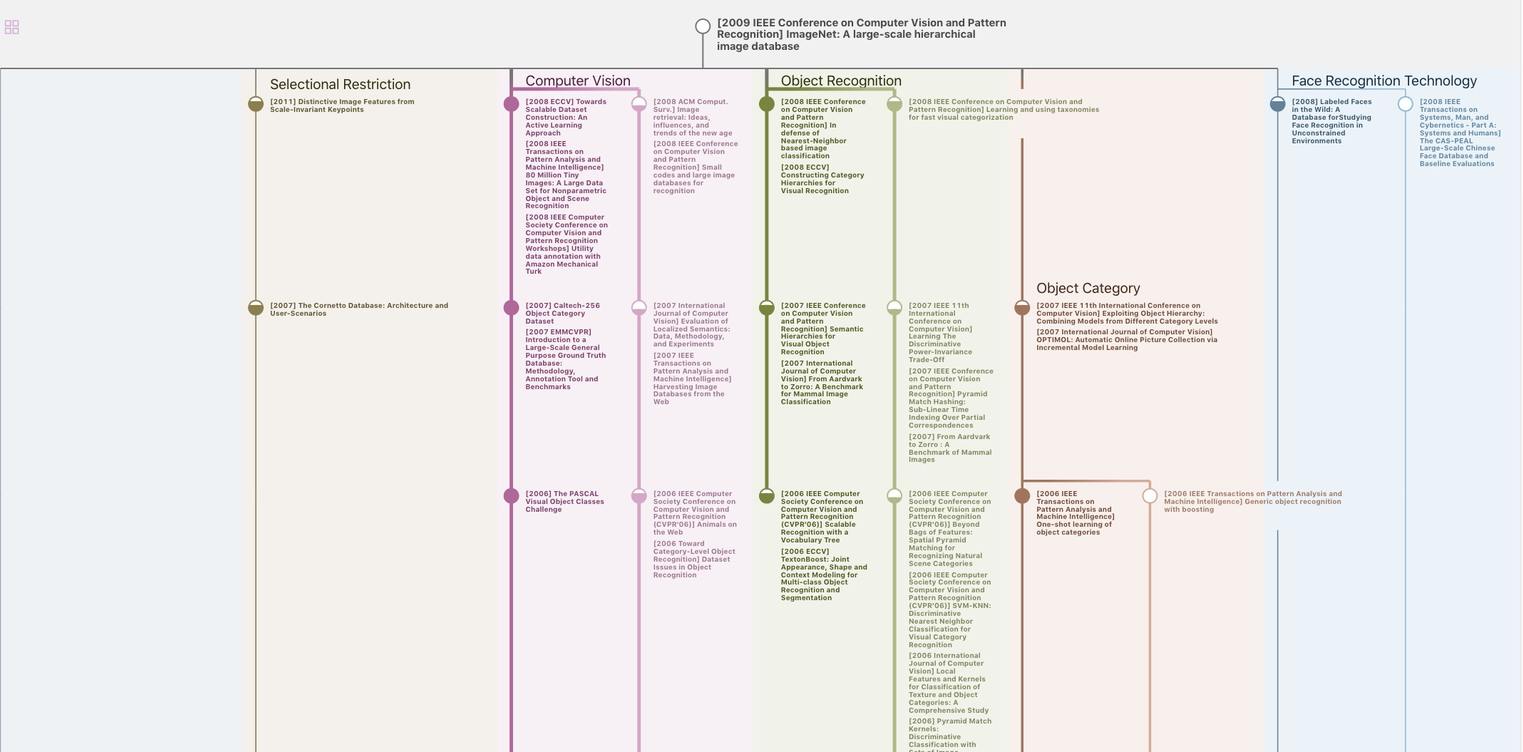
生成溯源树,研究论文发展脉络
Chat Paper
正在生成论文摘要