A Novel Deep Learning Model with Optimized Sparrow Search for Efficient Plant Stress Identification
crossref(2024)
摘要
Abstract In the current economic climate, one of the biggest problems facing the agriculture industry is the accurate and real-timeidentification of biotic and abiotic plant stressors that impact crop yield. The upshot is that illness detection models can’t handlethe pressures of practical, real-time use. By stressing the significance of adapting deep learning models to the intricacies ofdisease-specific datasets, our research presented a fresh approach to solving this critical problem. We provide a novel hybridsparrow search method that can optimise deep convolutional neural network hyperparameters (CNNs). Consequently, themethod for detecting plant stress has become more accurate and precise. An unprecedented leap in model performance isachieved by DeepHSSA, which merges the exploratory dynamics of Sparrow Search with the adaptive procedures of Searchand Rescue Optimization. The robustness of DeepHSSA was evaluated in response to dynamic, real-time field circumstancesacross diverse agricultural landscapes in India using a comprehensive dataset consisting of seven abiotic stress indicators andseven biotic stress indicators. In comparison to more conventional models, DeepHSSA achieved an impressive accuracy rateof 99.94 percent.
更多查看译文
AI 理解论文
溯源树
样例
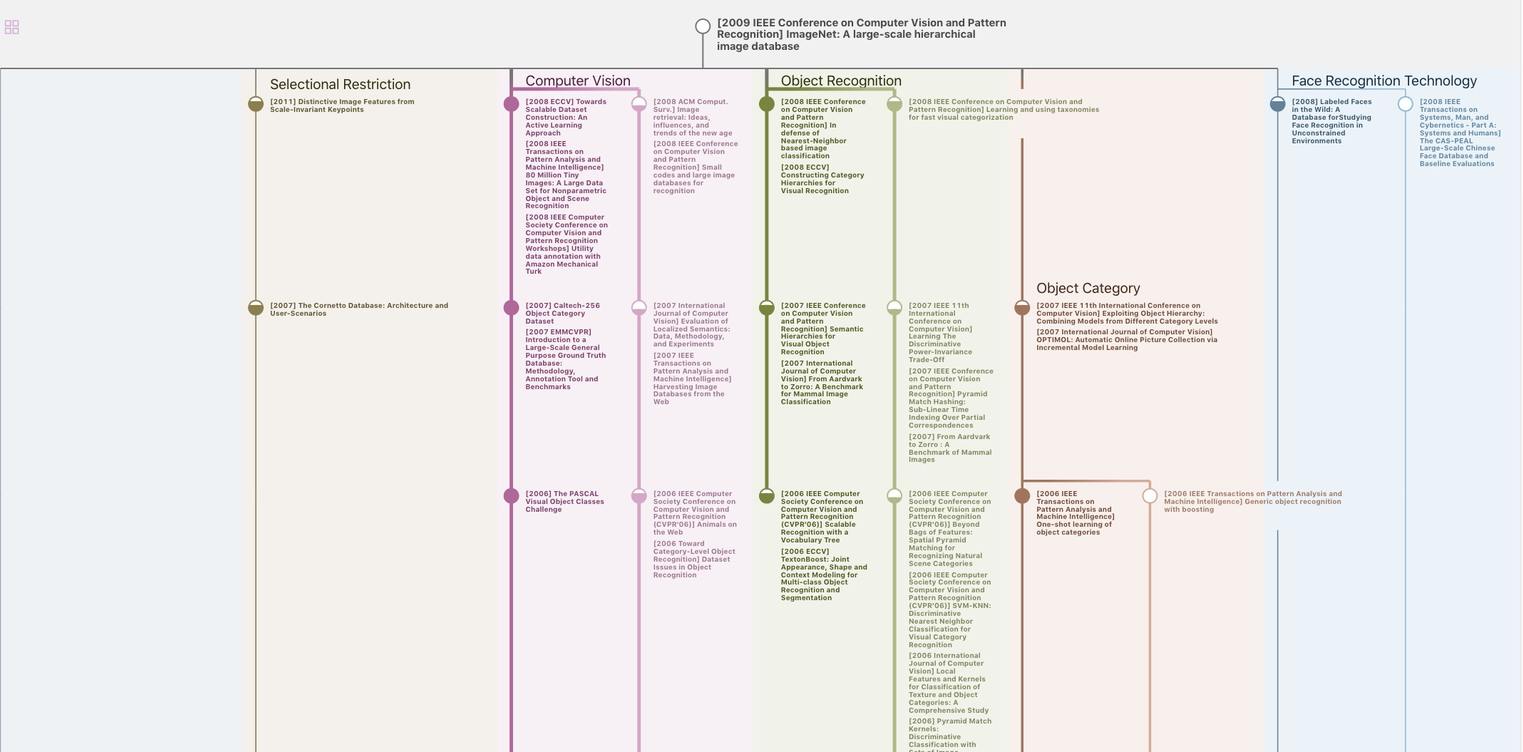
生成溯源树,研究论文发展脉络
Chat Paper
正在生成论文摘要