Advancing blast fragmentation simulation of RC slabs: A graph neural network approach
Engineering Structures(2024)
摘要
Accurate prediction of blast-induced fragmentation in reinforced concrete (RC) structures is pivotal for structural debris hazard assessment in an explosion event. This assessment is essential for designing effective measures for protection of people and structure in the vicinity. While conventional computational techniques are proficient, they are computationally very demanding and may not be readily adaptable in practical applications. This study proposes a novel approach, the Fragment Graph Network (FGN), a specialized variant of graph neural networks (GNNs), to simulate complex interactions between macro-level particles of RC structures subjected to close-in blast loads. The RC structure is discretised into particles, with the model predicting structural responses and fragmentation based on states of these particles. Utilizing an autoregressive prediction mechanism, our model can accurately simulate spatiotemporal responses of RC slabs against blast loads. The efficacy of the model was tested on a dataset consisting of 149 simulated cases, encompassing around 180,000 states each, covering a wide range of blasting scenarios and structural design parameters including TNT charge weight, standoff distance, slab dimension, concrete strength, and reinforcement ratio and layout. Notably, the proposed model delivered accurate predictions, achieving relative errors under 10% and R2 values exceeding 0.93 for both standard and extrapolated test scenarios as compared to the SPH simulations. Remarkably, our model dramatically outpaced traditional numerical approaches, reducing simulation times from hours to minutes on CPUs and seconds on GPUs. This pioneering effort paves the way for efficient and accurate simulations of RC blast fragmentations, offering a rapid alternative to standard numerical approaches.
更多查看译文
关键词
Fragmentation,RC slabs,Blast loads,Machine learning,Graph neural networks
AI 理解论文
溯源树
样例
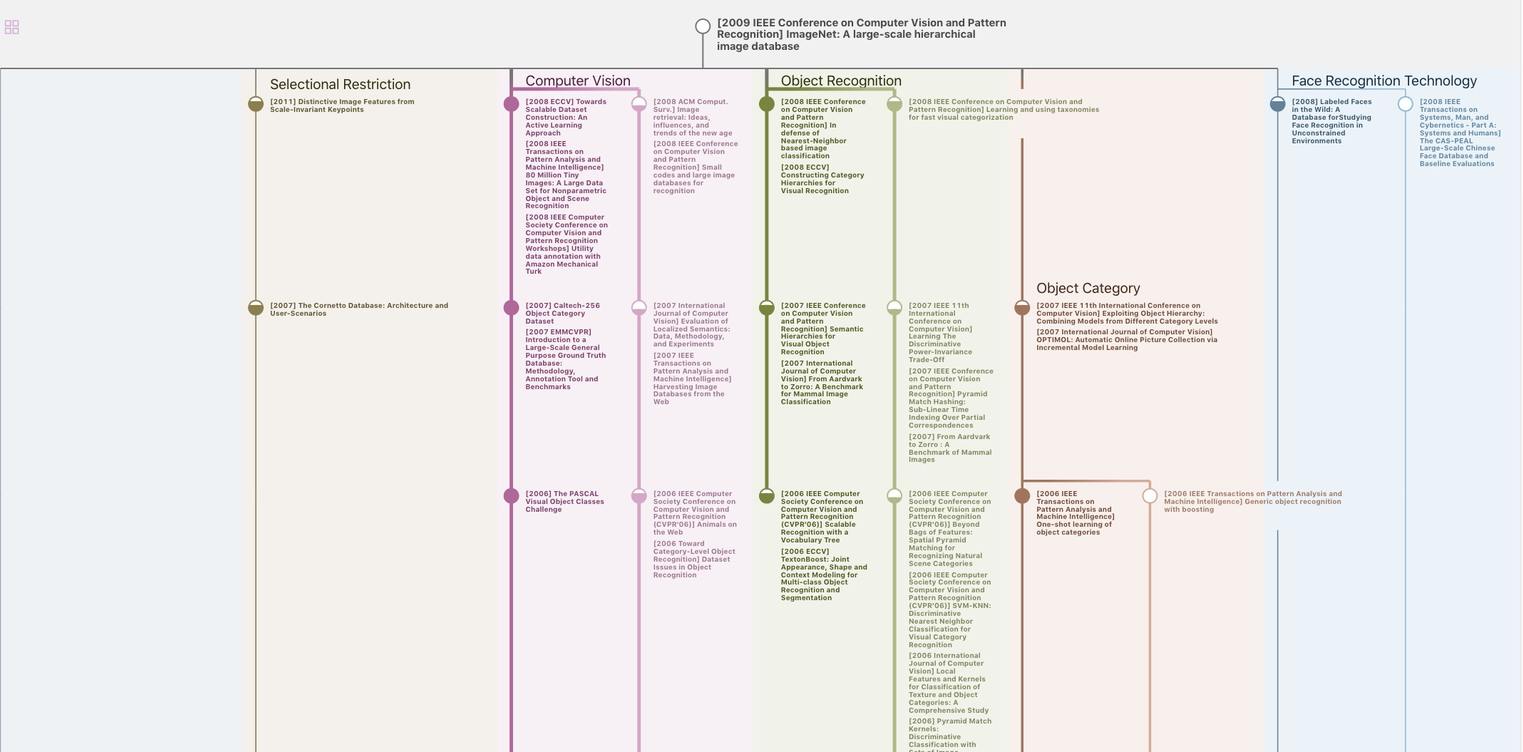
生成溯源树,研究论文发展脉络
Chat Paper
正在生成论文摘要