Unifying Obstacle Avoidance and Tracking Control of Redundant Manipulators Subject to Joint Constraints: A New Data-Driven Scheme
IEEE Transactions on Cognitive and Developmental Systems(2024)
摘要
In modern manufacturing, redundant manipulators have been widely deployed. Performing a task often requires the manipulator to follow specific trajectories while avoiding surrounding obstacles. Different from most existing obstacle-avoidance schemes that rely on the kinematic model of redundant manipulators, in this paper, we propose a new data-driven obstacle-avoidance (DDOA) scheme for the collision-free tracking control of redundant manipulators. The obstacle-avoidance task is formulated as a quadratic programming problem with inequality constraints. Then, the objectives of obstacle avoidance and tracking control are unitedly transformed into a computation problem of solving a system including three recurrent neural networks. With the Jacobian estimators designed based on zeroing neural networks, the manipulator Jacobian and critical-point Jacobian can be estimated in a data-driven way without knowing the kinematic model. Finally, the effectiveness of the proposed scheme is validated through extensive simulations and experiments.
更多查看译文
关键词
Data-driven control,obstacle avoidance,redundant manipulator,zeroing neural network
AI 理解论文
溯源树
样例
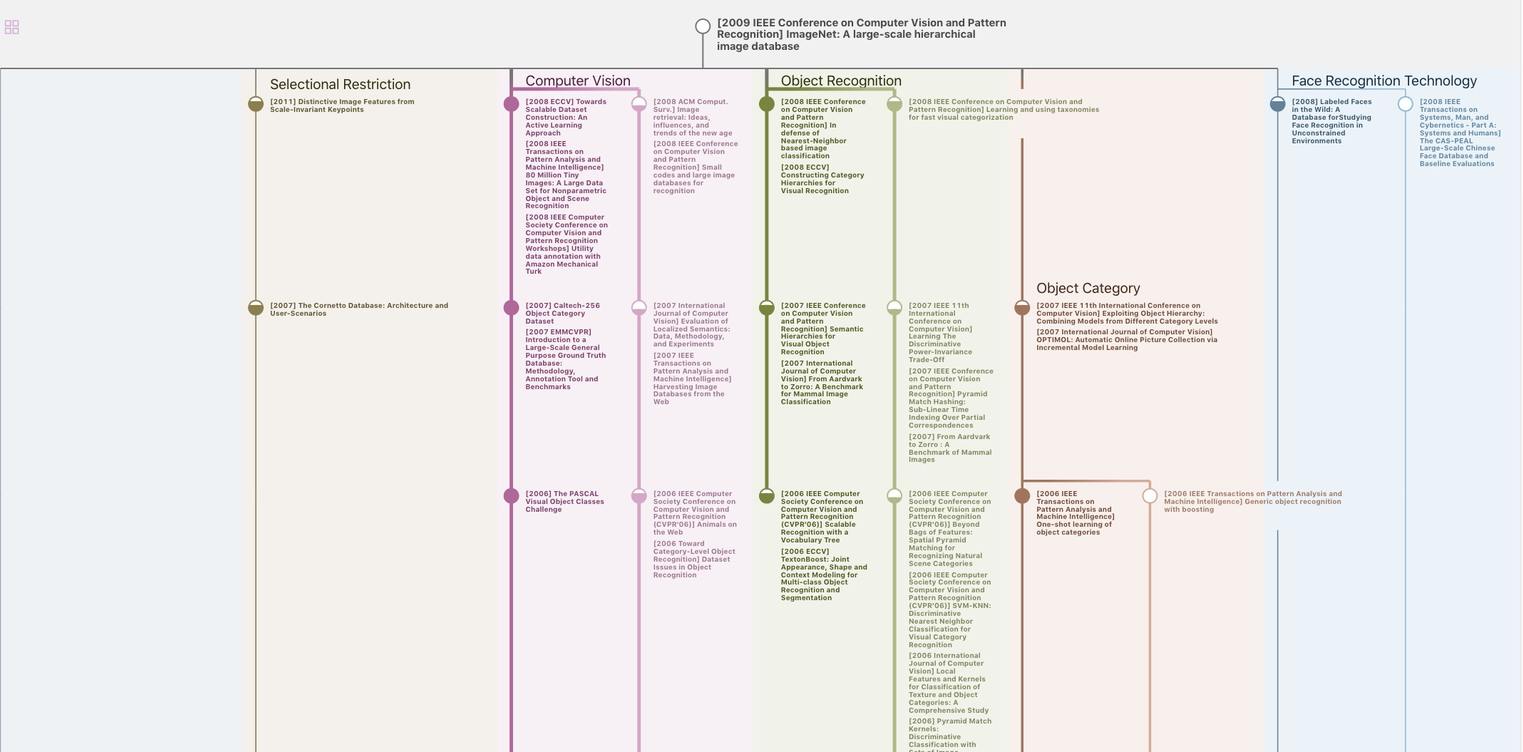
生成溯源树,研究论文发展脉络
Chat Paper
正在生成论文摘要