CMNet: Component-Aware Matching Network for Few-Shot Point Cloud Classification
IEEE Transactions on Multimedia(2024)
摘要
Few-shot point cloud classification is currently an under-explored problem which aims to learn a point cloud classifier for novel categories given a few annotated training data. Most existing methods achieve classification by matching a query point cloud to the most similar support category at the global representation level. However, due to the complicated structure of the point cloud and scarce available training data, the global representations of the point clouds and categories are of low quality, limiting the matching accuracy. Therefore, in this paper, we propose the Component-Aware Matching Network (CMNet) that matches the point clouds at the component level in addition to the global level. Specifically, we construct a component set for each query point cloud and support category and develop a metric to measure the similarity between two component sets. The final prediction is the weighted sum of the global and component matching probabilities. Besides, we carefully devise a component matching pretraining scheme for CMNet to enhance its ability to extract component features, further improving its performance. To evaluate the effectiveness of our design, we conduct comprehensive experiments on three benchmarks, namely ModelNet-FS, ShapeNet-FS and Shrec-FS. As a result, CMNet consistently outperforms the existing methods with significant margins in all the experiments of the three benchmarks and sets new state-of-the-art performance.
更多查看译文
关键词
few-shot,metric learning,meta learning,point cloud classification,component matching
AI 理解论文
溯源树
样例
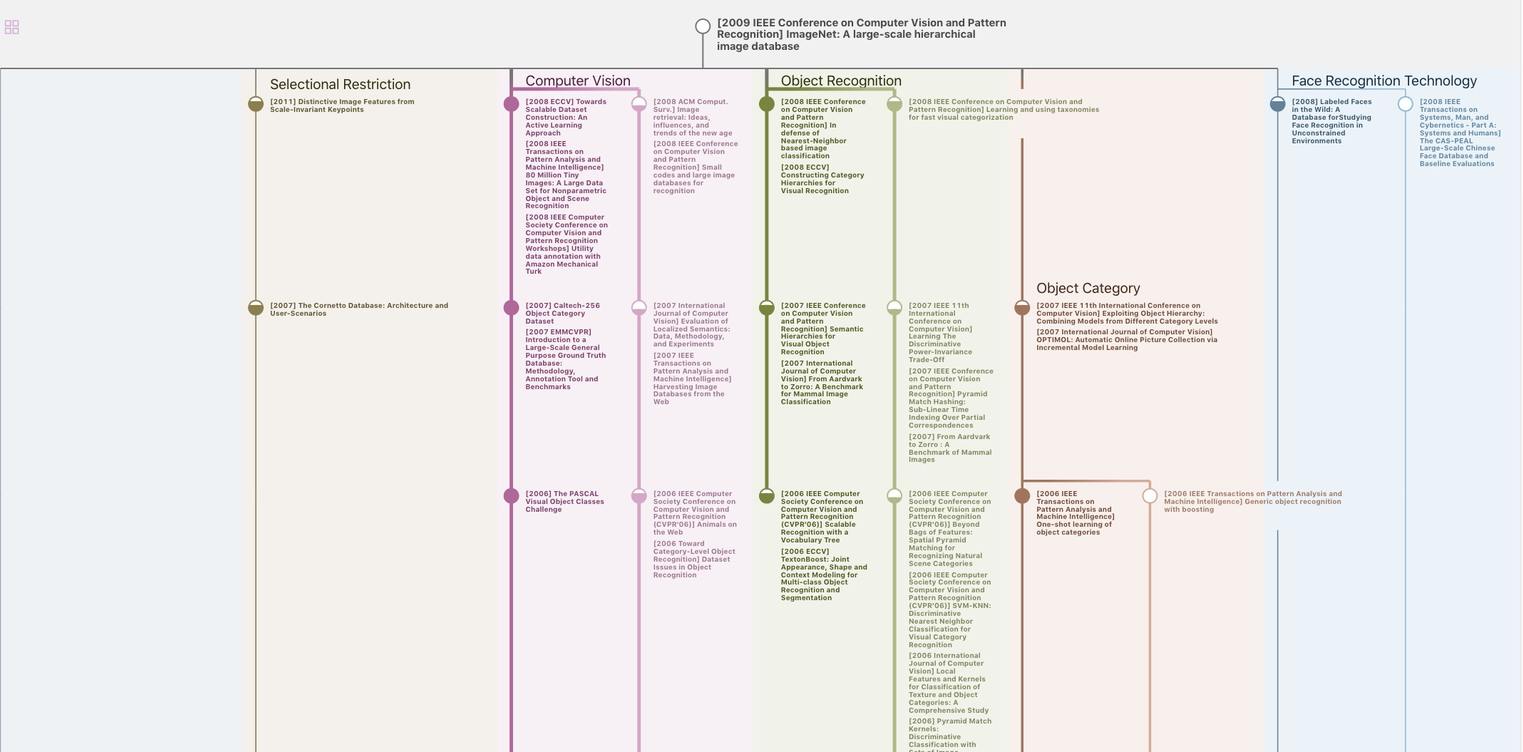
生成溯源树,研究论文发展脉络
Chat Paper
正在生成论文摘要