Noise Level and Blind Quality Evaluations in Abdominopelvic Electromagnetic X-ray Computed Tomographic Images using Deep-learning Reconstruction Method
JOURNAL OF MAGNETICS(2024)
摘要
This study aimed to confirm the characteristics of deep-learning image reconstruction (DLIR) intensity in abdominopelvic computed tomography (CT) using noise level and blind quality evaluation parameters. The study was conducted using phantoms and patients, and CT images were obtained while adjusting the intensity of DLIR to low (DLIR-L), middle (DLIR-M), and high (DLIR-H). To quantitatively evaluate image quality, the coefficient of variation (COV) and contrast-to-noise ratio (CNR), as well as natural image quality evaluation (NIQE) and blind/referenceless image spatial quality evaluator (BRISQUE), were used. In both the noise level and blind quality evaluation results, a higher strength of DLIR resulted in better results derived from the phantom and patient studies. In particular, the results of the phantom study confirmed that NIQE and BRISQUE of CT images acquired using DLIR-H were improved by approximately 5 % and 1 %, respectively, compared to the corresponding the application of DLIR-L. Moreover, when high-strength deep-learning was applied to a real patient's CT image reconstruction method, the NIQE and BRISQUE results improved by approximately 6 % and 4 %, respectively, compared with their respective medium levels. In conclusion, we quantitatively analyzed the image quality according to the intensity of the recently developed deep-learning-based CT image reconstruction method.
更多查看译文
关键词
Abdominopelvic computed tomography (CT),Deep-learning image reconstruction (DLIR) intensity,Noise level evaluation,Blind image quality evaluation
AI 理解论文
溯源树
样例
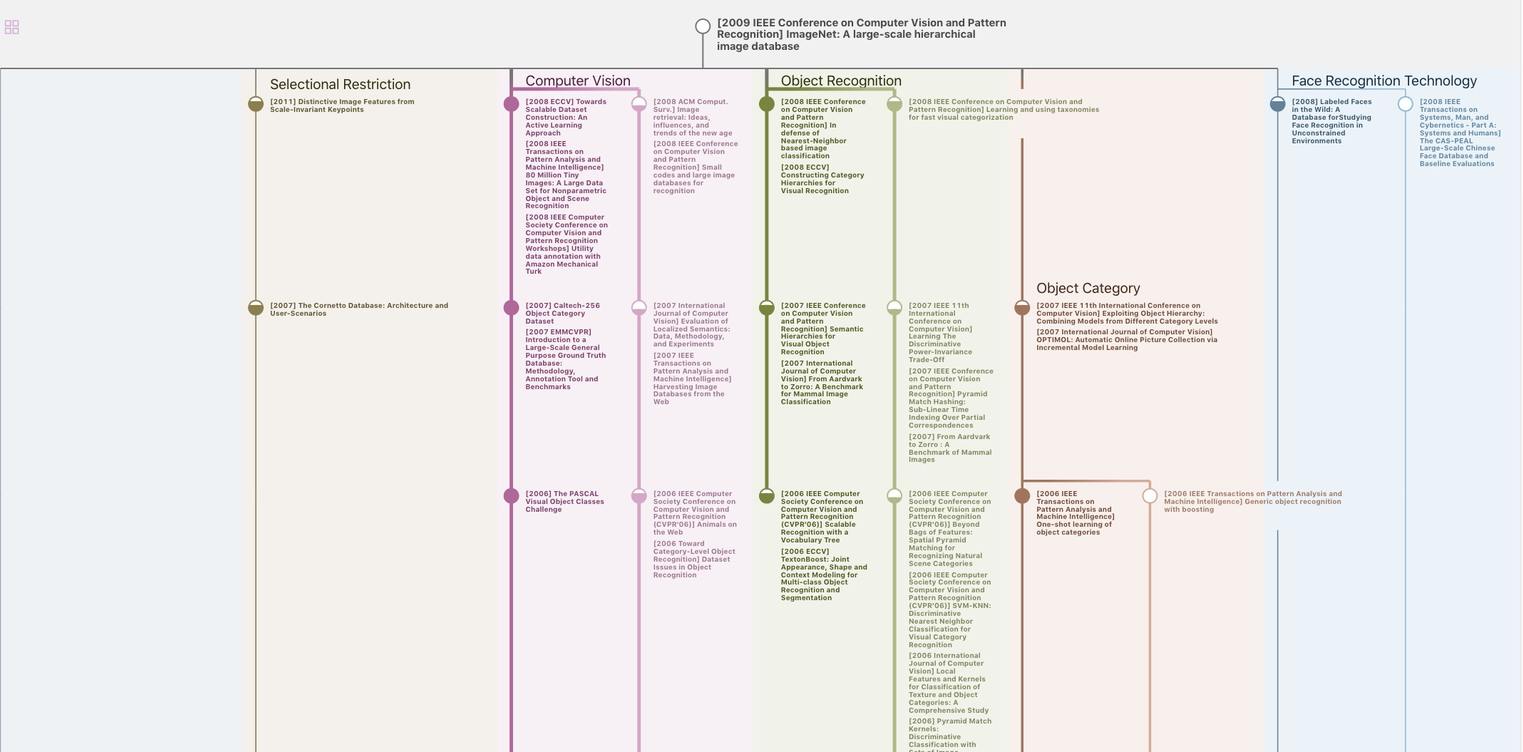
生成溯源树,研究论文发展脉络
Chat Paper
正在生成论文摘要