Bearing fault diagnosis based on transfer learning with dual-flow manifold ResNet and improved CapsNet
MEASUREMENT SCIENCE AND TECHNOLOGY(2024)
摘要
In intelligent fault diagnosis for rolling bearings, the variable operating conditions of rotating machinery lead to inconsistent distributions between training and testing data, inevitably decreasing fault diagnosis accuracy. To overcome this issue, we propose a bearing fault diagnosis method based on transfer learning with a dual-flow manifold residual network and improved capsule network (DMRCN). Firstly, we introduce a dual-flow manifold residual network structure consisting of continuous wide-kernel convolution, continuous narrow-kernel convolution, and manifold learning spatial information description blocks. This architecture is utilized to extract low-level features from the data. Then, we present a novel architecture of the capsule network with self-attention output. We incorporate a self-attention mechanism into the dynamic routing algorithm to transfer richer high-level features from the low-level capsule layer to the higher-level capsule layer. During training, we propose a loss function known as global-local distribution discrepancy loss to enhance the model's generalization ability and accelerate model convergence. Finally, we validate the performance of DMRCN in cross-condition transfer fault diagnosis on both the Case Western Reserve University public dataset and a laboratory-built bearing experimental rig dataset. Experimental results demonstrate that DMRCN outperforms other commonly used deep transfer learning methods regarding fault diagnosis across different operating conditions.
更多查看译文
关键词
transfer learning,bearing intelligent fault diagnosis,manifold residual network,capsule network,global-local distribution discrepancy loss
AI 理解论文
溯源树
样例
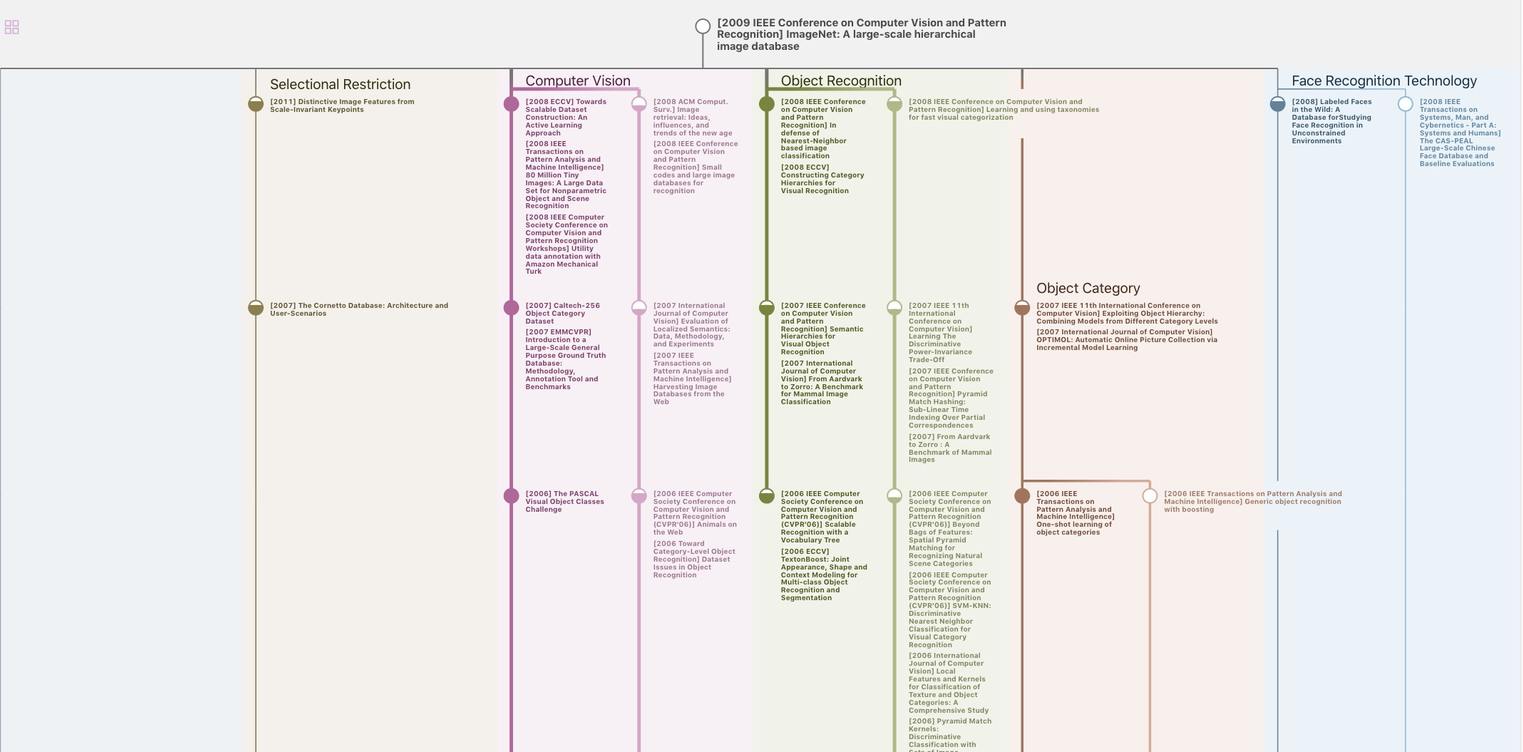
生成溯源树,研究论文发展脉络
Chat Paper
正在生成论文摘要