FARFusion: A Practical Roadside Radar-Camera Fusion System for Far-Range Perception
IEEE Robotics and Automation Letters(2024)
摘要
Far-range perception through roadside sensors is crucial to the effectiveness of intelligent transportation systems. The main challenge of far-range perception is due to the difficulty of performing accurate object detection and tracking under far distances
(e.g., > 150m)
at a low cost. To cope with such challenges, deploying both millimeter wave Radars and high-definition (HD) cameras, and fusing their data for joint perception has become a common practice. The key to this solution, however, is the precise association between the two types of data, which are captured from different perspectives and have different degrees of measurement noises. Towards this goal, the first question is which plane to conduct the association, i.e., the 2D image plane or the BEV plane. We argue that the former is more suitable because the magnitude of location errors in the perspective projection points is smaller at far distances on the 2D plane and can lead to more accurate association. Thus, we first project the Radar-based target locations (on the BEV plane) to the 2D plane and then associate them with the camera-based object locations that are modeled as a point on each object. Subsequently, we map the camera-based object locations to the BEV plane through inverse projection mapping (IPM) with the corresponding depth information from the Radar data. Finally, we engage a BEV tracking module to generate target trajectories for traffic monitoring. Since our approach involves transformation between the 2D plane and BEV plane, we also devise a transformation parameters refining approach based on a depth scaling technique, utilising the above fusion process without requiring any additional devices such as GPS. We have deployed an actual testbed on an urban expressway and conducted extensive experiments to evaluate the effectiveness of our system. The results show that our system can improve AP
BEV
by 32%, and reduce the location error by
0.56m
. Our system is capable of achieving an average location accuracy of
1.3m
when we extend the detection range up to
500m
. We thus believe that our proposed method offers a viable approach to efficient roadside far-range perception.
更多查看译文
关键词
Sensor fusion,object detection,calibration and identification
AI 理解论文
溯源树
样例
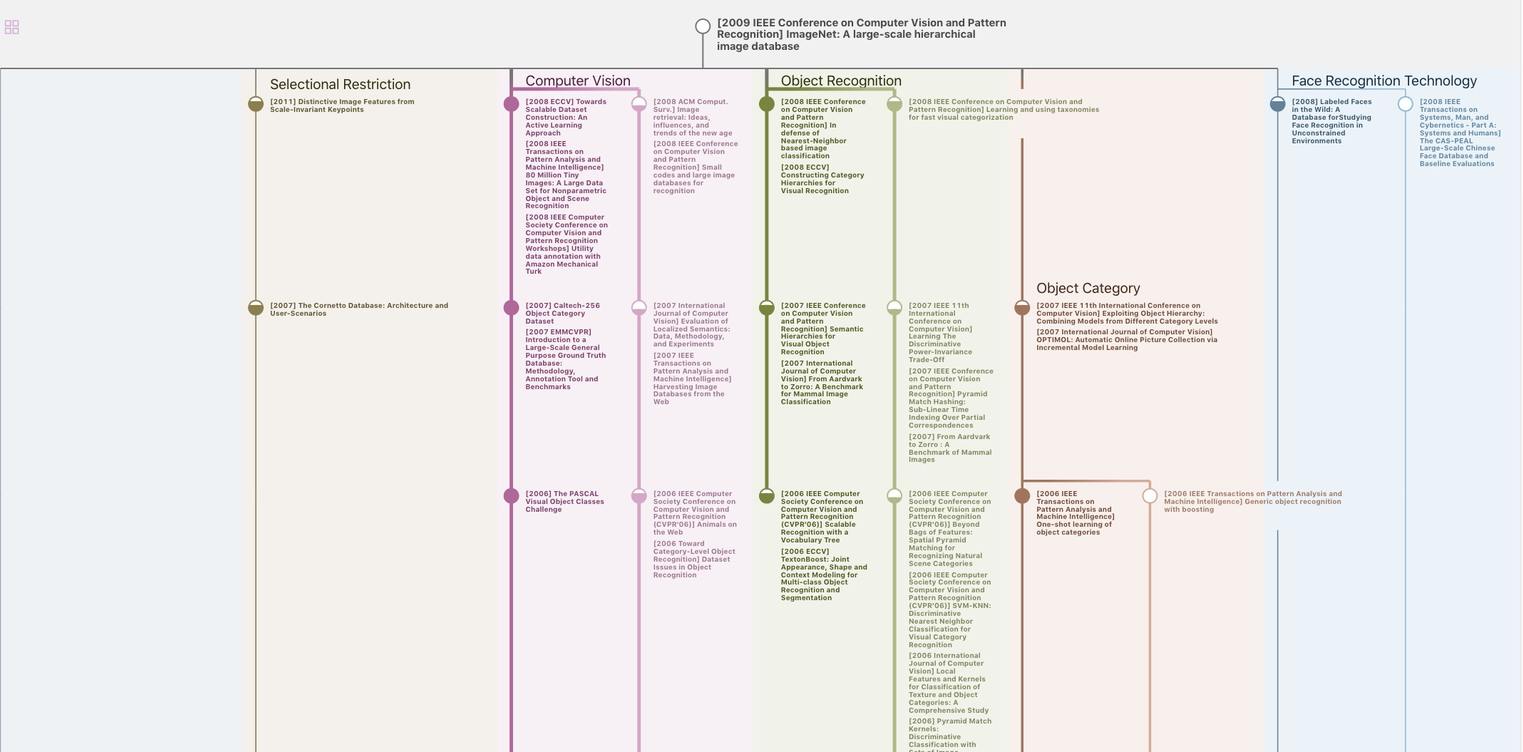
生成溯源树,研究论文发展脉络
Chat Paper
正在生成论文摘要