The science behind the nose: correlating volatile organic compound characterisation with canine biodetection of COVID-19.
ERJ open research(2024)
摘要
Background:The SARS-CoV-2 pandemic stimulated the advancement and research in the field of canine scent detection of COVID-19 and volatile organic compound (VOC) breath sampling. It remains unclear which VOCs are associated with positive canine alerts. This study aimed to confirm that the training aids used for COVID-19 canine scent detection were indeed releasing discriminant COVID-19 VOCs detectable and identifiable by gas chromatography (GC-MS).
Methods:Inexperienced dogs (two Labradors and one English Springer Spaniel) were trained over 19 weeks to discriminate between COVID-19 infected and uninfected individuals and then independently validated. Getxent tubes, impregnated with the odours from clinical gargle samples, used during the canines' maintenance training process were also analysed using GC-MS.
Results:Three dogs were successfully trained to detect COVID-19. A principal components analysis model was created and confirmed the ability to discriminate between VOCs from positive and negative COVID-19 Getxent tubes with a sensitivity of 78% and a specificity of 77%. Two VOCs were found to be very predictive of positive COVID-19 cases. When comparing the dogs with GC-MS, F1 and Matthew's correlation coefficient, correlation scores of 0.69 and 0.37 were observed, respectively, demonstrating good concordance between the two methods.
Interpretation:This study provides analytical confirmation that canine training aids can be safely and reliably produced with good discrimination between positive samples and negative controls. It is also a further step towards better understanding of canine odour discrimination of COVID-19 as the scent of interest and defining what VOC elements the canines interpret as "essential".
更多查看译文
AI 理解论文
溯源树
样例
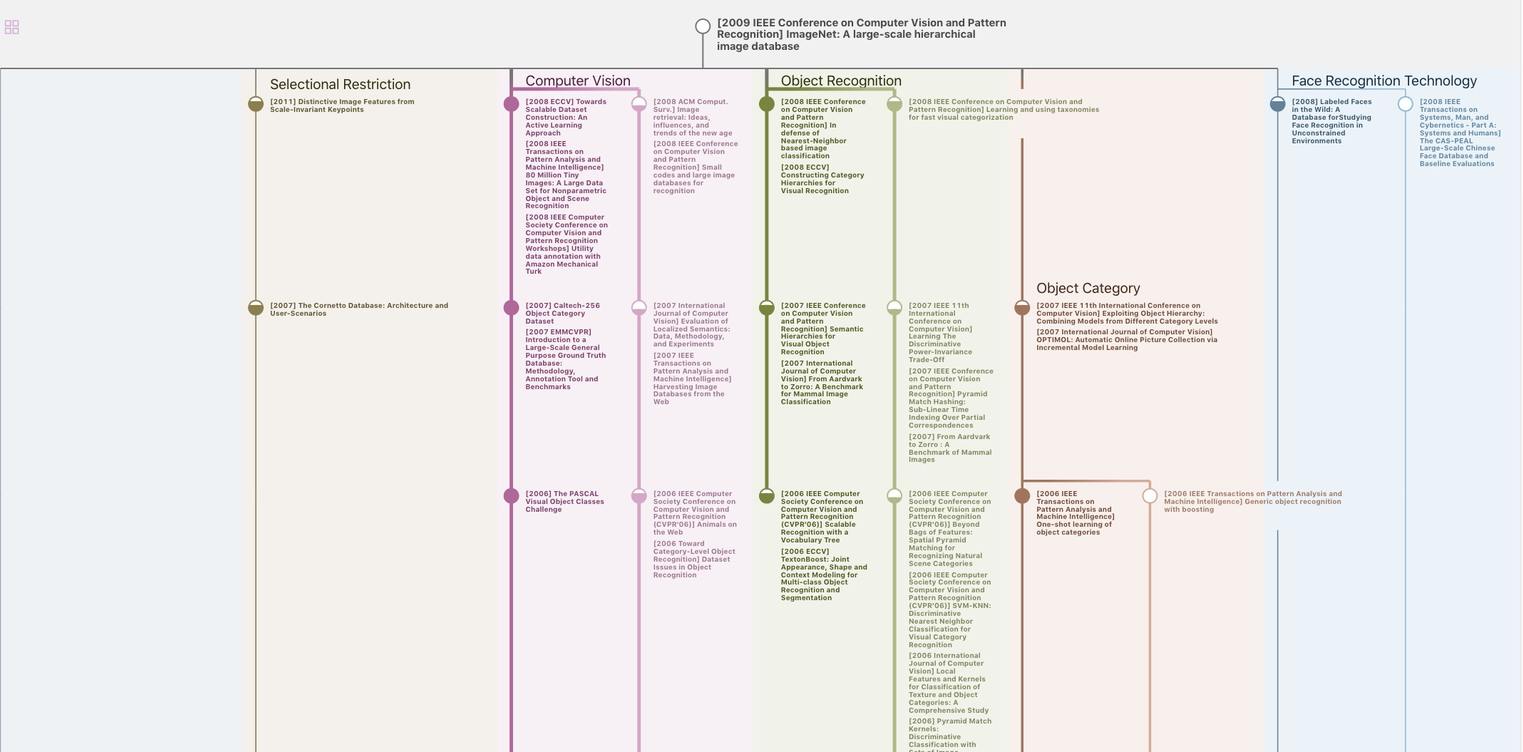
生成溯源树,研究论文发展脉络
Chat Paper
正在生成论文摘要