M-scan: A Multi-Scenario Causal-driven Adaptive Network for Recommendation
WWW 2024(2024)
关键词
Context-Aware Recommender Systems,Content-Based Recommendation,Trust-Aware Recommender Systems,User Modeling,Click-Through Rate Prediction
AI 理解论文
溯源树
样例
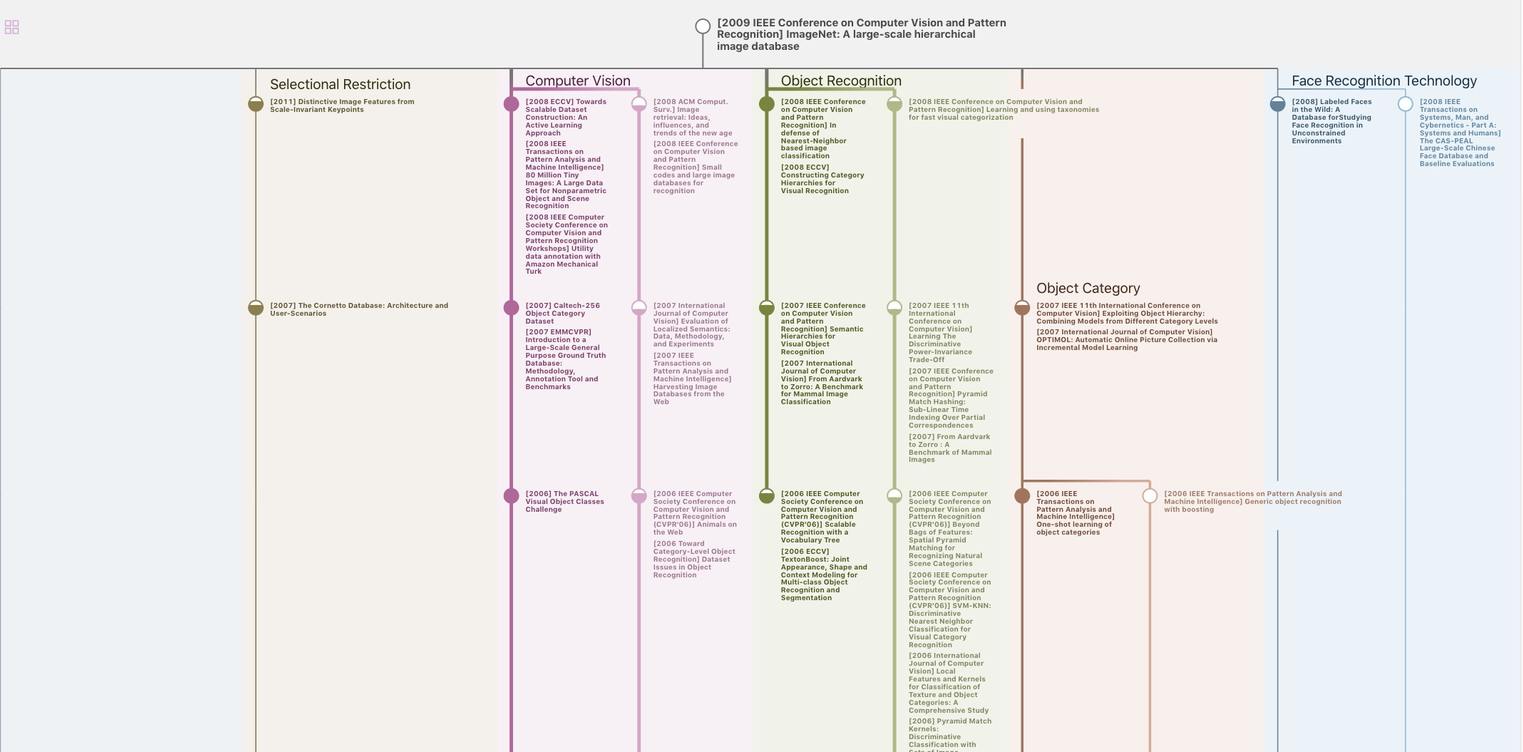
生成溯源树,研究论文发展脉络
Chat Paper
正在生成论文摘要