Does In-Context Learning Really Learn? Rethinking How Large Language Models Respond and Solve Tasks via In-Context Learning
arxiv(2024)
Abstract
In-context Learning (ICL) has emerged as a powerful capability alongside the
development of scaled-up large language models (LLMs). By instructing LLMs
using few-shot demonstrative examples, ICL enables them to perform a wide range
of tasks without updating millions of parameters. However, the precise
contributions of demonstrations towards improving end-task performance have not
been thoroughly investigated in recent analytical studies. In this paper, we
empirically decompose the overall performance of ICL into three dimensions,
label space, format, and discrimination, and we evaluate four general-purpose
LLMs across a diverse range of tasks. Counter-intuitively, we find that the
demonstrations have a marginal impact on provoking discriminative knowledge of
language models. However, ICL exhibits significant efficacy in regulating the
label space and format, which helps LLMs respond to desired label words. We
then demonstrate that this ability functions similar to detailed instructions
for LLMs to follow. We additionally provide an in-depth analysis of the
mechanism of retrieval helping with ICL. Our findings demonstrate that
retrieving the semantically similar examples notably boosts the model's
discriminative capability. However, we also observe a trade-off in selecting
good in-context examples regarding label diversity.
MoreTranslated text
AI Read Science
Must-Reading Tree
Example
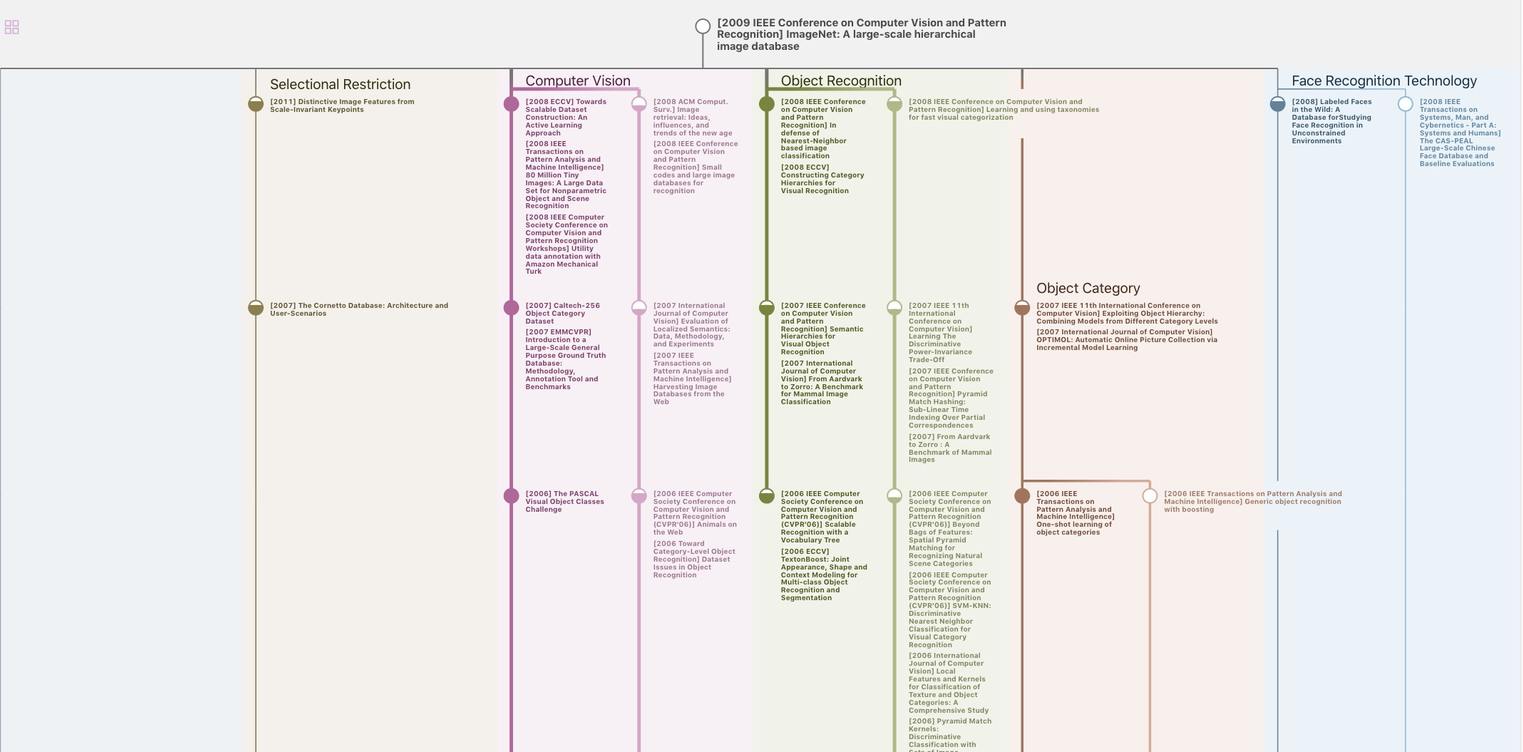
Generate MRT to find the research sequence of this paper
Chat Paper
Summary is being generated by the instructions you defined