Decentralized Landslide Disaster Prediction for Imbalanced and Distributed Data.
Annual IEEE International Conference on Pervasive Computing and Communications(2024)
摘要
Precise disaster prediction plays a critical role in saving lives. Traditional landslide prediction methods have predominantly relied on deep neural networks such as CNNs and LSTMs. However, these methods face two main challenges. The first is the class imbalance issue, as landslides are rare, disrupting the training process. The second challenge stems from decentralized data management, with variations in volume and characteristics across regions. Typically, local municipalities manage disaster data within their regions, and sharing or migrating this data is not straightforward. This paper presents SlideSafe: a novel landslide prediction system that combines spatio-temporal contrastive learning and collaborative learning
1
1
Our implementation is available here (https://github.com/mclab-osaka/slidesafe). It begins by training a contrastive learning model to extract meaningful representations of land characteristics in each region. Subsequently, these trained models are merged among regions with similar characteristics, leveraging federated learning. The federated models are then fine-tuned and customized for the landslide event prediction using the data specific to each region. Experimental results indicate that the proposed system achieves higher precision under 100% recall compared to state-of-the-art federated learning methods, which are often adversely affected by non-iid data and data scarcity.
更多查看译文
关键词
Decentralized Landslide Prediction,Class imbalance,Contrastive Learning,Selective Federated Learning
AI 理解论文
溯源树
样例
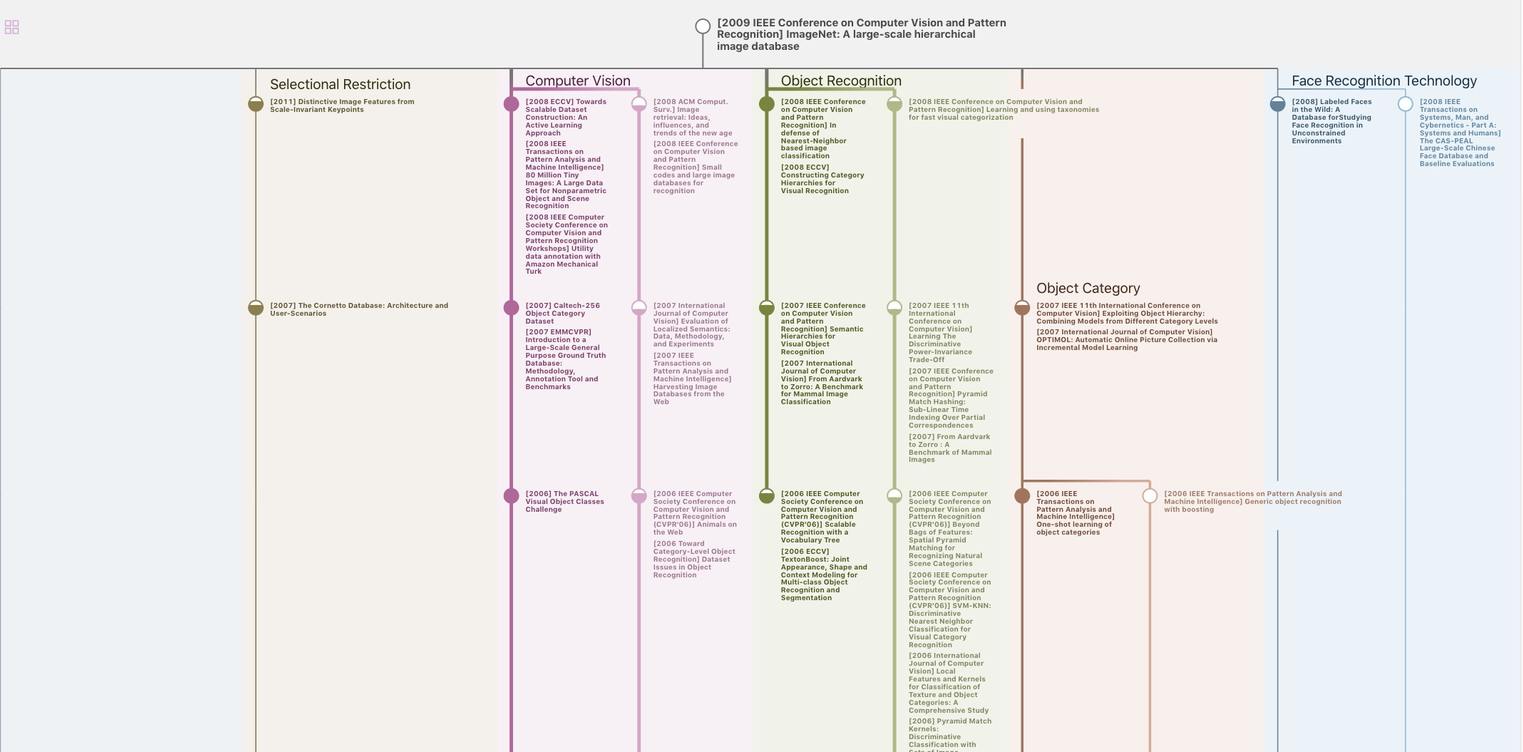
生成溯源树,研究论文发展脉络
Chat Paper
正在生成论文摘要