Hyperspectral imaging predicts free fatty acid levels, peroxide values, and linoleic acid and oleic acid concentrations in tree nut kernels
LWT(2024)
Abstract
Imaging technologies are advancing rapidly in food processing systems to reduce food waste. However, modelling techniques, sample sizes and dataset proportioning methods significantly affect the performance of models in predicting food quality variables. This study examined the potential of hyperspectral imaging to predict peroxide values (PV), free fatty acid (FFA) levels and fatty acid concentrations, including oleic and linoleic acid, in two tree nuts, canarium and macadamia. The effectiveness of artificial neural network (ANN) regression and partial least squares regression (PLSR) techniques to make these predictions was examined. Additionally, the importance of the dataset size on prediction accuracy and the dataset-proportioning method for developing predictive models were assessed. Both ANN and PLSR models predicted FFA levels and oleic acid concentrations with high accuracy, but PV and linoleic acid concentrations were predicted poorly. Changing the test-dataset proportioning method for the small dataset led to comparable R2test values by both ANN and PLSR in predicting FFA levels. Successful prediction of FFA levels could be explained partly by high variability, even in the dataset with the small number of samples. The study highlights the significance of factors like dataset size, test-dataset proportioning method, and model selection when predicting the quality attributes.
MoreTranslated text
Key words
Canarium indicum,Hyperspectral,Macadamia integrifolia,Non-destructive,Nuts
AI Read Science
Must-Reading Tree
Example
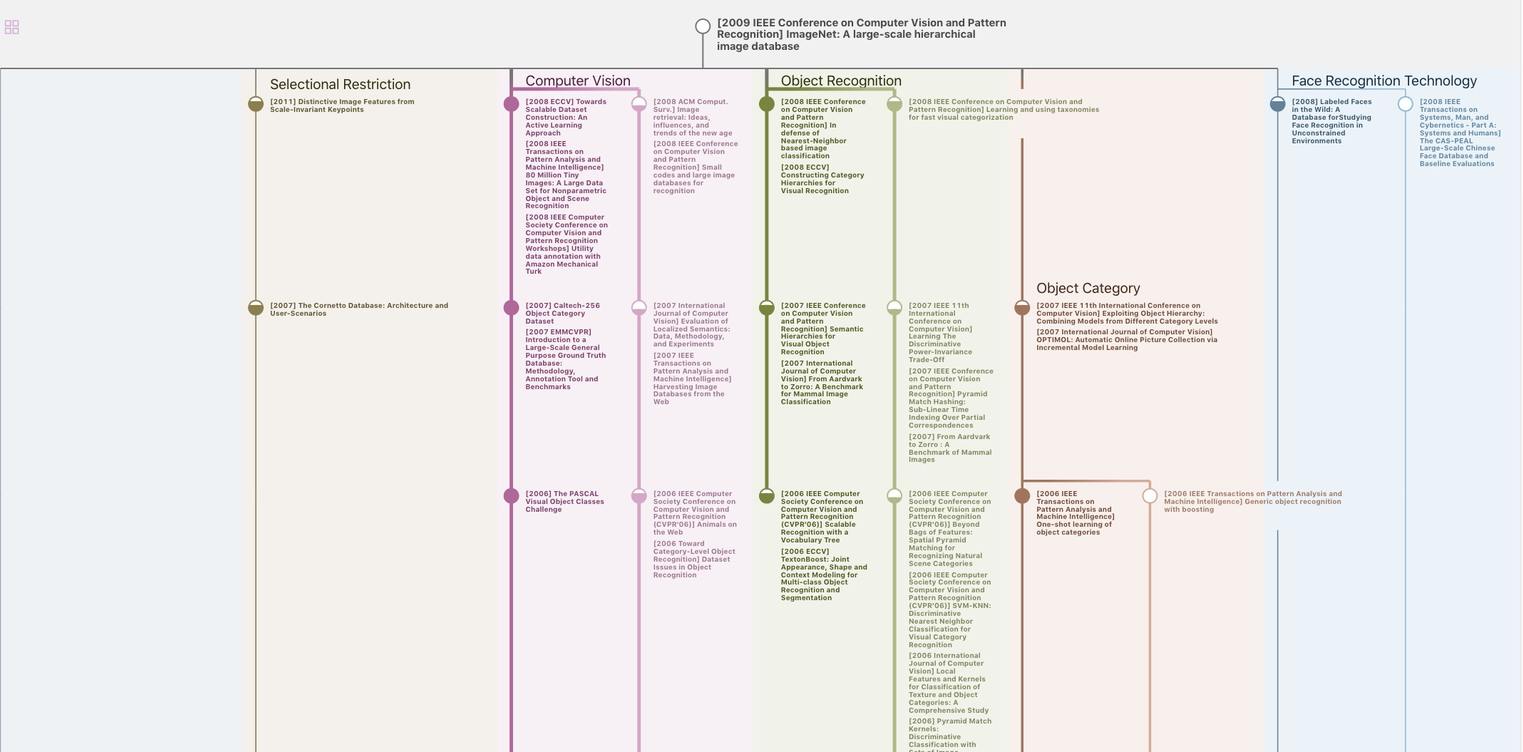
Generate MRT to find the research sequence of this paper
Chat Paper
Summary is being generated by the instructions you defined