A Novel Approach to Incomplete Multimodal Learning for Remote Sensing Data Fusion
IEEE Transactions on Geoscience and Remote Sensing(2024)
摘要
The mechanism of connecting multimodal signals through self-attention operation is a key factor in the success of multimodal Transformer networks in remote sensing data fusion tasks. However, traditional approaches assume access to all modalities during both training and inference, which can lead to severe degradation when dealing with modal-incomplete inputs in downstream applications. To address this limitation, we propose a novel approach to incomplete multimodal learning in the context of remote sensing data fusion and the multimodal Transformer. This approach can be used in both supervised and self-supervised pre-training paradigms. It leverages the additional learned fusion tokens in combination with modality attention and masked self-attention mechanisms to collect multimodal signals in a multimodal Transformer. The proposed approach employs reconstruction and contrastive loss to facilitate fusion in pre-training, while allowing for random modality combinations as inputs in network training. Experimental results show that the proposed method delivers state-of-the-art performance on two multimodal datasets for tasks such as building instance / semantic segmentation and land-cover mapping when dealing with incomplete inputs during inference.
更多查看译文
关键词
Data Fusion,Multimodal,Transformer,Remote Sensing
AI 理解论文
溯源树
样例
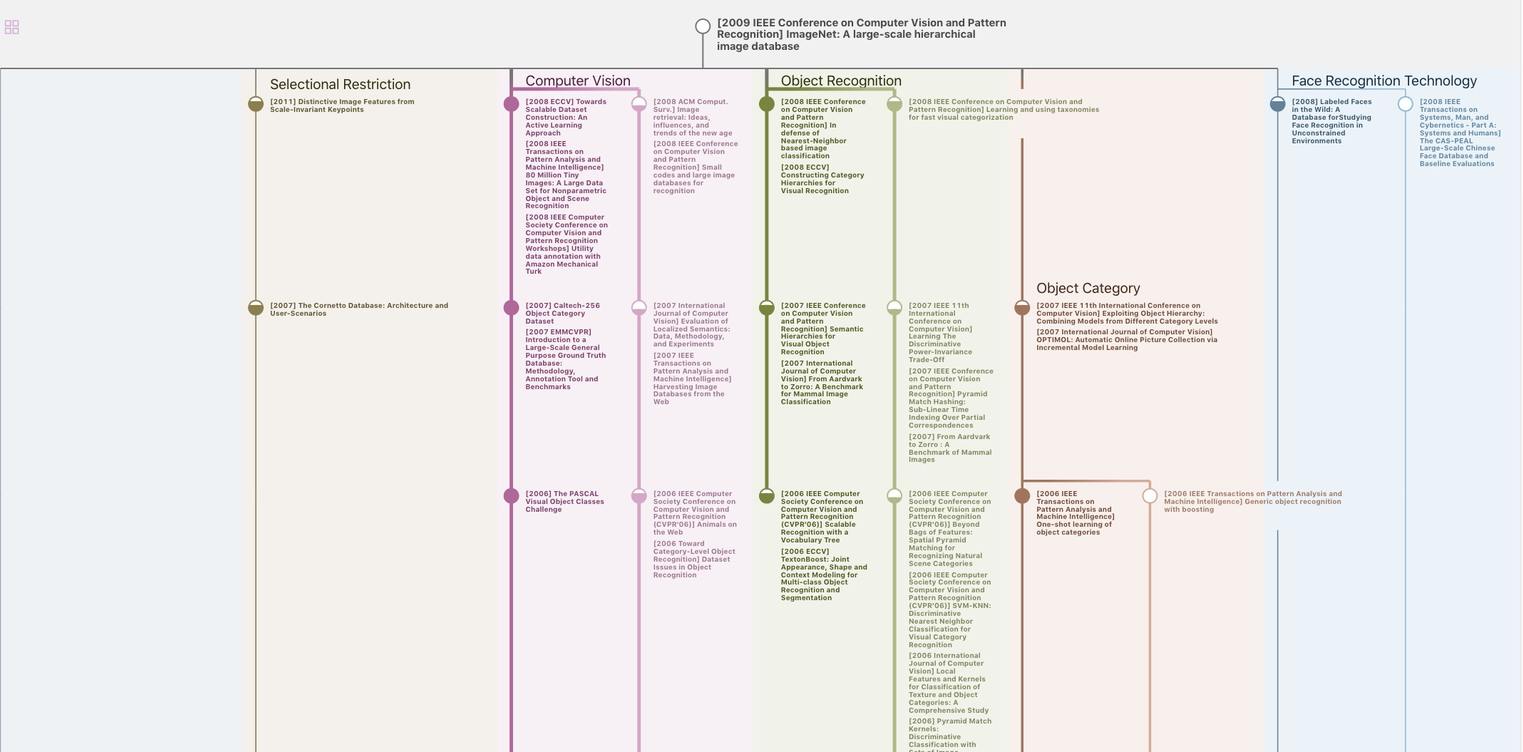
生成溯源树,研究论文发展脉络
Chat Paper
正在生成论文摘要