Knowledge Distillation-Guided Cost-Sensitive Ensemble Learning Framework for Imbalanced Fault Diagnosis
IEEE Internet of Things Journal(2024)
摘要
In industrial scenarios, mechanical faults are episodic and uncertain. Thus the monitoring data collected is usually extremely imbalanced, resulting in intelligent diagnostic models that suffer from majority-class dominance, minority-class overfitting, and poor generalization performance. Therefore, a knowledge distillation-guided cost-sensitive ensemble learning framework is proposed. It effectively combines ensemble learning and cost-sensitive learning to fully extract the multiscale features, effectively leverage the critical multi-depth features, and emphasize classifying the most confusing classes. Specifically, multiple-scale feature extraction and multi-order fusion are first employed to fully utilize the fault information. Afterward, the complementary diagnostic knowledge at different depths of the network is embedded into a novel ensemble learning process for better integration decisions. Then an improved knowledge distillation method achieves the mutual transfer and sublimation of excellent diagnostic knowledge while focusing on the most confusing fault classes to achieve the effective representation of various types of faults. Finally, a cost-sensitive strategy is applied to further increase attention to minority classes. The experimental results for various complex data imbalance scenarios, including extreme imbalance, step imbalance, continuous imbalance, interclass imbalance, and intra-class imbalance, all indicate that the proposed method can achieve state-of-the-art performance and provide a promising solution for the practical industrial application of intelligent diagnostic methods.
更多查看译文
关键词
fault diagnosis,data imbalance,cost sensitive,ensemble learning,knowledge distillation,multi-depth features
AI 理解论文
溯源树
样例
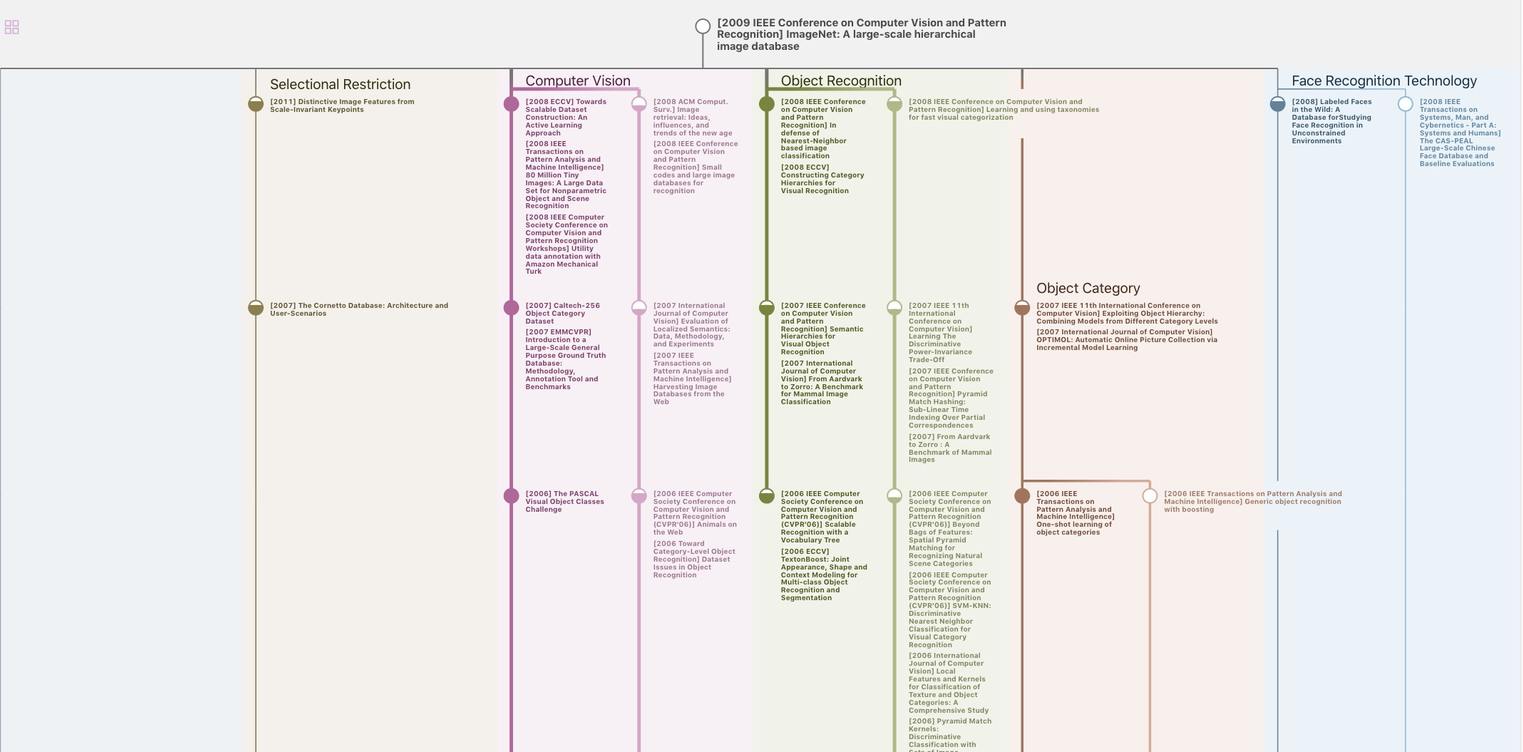
生成溯源树,研究论文发展脉络
Chat Paper
正在生成论文摘要