Multiple reference points-based multi-objective feature selection for multi-label learning
Applied Intelligence(2024)
摘要
In the real world, data often exhibits high-dimensional and complex characteristics. In addition, an object may correspond to multiple class labels. Therefore, filtering and processing such data has become a hot research topic. Multi-label feature selection is one of the key preprocessing techniques for effectively solving redundant information. However, due to the high complexity of multi-label learning feature selection and involving optimization of multiple objectives, careful consideration is required to achieve effective optimization. To address these issues, this paper proposes a multiple reference points-based multi-objective feature selection for multi-label learning. Firstly, the neighborhood crossover strategy utilizes individuals in the same neighborhood for crossover, thereby enhancing the search effect within that decision space. The distribution-based mutation strategy adjusts the mutation probability of different features based on their distribution in selected candidate solutions to increase the likelihood of potentially useful features being selected. Secondly, the multiple reference points-based selection strategy uses a scalar function to uniformly evaluate offspring individuals and control their distance from each other through multiple reference points. This approach helps in selecting convergent solutions with balanced diversity. Finally, experimental results demonstrate that the proposed algorithm attains an optimal performance of 83
更多查看译文
关键词
Feature selection,Multi-label learning,Multi-objective optimization,Multiple reference points,Evolutionary algorithm
AI 理解论文
溯源树
样例
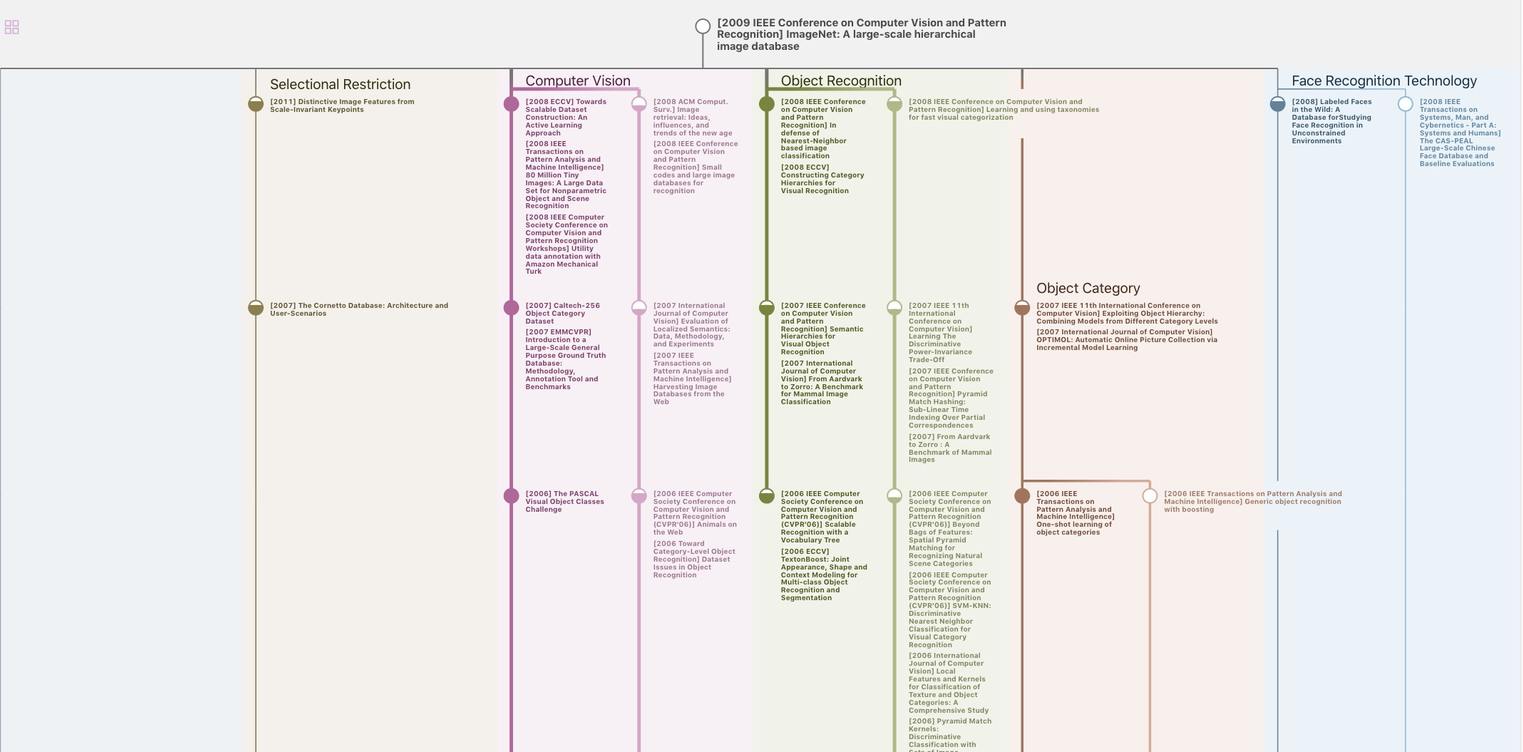
生成溯源树,研究论文发展脉络
Chat Paper
正在生成论文摘要