A Progressive Decoupled Network for Blind Image Super-Resolution
IEEE ACCESS(2024)
Abstract
Blind super-resolution (Blind SR) has become a popular research topic in computer vision in super-resolution, which aims to enhance low-resolution (LR) images with unknown or partially known degradation blur kernels. At present, most existing methods need to estimate the degradation kernel first and then use the estimated degradation kernel to get the final super-resolution result. However, degradation kernels are expected to burden the network. Moreover, the estimated degradation kernels are often inaccurate. Most existing methods fail to estimate blur kernels to produce satisfactory restoration results. In this paper, we propose a blind SR model based on deep learning, namely A Progressive Decoupled Network for Blind Super-Resolution (PDNet). The method differs from other methods since it decouples the degradation information from the super-resolution content and does not estimate the degradation kernel. The proposed method separates the degradation blur kernel while preserving super-resolution components progressively from degradation low-resolution images. To this end, a dual-path architecture is constructed, learning the combined features and the mutual relationships. Specifically, we design a decoupled representation module to exploit the coarse feature and a decoupled cross-attention module to separate the super-resolution and degradation content from the combined features. Our model can effectively recover super-resolution images with high fidelity and preserve edge details. The comprehensive experiments are conducted on various datasets and evaluate the performance of the proposed method against state-of-the-art approaches. The results demonstrate that our model outperforms existing methods in terms of both quantitative and qualitative evaluations.
MoreTranslated text
Key words
Degradation,Superresolution,Feature extraction,Data mining,Convolutional neural networks,Noise measurement,Image resolution,Image restoration,Image edge detection,Computer vision,Computer architecture,Deep learning,Blind super-resolution,blur kernel,decoupled network,dual-path architecture,progressive strategy
AI Read Science
Must-Reading Tree
Example
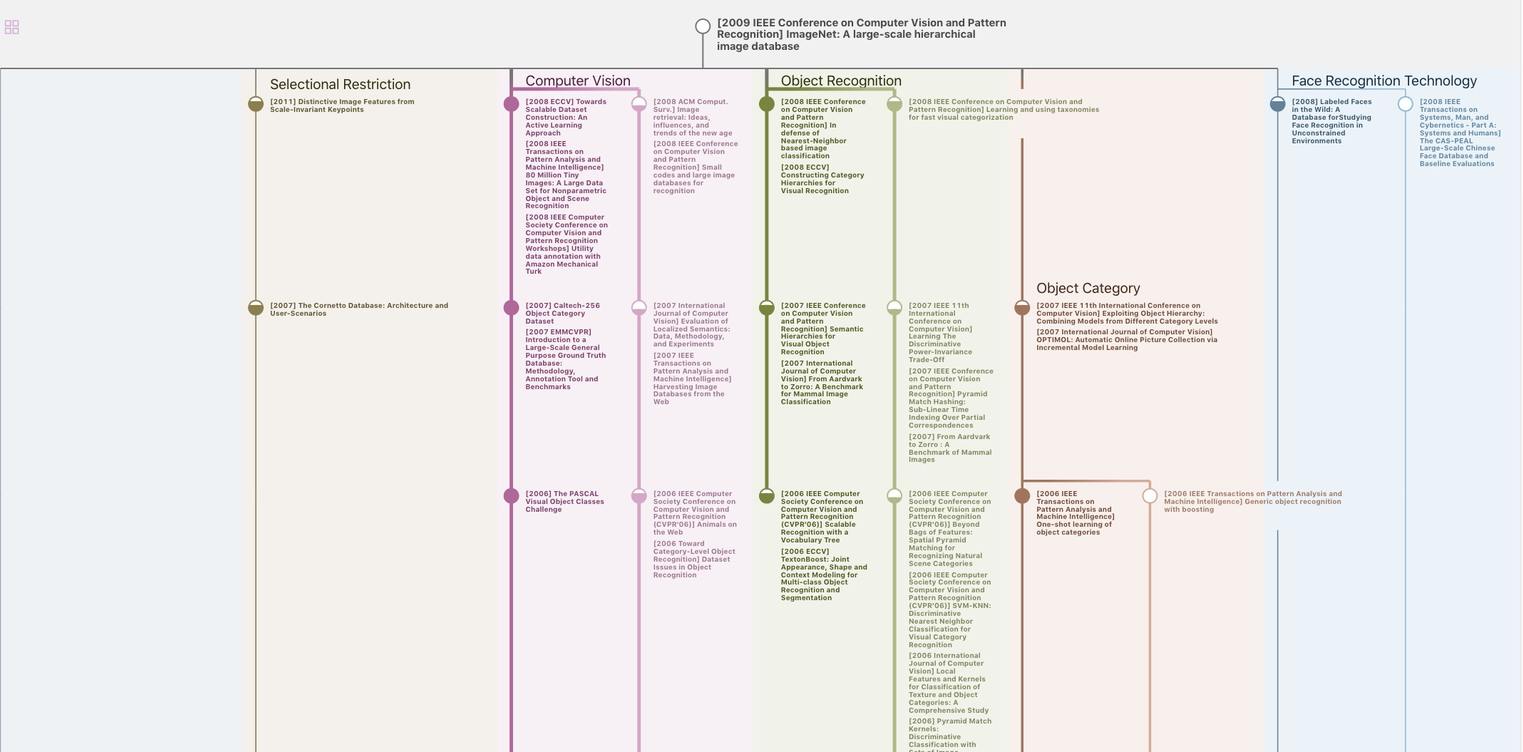
Generate MRT to find the research sequence of this paper
Chat Paper
Summary is being generated by the instructions you defined