LsiA3CS: Deep Reinforcement Learning-Based Cloud-Edge Collaborative Task Scheduling in Large-Scale IIoT
IEEE Internet of Things Journal(2024)
摘要
Task scheduling in large-scale industrial Internet of Things (IIoT) is characterized by the presence of diverse resources and the requirement for efficient and synchronized processing across distributed edge clouds, raising a significant challenge. This paper proposes a task scheduling framework across edge clouds, namely LsiA3CS, which employs deep reinforcement learning (DRL) and heuristic guidance to achieve distributed, asynchronous task scheduling for large-scale IIoT. Specifically, the Markov game-based model and the asynchronous advantage actor-critic (A3C) algorithm are leveraged to orchestrate diverse computational resources, effectively balancing workloads and reducing communication latency. Moreover, the incorporation of heuristic policy annealing and action masking techniques further refines the adaptability of the proposed framework to the unpredictable requirements of large-scale IIoT systems. Real-world task datasets are utilized to conduct extensive experimental evaluations on a simulated large-scale multi-edge cloud IIoT. The results shows that LsiA3CS significantly reduces task completion times and energy consumption while managing unpredictable task arrivals and variable resource capacities.
更多查看译文
关键词
Large-scale IIoT,task scheduling,asynchronous advantage actor-critic (A3C),deep reinforcement learning (DRL),heuristic algorithm
AI 理解论文
溯源树
样例
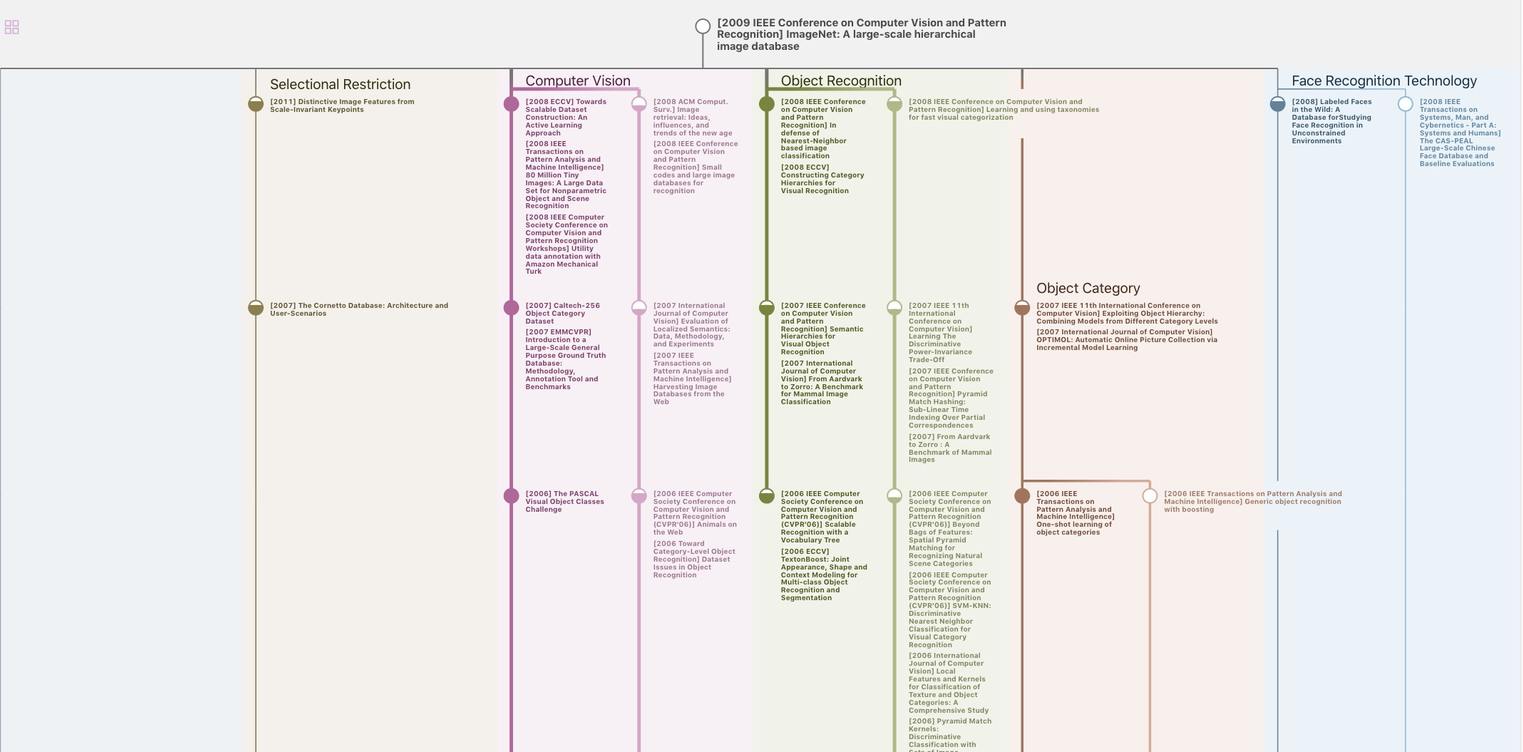
生成溯源树,研究论文发展脉络
Chat Paper
正在生成论文摘要