In-Season Cotton Yield Prediction with Scale-Aware Convolutional Neural Network Models and Unmanned Aerial Vehicle RGB Imagery.
Sensors (Basel, Switzerland)(2024)
摘要
In the pursuit of sustainable agriculture, efficient water management remains crucial, with growers relying on advanced techniques for informed decision-making. Cotton yield prediction, a critical aspect of agricultural planning, benefits from cutting-edge technologies. However, traditional methods often struggle to capture the nuanced complexities of crop health and growth. This study introduces a novel approach to cotton yield prediction, leveraging the synergy between Unmanned Aerial Vehicles (UAVs) and scale-aware convolutional neural networks (CNNs). The proposed model seeks to harness the spatiotemporal dynamics inherent in high-resolution UAV imagery to improve the accuracy of the cotton yield prediction. The CNN component adeptly extracts spatial features from UAV-derived imagery, capturing intricate details related to crop health and growth, modeling temporal dependencies, and facilitating the recognition of trends and patterns over time. Research experiments were carried out in a cotton field at the USDA-ARS Cropping Systems Research Laboratory (CSRL) in Lubbock, Texas, with three replications evaluating four irrigation treatments (rainfed, full irrigation, percent deficit of full irrigation, and time delay of full irrigation) on cotton yield. The prediction revealed that the proposed CNN regression models outperformed conventional CNN models, such as AlexNet, CNN-3D, CNN-LSTM, ResNet. The proposed CNN model showed state-of-art performance at different image scales, with the R2 exceeding 0.9. At the cotton row level, the mean absolute error (MAE) and mean absolute percentage error (MAPE) were 3.08 pounds per row and 7.76%, respectively. At the cotton grid level, the MAE and MAPE were 0.05 pounds and 10%, respectively. This shows the proposed model's adaptability to the dynamic interplay between spatial and temporal factors that affect cotton yield. The authors conclude that integrating UAV-derived imagery and CNN regression models is a potent strategy for advancing precision agriculture, providing growers with a powerful tool to optimize cultivation practices and enhance overall cotton productivity.
更多查看译文
关键词
cotton,irrigation,UAV,convolutional neural networks,yield
AI 理解论文
溯源树
样例
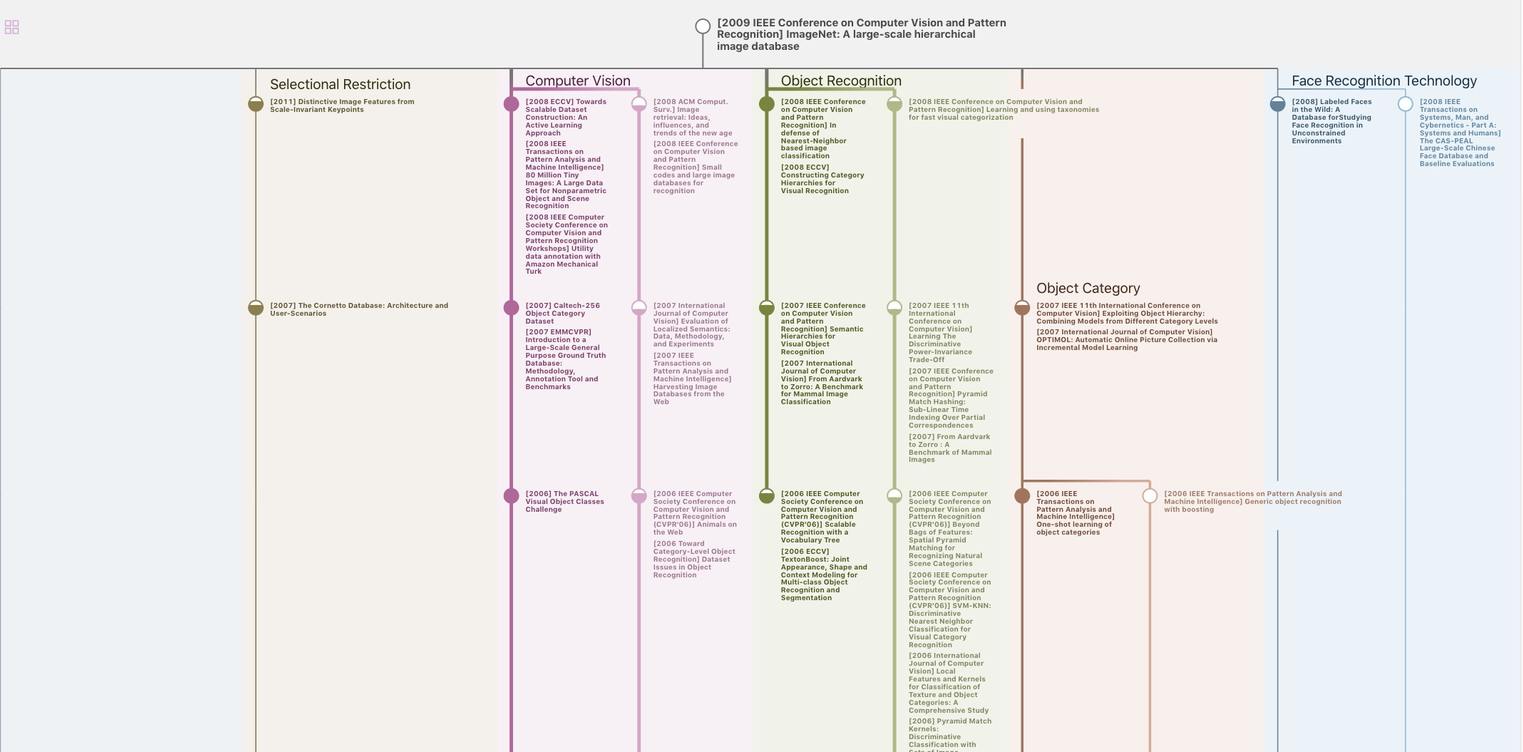
生成溯源树,研究论文发展脉络
Chat Paper
正在生成论文摘要