Adversarial purification for no-reference image-quality metrics: applicability study and new methods
arxiv(2024)
摘要
Recently, the area of adversarial attacks on image quality metrics has begun
to be explored, whereas the area of defences remains under-researched. In this
study, we aim to cover that case and check the transferability of adversarial
purification defences from image classifiers to IQA methods. In this paper, we
apply several widespread attacks on IQA models and examine the success of the
defences against them. The purification methodologies covered different
preprocessing techniques, including geometrical transformations, compression,
denoising, and modern neural network-based methods. Also, we address the
challenge of assessing the efficacy of a defensive methodology by proposing
ways to estimate output visual quality and the success of neutralizing attacks.
Defences were tested against attack on three IQA metrics – Linearity, MetaIQA
and SPAQ. The code for attacks and defences is available at: (link is hidden
for a blind review).
更多查看译文
AI 理解论文
溯源树
样例
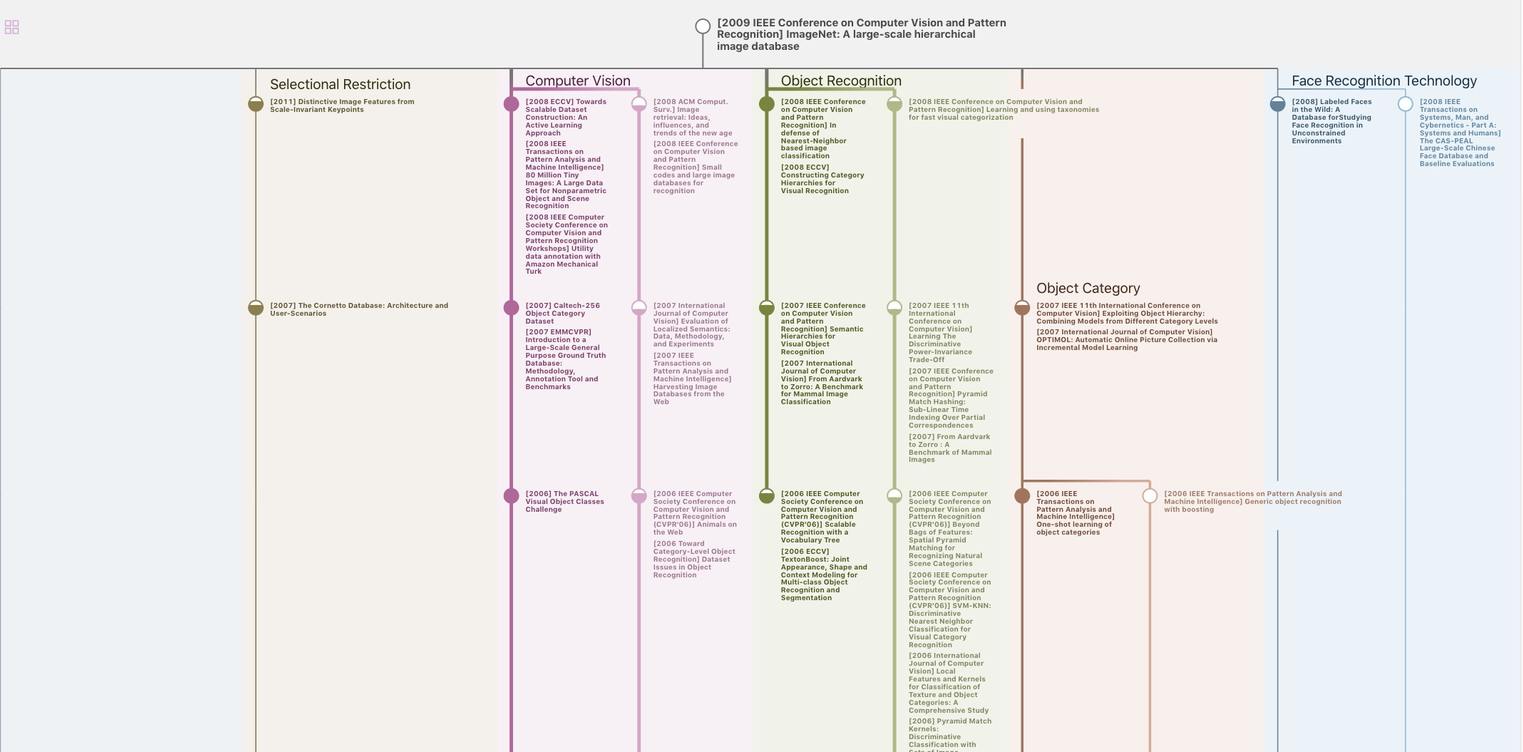
生成溯源树,研究论文发展脉络
Chat Paper
正在生成论文摘要