Adapting LLaMA Decoder to Vision Transformer
arxiv(2024)
摘要
This work examines whether decoder-only Transformers such as LLaMA, which
were originally designed for large language models (LLMs), can be adapted to
the computer vision field. We first "LLaMAfy" a standard ViT step-by-step to
align with LLaMA's architecture, and find that directly applying a causal mask
to the self-attention brings an attention collapse issue, resulting in the
failure to the network training. We suggest to reposition the class token
behind the image tokens with a post-sequence class token technique to overcome
this challenge, enabling causal self-attention to efficiently capture the
entire image's information. Additionally, we develop a soft mask strategy that
gradually introduces a causal mask to the self-attention at the onset of
training to facilitate the optimization behavior. The tailored model, dubbed as
image LLaMA (iLLaMA), is akin to LLaMA in architecture and enables direct
supervised learning. Its causal self-attention boosts computational efficiency
and learns complex representation by elevating attention map ranks. iLLaMA
rivals the performance with its encoder-only counterparts, achieving 75.1
ImageNet top-1 accuracy with only 5.7M parameters. Scaling the model to
∼310M and pre-training on ImageNet-21K further enhances the accuracy to
86.0
shape-texture bias, calibration, quantization compatibility, ADE20K
segmentation and CIFAR transfer learning. We hope our study can kindle fresh
views to visual architectures in the wave of LLMs and inspire the development
of unified multimodal models. Pre-trained models and codes are available
https://github.com/techmonsterwang/iLLaMA.
更多查看译文
AI 理解论文
溯源树
样例
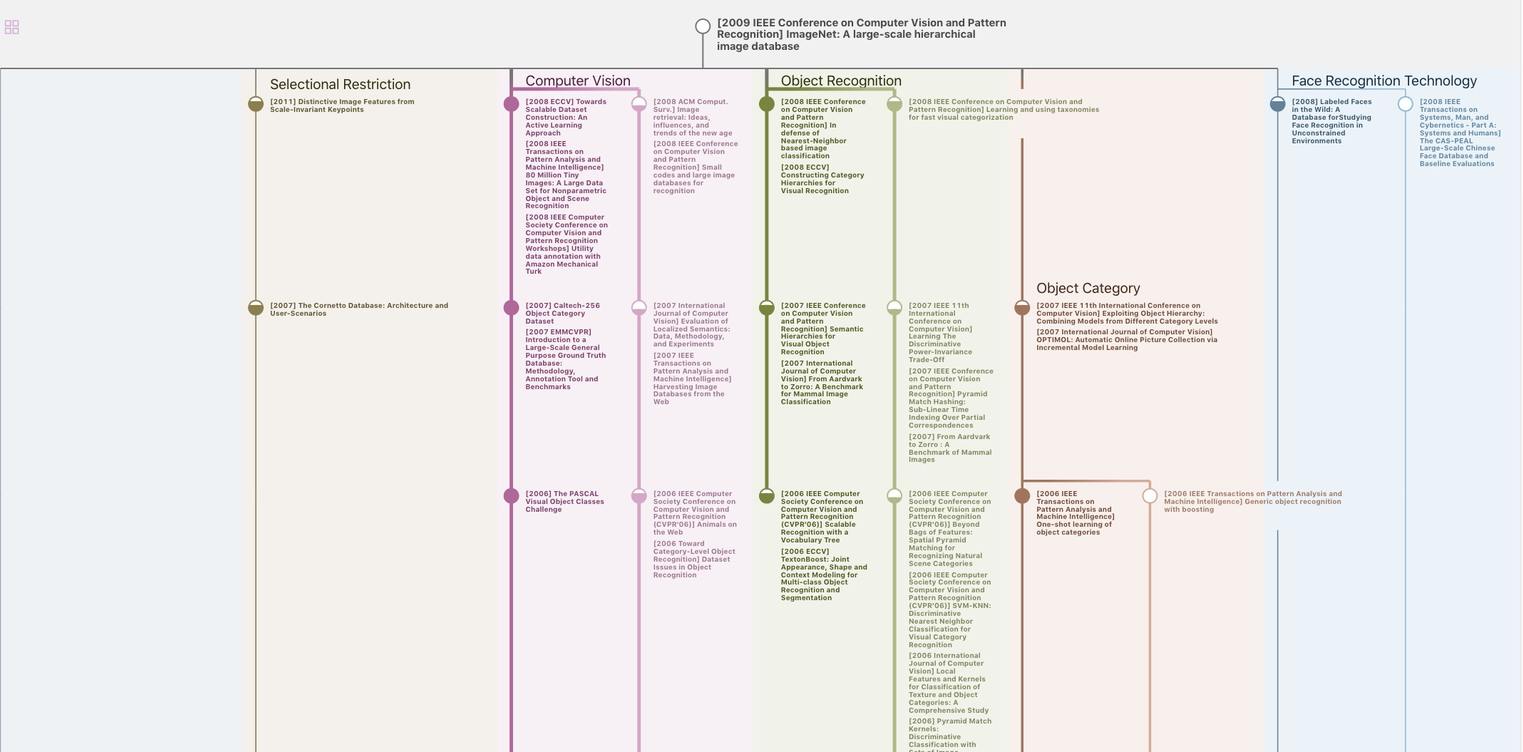
生成溯源树,研究论文发展脉络
Chat Paper
正在生成论文摘要