Covariance Regression with High-Dimensional Predictors
arxiv(2024)
摘要
In the high-dimensional landscape, addressing the challenges of covariance
regression with high-dimensional covariates has posed difficulties for
conventional methodologies. This paper addresses these hurdles by presenting a
novel approach for high-dimensional inference with covariance matrix outcomes.
The proposed methodology is illustrated through its application in elucidating
brain coactivation patterns observed in functional magnetic resonance imaging
(fMRI) experiments and unraveling complex associations within anatomical
connections between brain regions identified through diffusion tensor imaging
(DTI). In the pursuit of dependable statistical inference, we introduce an
integrative approach based on penalized estimation. This approach combines data
splitting, variable selection, aggregation of low-dimensional estimators, and
robust variance estimation. It enables the construction of reliable confidence
intervals for covariate coefficients, supported by theoretical confidence
levels under specified conditions, where asymptotic distributions are provided.
Through various types of simulation studies, the proposed approach performs
well for covariance regression in the presence of high-dimensional covariates.
This innovative approach is applied to the Lifespan Human Connectome Project
(HCP) Aging Study, which aims to uncover a typical aging trajectory and
variations in the brain connectome among mature and older adults. The proposed
approach effectively identifies brain networks and associated predictors of
white matter integrity, aligning with established knowledge of the human brain.
更多查看译文
AI 理解论文
溯源树
样例
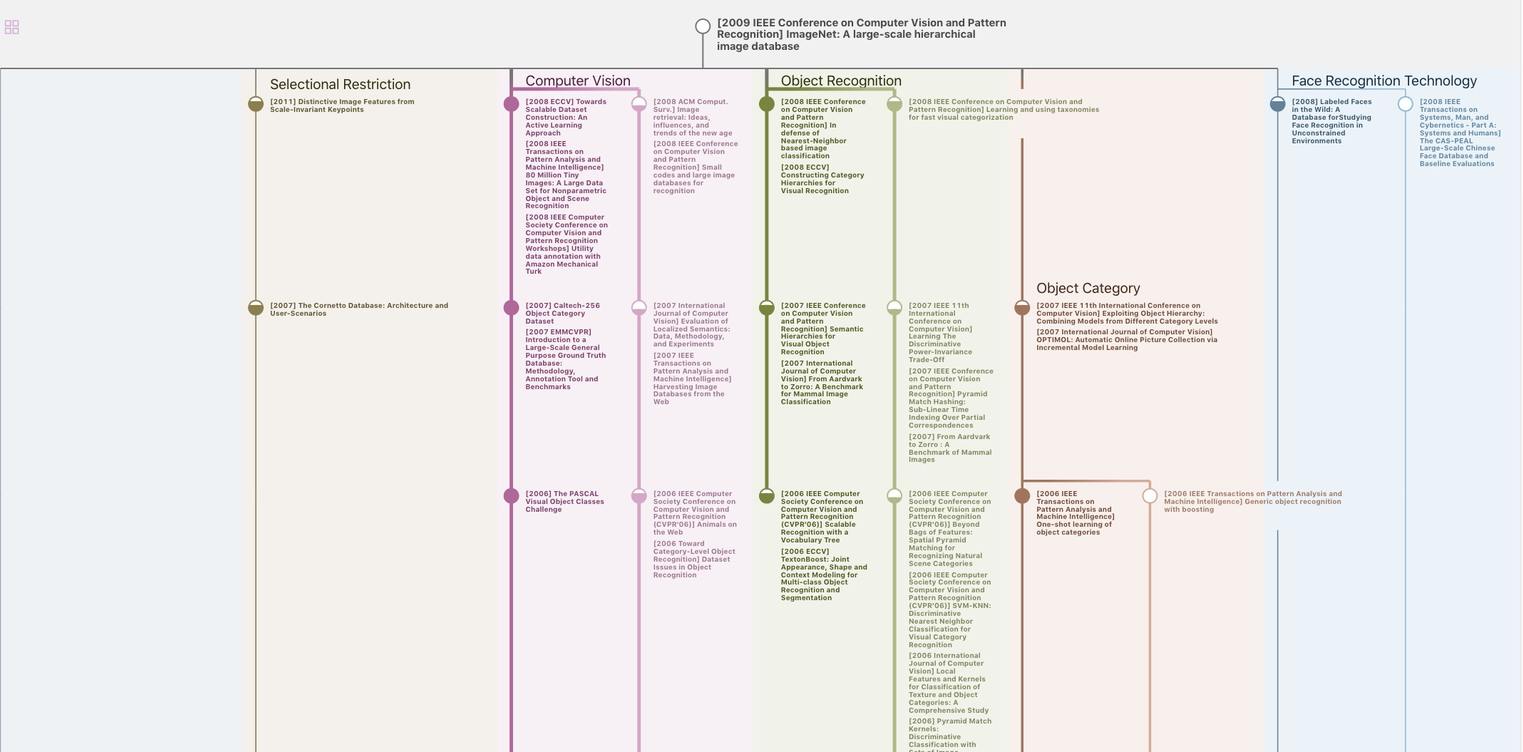
生成溯源树,研究论文发展脉络
Chat Paper
正在生成论文摘要