Toward Cross-Layer Energy Optimizations in Machine Learning Systems
arxiv(2024)
摘要
The enormous energy consumption of machine learning (ML) and generative AI
workloads shows no sign of waning, taking a toll on operating costs, power
delivery, and environmental sustainability. Despite a long line of research on
energy-efficient hardware, we found that software plays a critical role in ML
energy optimization through two recent works: Zeus and Perseus. This is
especially true for large language models (LLMs) because their model sizes and,
therefore, energy demands are growing faster than hardware efficiency
improvements. Therefore, we advocate for a cross-layer approach for energy
optimizations in ML systems, where hardware provides architectural support that
pushes energy-efficient software further, while software leverages and
abstracts the hardware to develop techniques that bring hardware-agnostic
energy-efficiency gains.
更多查看译文
AI 理解论文
溯源树
样例
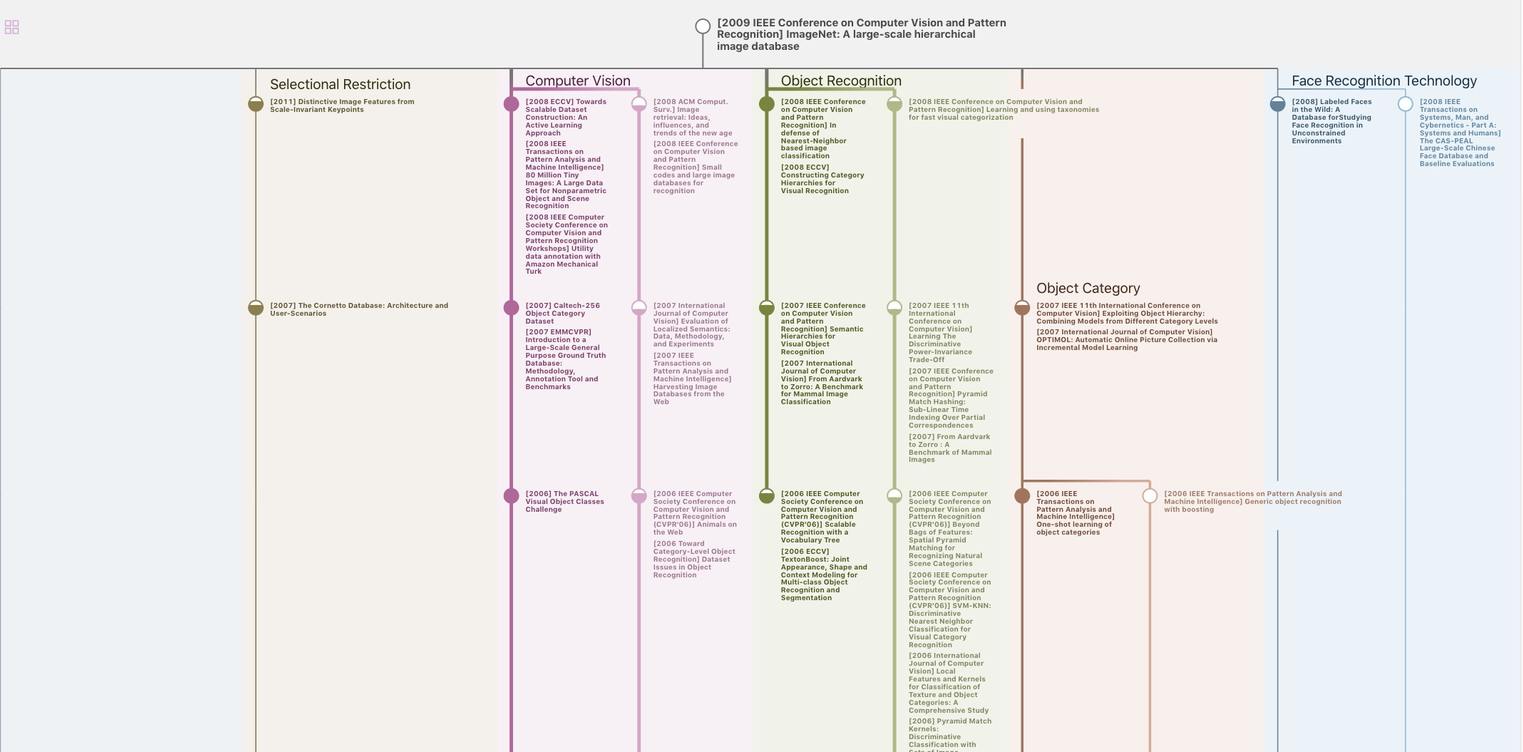
生成溯源树,研究论文发展脉络
Chat Paper
正在生成论文摘要