Transferring Multi-Modal Domain Knowledge to Uni-Modal Domain for Urban Scene Segmentation
IEEE TRANSACTIONS ON INTELLIGENT TRANSPORTATION SYSTEMS(2024)
Abstract
Synthetic data (i.e., source domain) have been widely adopted to improve the semantic segmentation performance for real-world images (i.e., target domain), since obtaining pixel-level annotations is fairly easy in the synthetic environment. Traditional domain adaptation methods normally focus on learning in the RGB modality only. We notice that the synthetic environment can generate depth information of semantic objects at almost no cost, while it is nontrivial to collect such information in the real-world scenario. In this case, we employ the depth information of synthetic data in this work to further boost the segmentation performance, and then transform the uni-modal problem into a multi-modal one. In this work, we focus on urban scene understanding and make a pioneer attempt on learning uni-modal feature representations for real-world images by mining from multi-modal knowledge of synthetic images with additional depth information. To this end, we propose a novel method called Multi-modal Domain Knowledge Transfer (MDKT), which transfers the multi-modal knowledge of the source domain to the uni-modal target domain through domain adaptation. In MDKT, we first employ the Cross-Modal Correlation (CMC) module to enhance the source features by fusing the RGB and depth information. Then, the uni-modal target domain feature and multi-modal source domain feature are aligned through the Modal-Imbalanced Adversarial Training (MIAT) strategy, which transfers the multi-modal knowledge to the uni-modal network in the target domain. We conduct extensive experiments on several benchmark settings for urban scene understanding. The promising results clearly show the effectiveness of our proposed MDKT approach.
MoreTranslated text
Key words
Urban scene understanding,domain adaptation,semantic segmentation,multi-modal learning
AI Read Science
Must-Reading Tree
Example
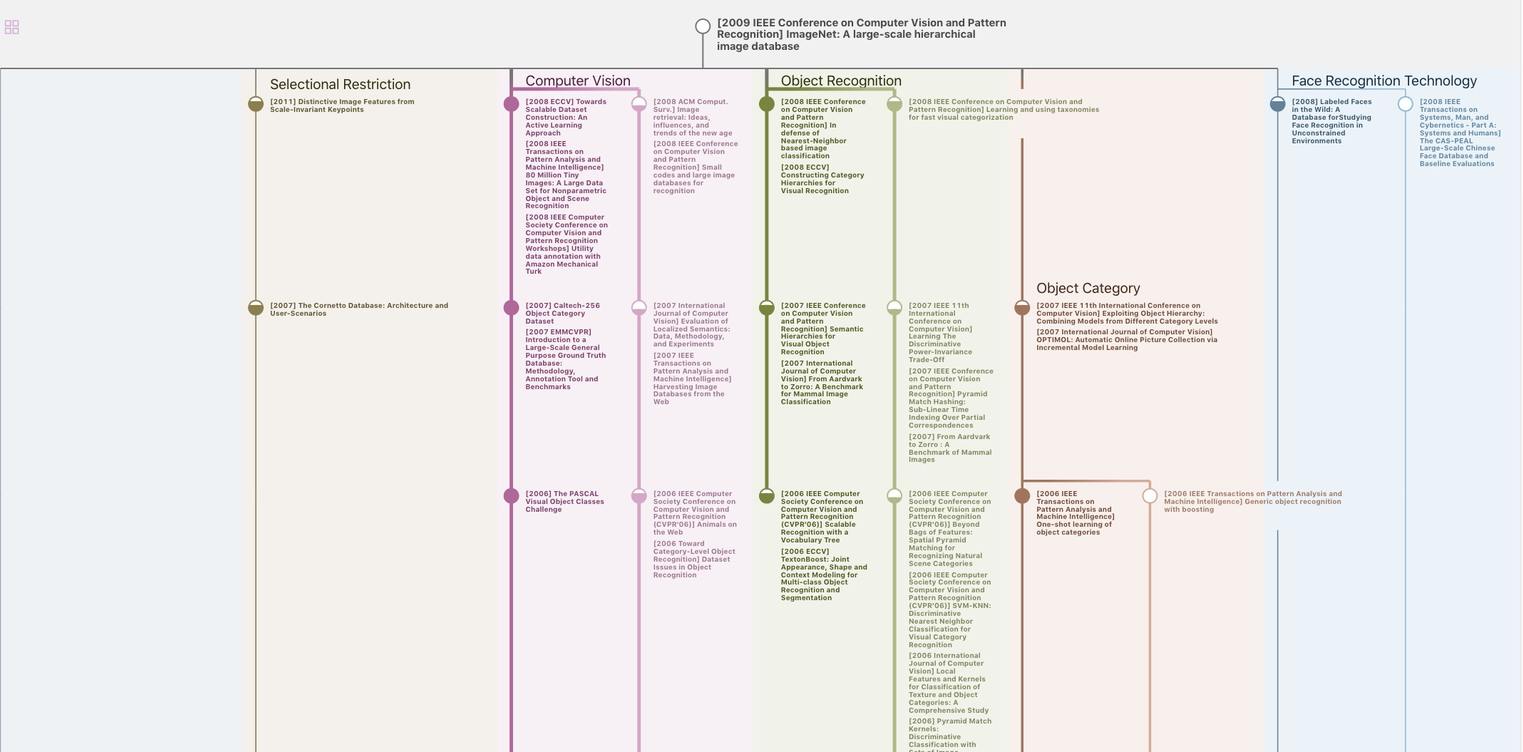
Generate MRT to find the research sequence of this paper
Chat Paper
Summary is being generated by the instructions you defined