Accurate State of Health Estimation of Battery System based on Multi-stage Constant Current Charging and Behavior Analysis in Real-world Electric Vehicles
IEEE Transactions on Transportation Electrification(2024)
Abstract
With the burgeoning development of electric vehicles (EVs), the efficient and economical charging mode, represented by the multi-stage constant current (MsCC) protocol, becomes increasingly popular during daily usage. In this paper, the health indicators (HIs) extracted from the MsCC charging data sets based on the charging behaviour analysis of real-world EVs are discussed, and a novel data-driven strategy for battery state of health (SOH) estimation for the MsCC charging process is proposed. First, the battery HIs are acquired from the MsCC charging datasets and delicately sifted according to the user behaviour of real-world EVs. Next, the correlation between HIs and battery SOH is analyzed through a Pearson correlation coefficients (PCC) matrix to select highly relevant HIs and avoid redundancy. Finally, the mapping relationship is constructed by the long short-term memory (LSTM) model, and its effectiveness is verified by comparison with the other three methods. To emphasize the robustness and generality of the proposed strategy, two training and testing settings and different kinds of MsCC test datasets are applied to test the model performance. The results show that the LSTM-based structure realizes about 32%, 36%, and 49% improvement in battery SOH estimation compared to the benchmark methods, and a promotion of about 18%, 27%, and 10% in prediction accuracy is observed based on another dataset.
MoreTranslated text
Key words
Battery ageing assessment,data-driven method,long short-term memory,real-world datasets,behaviour analysis
AI Read Science
Must-Reading Tree
Example
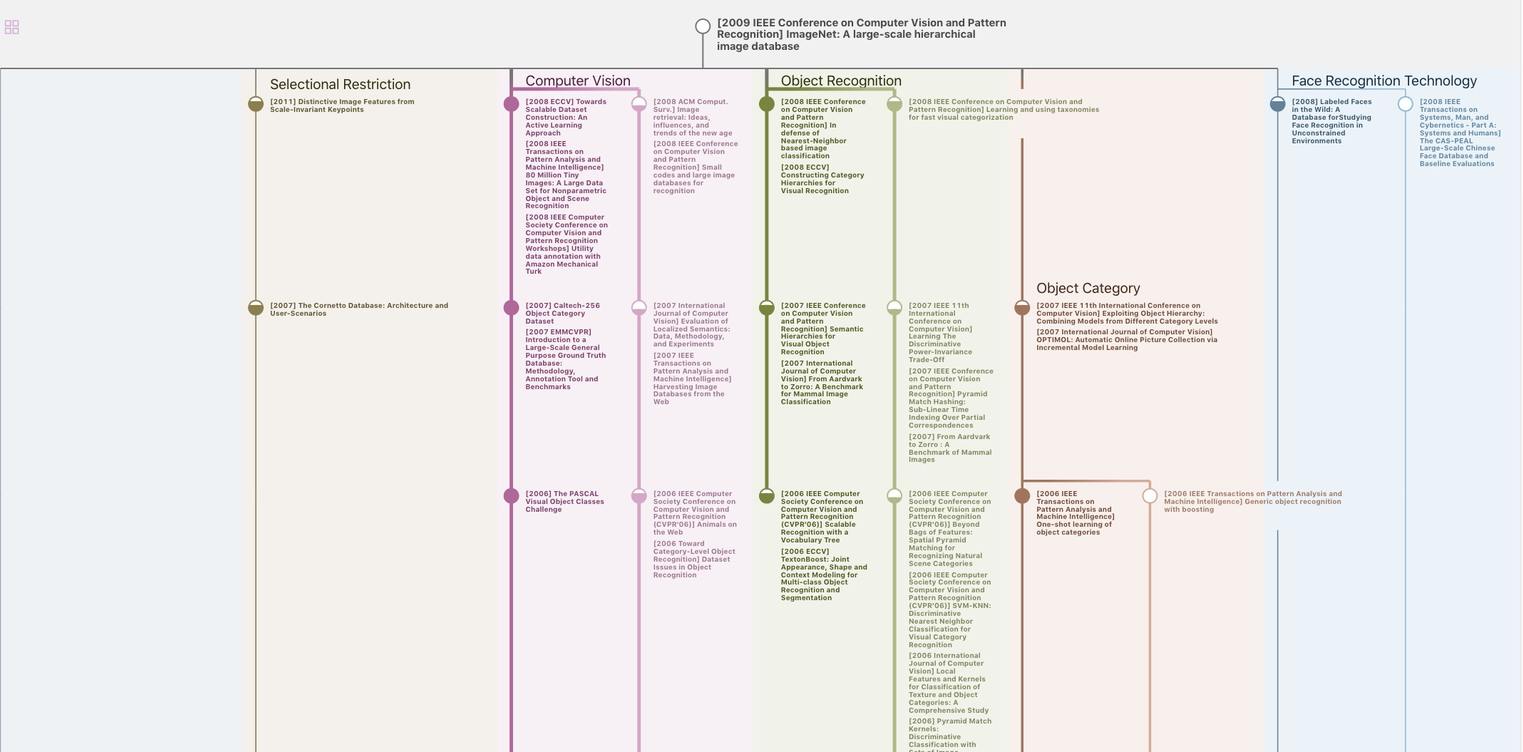
Generate MRT to find the research sequence of this paper
Chat Paper
Summary is being generated by the instructions you defined