Semantic segmentation for multisource remote sensing images incorporating feature slice reconstruction and attention upsampling
INTERNATIONAL JOURNAL OF REMOTE SENSING(2024)
摘要
Multisource remote sensing images have rich features and high interpretability and are widely employed in many applications. However, highly unbalanced category distributions and complex backgrounds have created some difficulties in the application of remote sensing image semantic segmentation tasks, such as low accuracy of small target segmentation and inaccurate edge extraction. To solve these problems, in this paper, a feature map segmentation reconstruction module and an attention upsampling module are proposed. In the encoder part, the input feature map is equally segmented, and the segmented feature map is enlarged to effectively improve the small target feature information expression ability in the model. In the decoder part, the key segmentation and location information of shallow features are obtained using the global view. The deep semantic information and shallow spatial location information are fully combined to achieve a more refined upsampling operation. In addition, the attention mechanism of the spatial and channel squeeze and excitation block (scSE) is applied to pay more attention to important features and to suppress irrelevant background and redundant information. To verify the effectiveness of the proposed method, the WHU-OPT-SAR dataset and six state-of-the-art algorithms are utilized in comparative experiments. The experimental results show that our model has demonstrated the best performance and low computational complexity. With only approximately half the floating-point operation count and the number of model parameters of the MCANet model, which is specially designed for the dataset, our model surpasses MCANet by 1.52% and 1.53% in terms of mean intersection over union (mIoU) and F1 score, respectively. In particular, for small object regions such as roads and other categories, compared to the baseline model, the IoU and F1 score of our model are improved by 5.27% and 3.99% and by 5.68% and 5.65%, respectively. These results demonstrate the superior performance of our model in terms of accuracy and efficiency.
更多查看译文
关键词
Remote sensing images,feature slice reconstruction,attention upsampling,attention mechanism,semantic segmentation
AI 理解论文
溯源树
样例
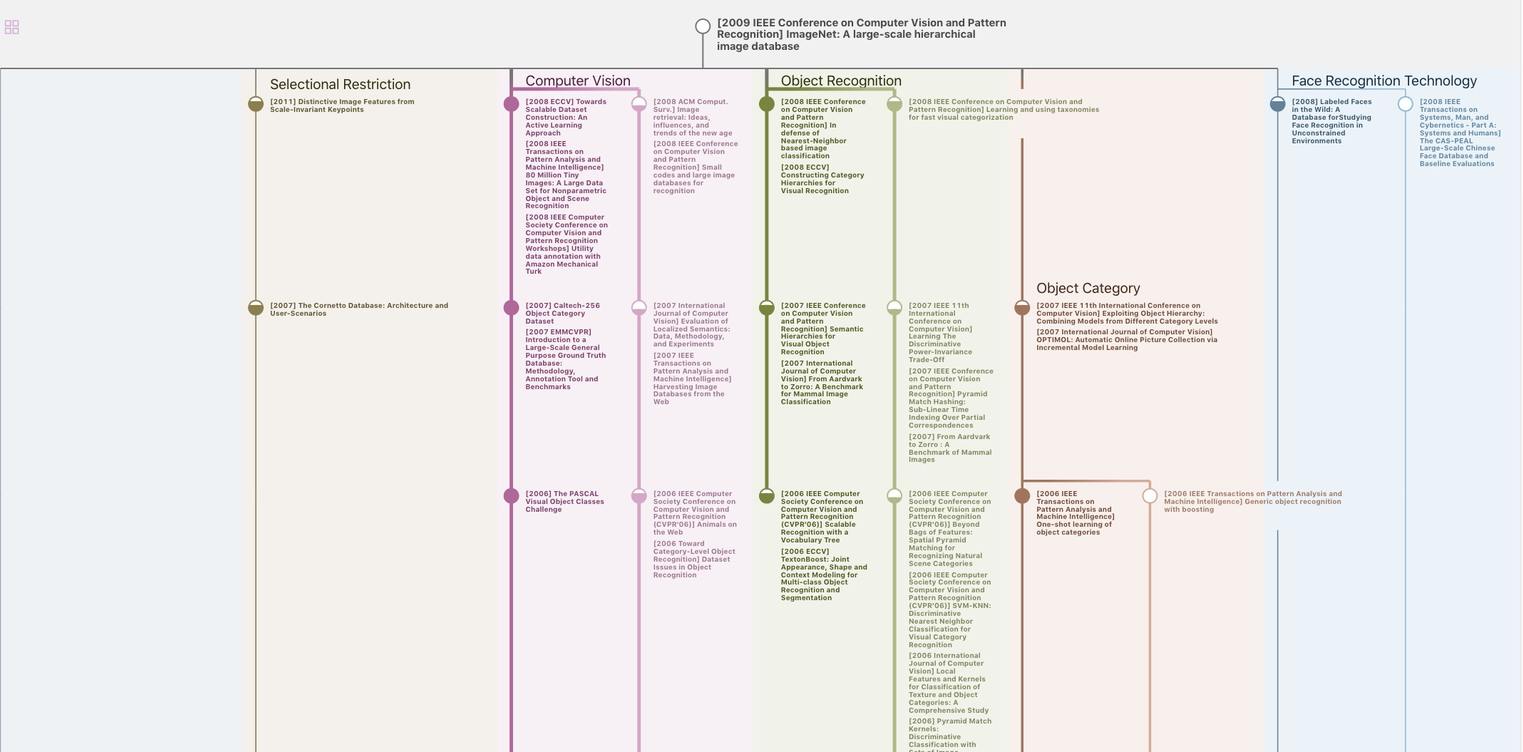
生成溯源树,研究论文发展脉络
Chat Paper
正在生成论文摘要