Predicting Rutting Development Using Machine Learning Methods Based on RIOCHTrack Data
Applied Sciences(2024)
摘要
As the main cause of asphalt pavement distress, rutting severely affects pavement safety. Establishing an accurate rutting prediction model is crucial for asphalt pavement maintenance, pavement structure design, and pavement repair. This study explores five machine learning methods, namely Support Vector Regression (SVR), Artificial Neural Network (ANN), Gradient Boosting Decision Tree (GBDT), Random Forest (RF), and Extra Trees, to predict the development of rutting depth using data from RIOHTRack. The model’s performance is measured by comparing the performance evaluation indicators of different models, such as the coefficient of determination, root mean square error, mean absolute error, and mean absolute percentage error. The results demonstrate that integrated learning techniques such as RF, GBDT, and Extra Trees works best with R2 = 0.9761, 0.9833, and 0.9747. Moreover, the GBFT model can capture the trend of the measured rutting progression curve better than the mechanistic-empirical (M-E) model. The analysis of feature importance reveals that, in addition to external factors such as temperature and axle load, the aggregate of the asphalt concrete layer and air void crucially affect rutting. The higher the base strength, the smaller the rutting depth. The proposed model is highly straightforward and serves as an accessible analysis tool for engineers in practice.
更多查看译文
关键词
rutting prediction,machine learning,RIOHTrack,pavement performance,SHAP
AI 理解论文
溯源树
样例
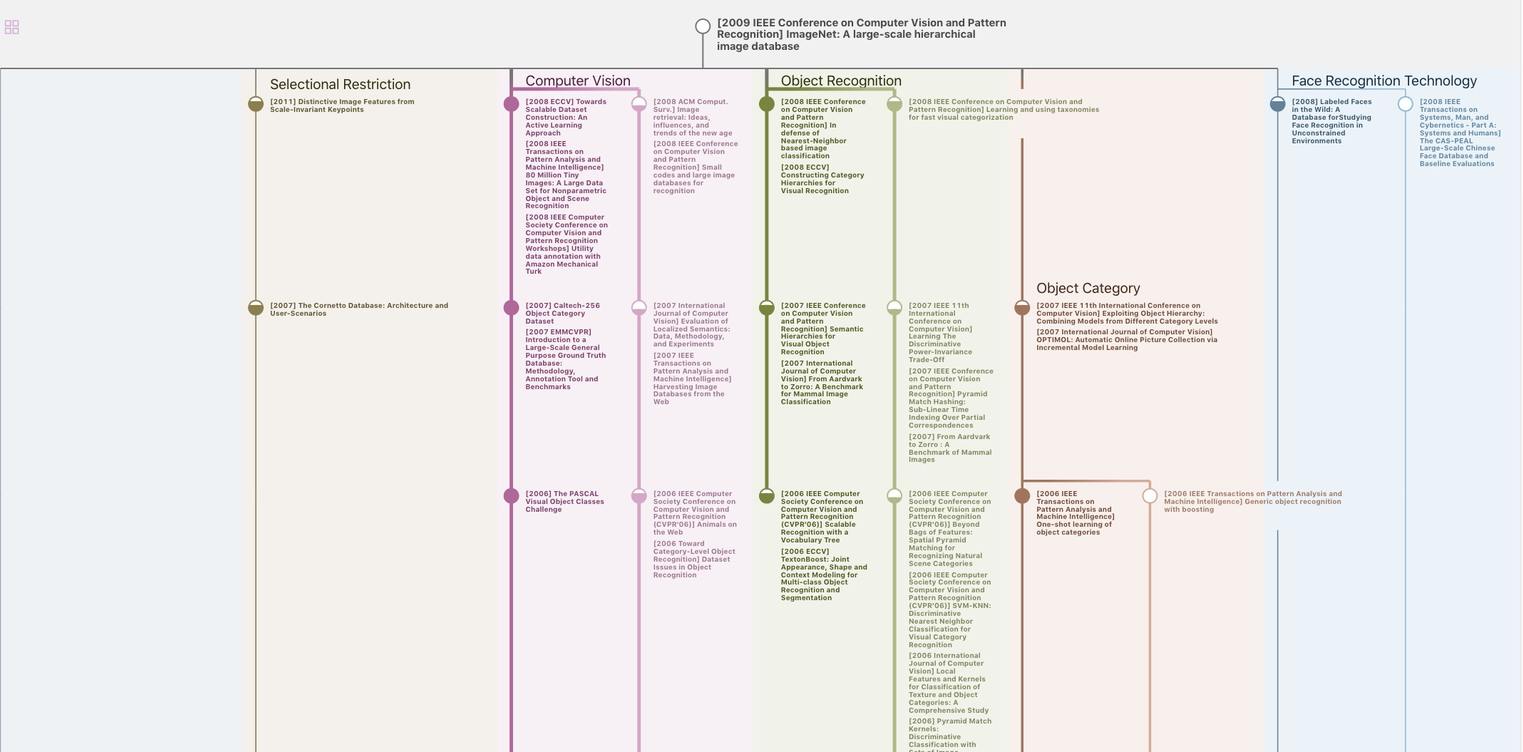
生成溯源树,研究论文发展脉络
Chat Paper
正在生成论文摘要