Toward Precision Diagnosis: Machine Learning in Identifying Malignant Orbital Tumors With Multiparametric 3 T MRI.
Investigative radiology(2024)
摘要
BACKGROUND:Orbital tumors present a diagnostic challenge due to their varied locations and histopathological differences. Although recent advancements in imaging have improved diagnosis, classification remains a challenge. The integration of artificial intelligence in radiology and ophthalmology has demonstrated promising outcomes.
PURPOSE:This study aimed to evaluate the performance of machine learning models in accurately distinguishing malignant orbital tumors from benign ones using multiparametric 3 T magnetic resonance imaging (MRI) data.
MATERIALS AND METHODS:In this single-center prospective study, patients with orbital masses underwent presurgery 3 T MRI scans between December 2015 and May 2021. The MRI protocol comprised multiparametric imaging including dynamic contrast-enhanced (DCE), diffusion-weighted imaging (DWI), intravoxel incoherent motion (IVIM), as well as morphological imaging acquisitions. A repeated nested cross-validation strategy using random forest classifiers was used for model training and evaluation, considering 8 combinations of explanatory features. Shapley additive explanations (SHAP) values were used to assess feature contributions, and the model performance was evaluated using multiple metrics.
RESULTS:One hundred thirteen patients were analyzed (57/113 [50.4%] were women; average age was 51.5 ± 17.5 years, range: 19-88 years). Among the 8 combinations of explanatory features assessed, the performance on predicting malignancy when using the most comprehensive model, which is the most exhaustive one incorporating all 46 explanatory features-including morphology, DWI, DCE, and IVIM, achieved an area under the curve of 0.9 [0.73-0.99]. When using the streamlined "10-feature signature" model, performance reached an area under the curve of 0.88 [0.71-0.99]. Random forest feature importance graphs measured by the mean of SHAP values pinpointed the 10 most impactful features, which comprised 3 quantitative IVIM features, 4 quantitative DCE features, 1 quantitative DWI feature, 1 qualitative DWI feature, and age.
CONCLUSIONS:Our findings demonstrate that a machine learning approach, integrating multiparametric MRI data such as DCE, DWI, IVIM, and morphological imaging, offers high-performing models for differentiating malignant from benign orbital tumors. The streamlined 10-feature signature, with a performance close to the comprehensive model, may be more suitable for clinical application.
更多查看译文
AI 理解论文
溯源树
样例
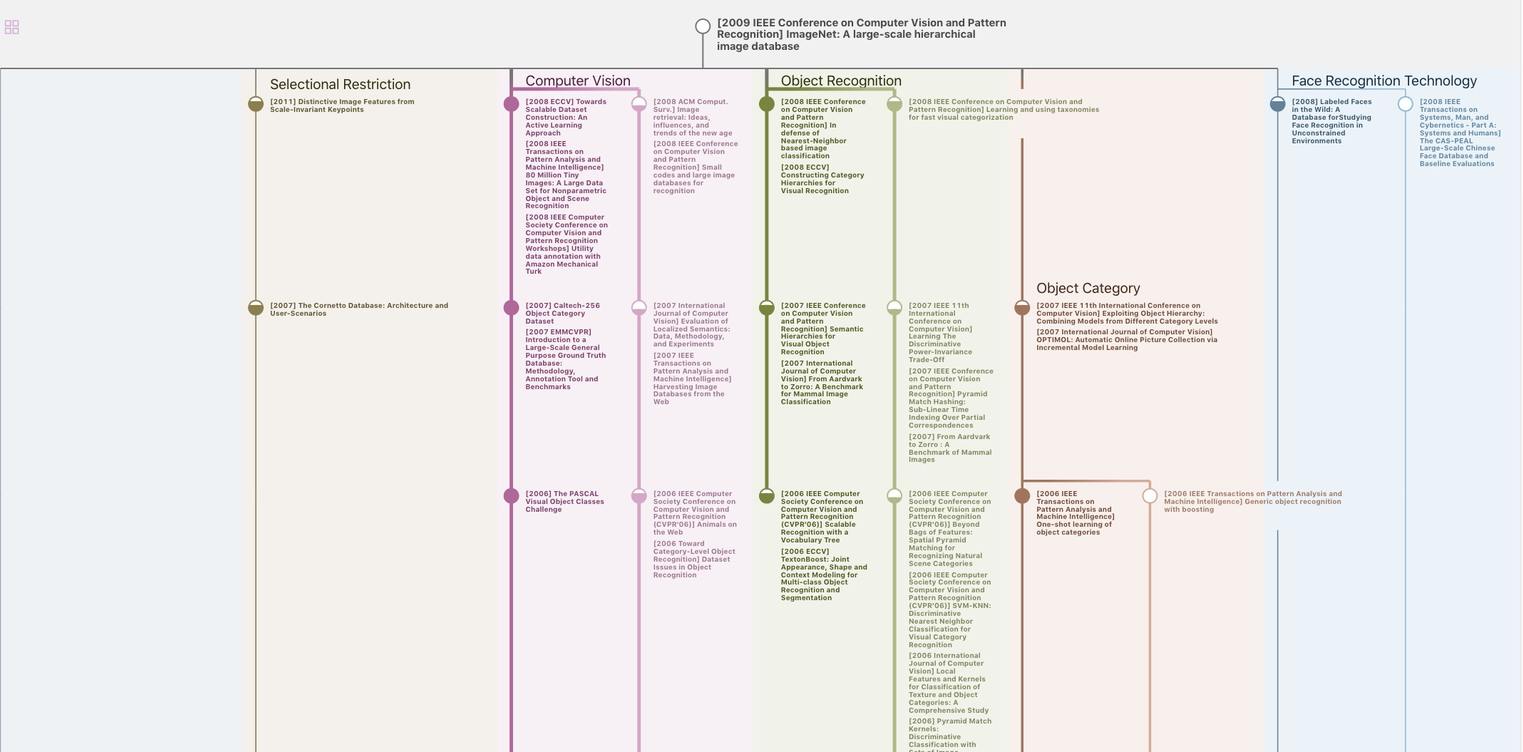
生成溯源树,研究论文发展脉络
Chat Paper
正在生成论文摘要