Improved particle-flow event reconstruction with scalable neural networks for current and future particle detectors
COMMUNICATIONS PHYSICS(2024)
摘要
Efficient and accurate algorithms are necessary to reconstruct particles in the highly granular detectors anticipated at the High-Luminosity Large Hadron Collider and the Future Circular Collider. We study scalable machine learning models for event reconstruction in electron-positron collisions based on a full detector simulation. Particle-flow reconstruction can be formulated as a supervised learning task using tracks and calorimeter clusters. We compare a graph neural network and kernel-based transformer and demonstrate that we can avoid quadratic operations while achieving realistic reconstruction. We show that hyperparameter tuning significantly improves the performance of the models. The best graph neural network model shows improvement in the jet transverse momentum resolution by up to 50% compared to the rule-based algorithm. The resulting model is portable across Nvidia, AMD and Habana hardware. Accurate and fast machine-learning based reconstruction can significantly improve future measurements at colliders. The next generation of high energy particle colliders will have features that allows for highly granular detectors and current methods for particle collision reconstruction are limited. The authors explore machine learning algorithms for reconstructing events in electron-positron collisions for such future colliders obtaining a best-performing graph neural network that enhances the jet transverse momentum resolution by up to 50%, outperforming traditional methods and promising significant advancements in future collider measurements.
更多查看译文
AI 理解论文
溯源树
样例
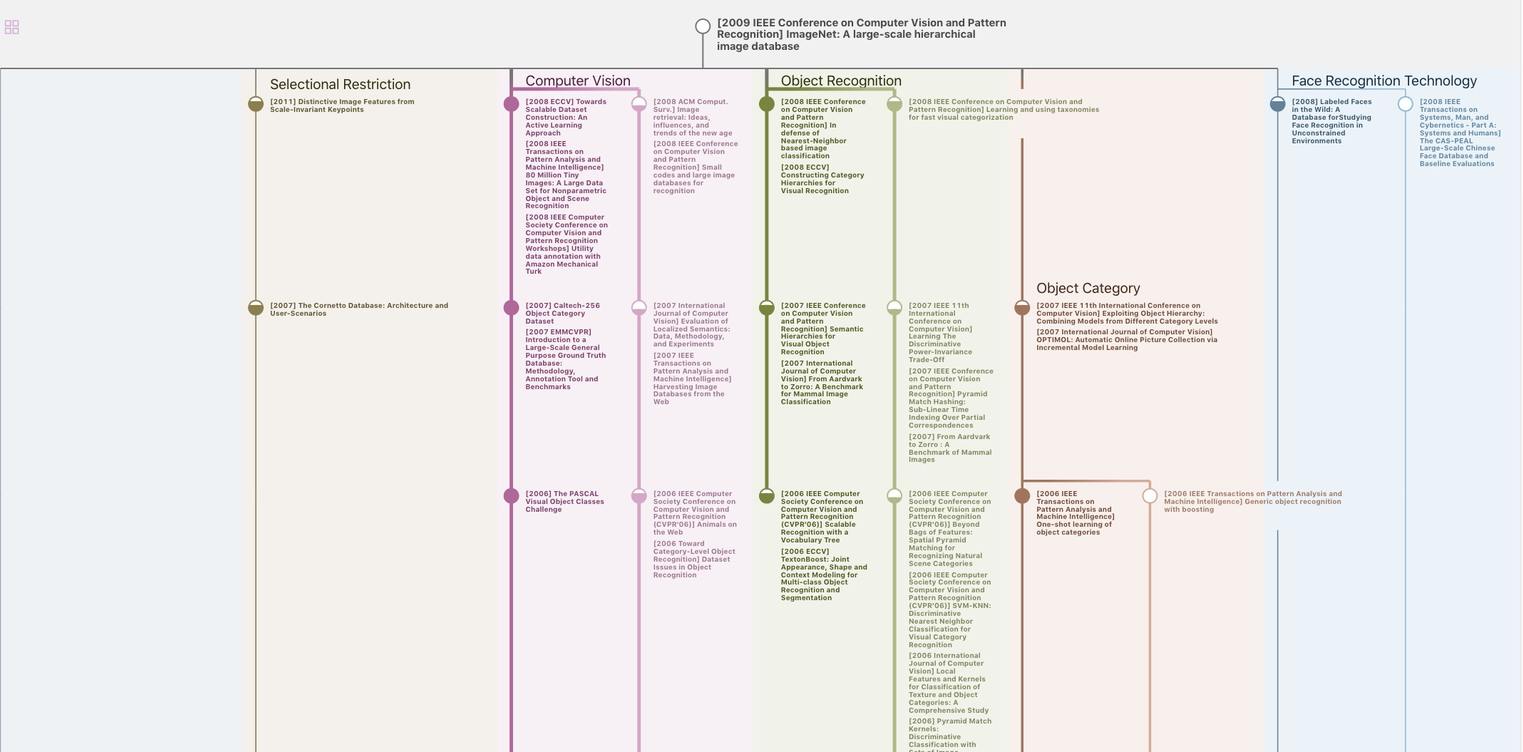
生成溯源树,研究论文发展脉络
Chat Paper
正在生成论文摘要