A short-term load forecasting method for integrated community energy system based on STGCN
Electric Power Systems Research(2024)
Abstract
Accurate integrated energy load forecasting is a crucial prerequisite for energy scheduling and strategy formulation in integrated community energy systems. However, the complex interrelationships among multiple loads within the integrated community energy system often hinder the improvement of load forecasting accuracy. To address the issue of high volatility in forecasting caused by the deep coupling of load relationships, a short-term load forecasting method for integrated community energy systems based on Spatio-Temporal Graph Convolutional Neural Network (STGCN) is proposed. Firstly, an integrated energy node clustering method is proposed, considering load fluctuation characteristics to address the error superposition problem caused by an excessive number of load nodes. Similar load nodes are gathered to reduce random errors in cooling and heating loads. Secondly, we design a dynamic adjacency matrix construction method based on load bias correlation to address situations where multiple load correlations influence each other. Bias correlation is utilized for the dynamic update of the matrix, ensuring accurate load correlations. Furthermore, we construct an STGCN-based integrated energy load forecasting model to mitigate short-term load forecasting fluctuations and identify different periodic patterns for distinct loads. The model incorporates multi-scale convolution kernels to capture integrated energy local features, enhancing feature representation and improving load forecasting accuracy. The proposed method is tested and verified using a real integrated community energy system dataset, showing higher prediction accuracy. Specifically, when compared to the current mainstream MTL-LSTM model, the proposed method predicts an 18.1 % increase in the MAPE index.
MoreTranslated text
Key words
Integrated community energy system,Load characteristics,Correlation network,STGCN
AI Read Science
Must-Reading Tree
Example
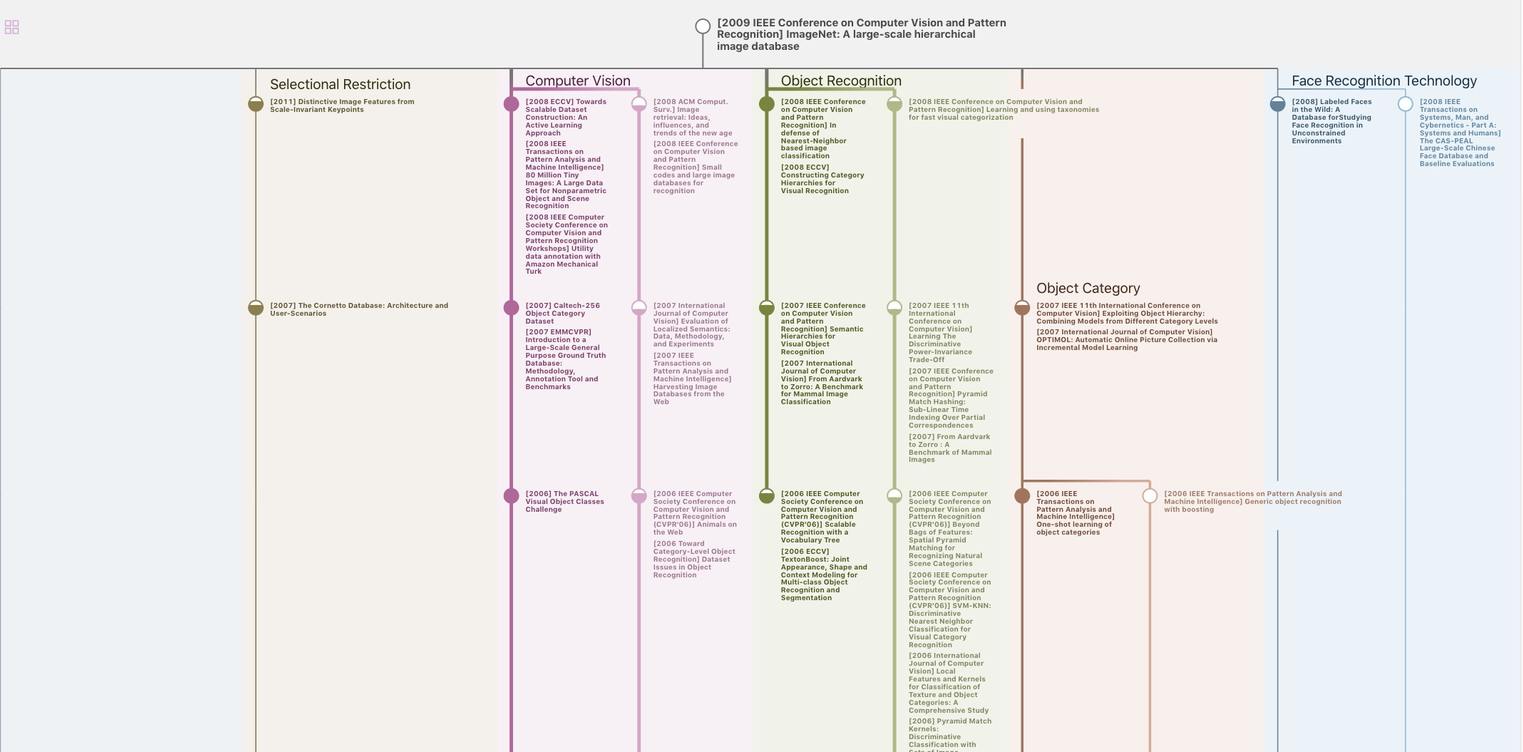
Generate MRT to find the research sequence of this paper
Chat Paper
Summary is being generated by the instructions you defined