An Evaluation of Machine Learning Techniques for Retrieval of Chlorophyll in Open and Coastal Waters
2023 IEEE India Geoscience and Remote Sensing Symposium (InGARSS)(2023)
摘要
Machine Learning (ML) offers a modern approach for estimating water quality parameters from aquatic remote sensing. We compared three ML algorithms: Support Vector Regression (SVR), Random Forest Regression (RFR), and Gradient Boost Regression (GBR), assessing their suitability for estimating chlorophyll (Chl) using remote sensing reflectance (Rrs) in coastal and open waters. We trained and validated the models using a global in-situ dataset from the NOMAD database, encompassing Chl and Rrs values. Chl predictions from all models closely aligned with the in-situ dataset ranges. Comparing the ML models, GBR exhibited better Chl predictions with lower root mean square error and high correlation values. We applied the GBR model to Sentinel-2A/B images to analyse coastal waters in Saudi Arabia. Using five years of Sentinel-2A/B images (2017-2021), we observed Chl changes driven by seasonal variations. This study highlights the adaptability of ML algorithms for aquatic remote sensing.
更多查看译文
关键词
Aquatic Remote Sensing,Chlorophyll,Remote Sensing Reflectance,ML,GBR
AI 理解论文
溯源树
样例
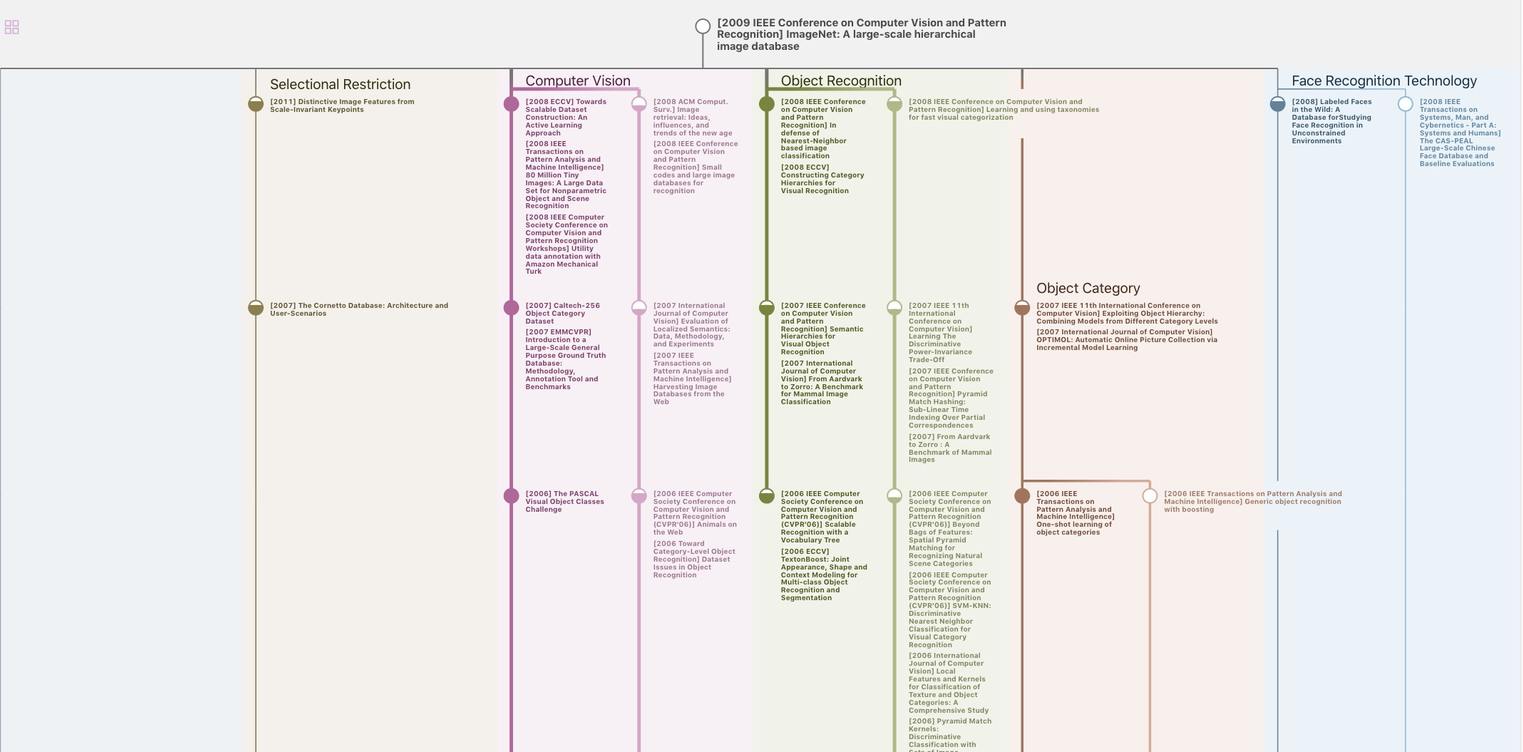
生成溯源树,研究论文发展脉络
Chat Paper
正在生成论文摘要